A sequential learning model with GNN for EEG-EMG-based stroke rehabilitation BCI
Frontiers in Neuroscience(2023)
摘要
IntroductionBrain-computer interfaces (BCIs) have the potential in providing neurofeedback for stroke patients to improve motor rehabilitation. However, current BCIs often only detect general motor intentions and lack the precise information needed for complex movement execution, mainly due to insufficient movement execution features in EEG signals.MethodsThis paper presents a sequential learning model incorporating a Graph Isomorphic Network (GIN) that processes a sequence of graph-structured data derived from EEG and EMG signals. Movement data are divided into sub-actions and predicted separately by the model, generating a sequential motor encoding that reflects the sequential features of the movements. Through time-based ensemble learning, the proposed method achieves more accurate prediction results and execution quality scores for each movement.ResultsA classification accuracy of 88.89% is achieved on an EEG-EMG synchronized dataset for push and pull movements, significantly outperforming the benchmark method's performance of 73.23%.DiscussionThis approach can be used to develop a hybrid EEG-EMG brain-computer interface to provide patients with more accurate neural feedback to aid their recovery.
更多查看译文
关键词
brain-computer interfaces,neurofeedback,graph neural network,stroke rehabilitation,corticomuscular coherence,sequential learning
AI 理解论文
溯源树
样例
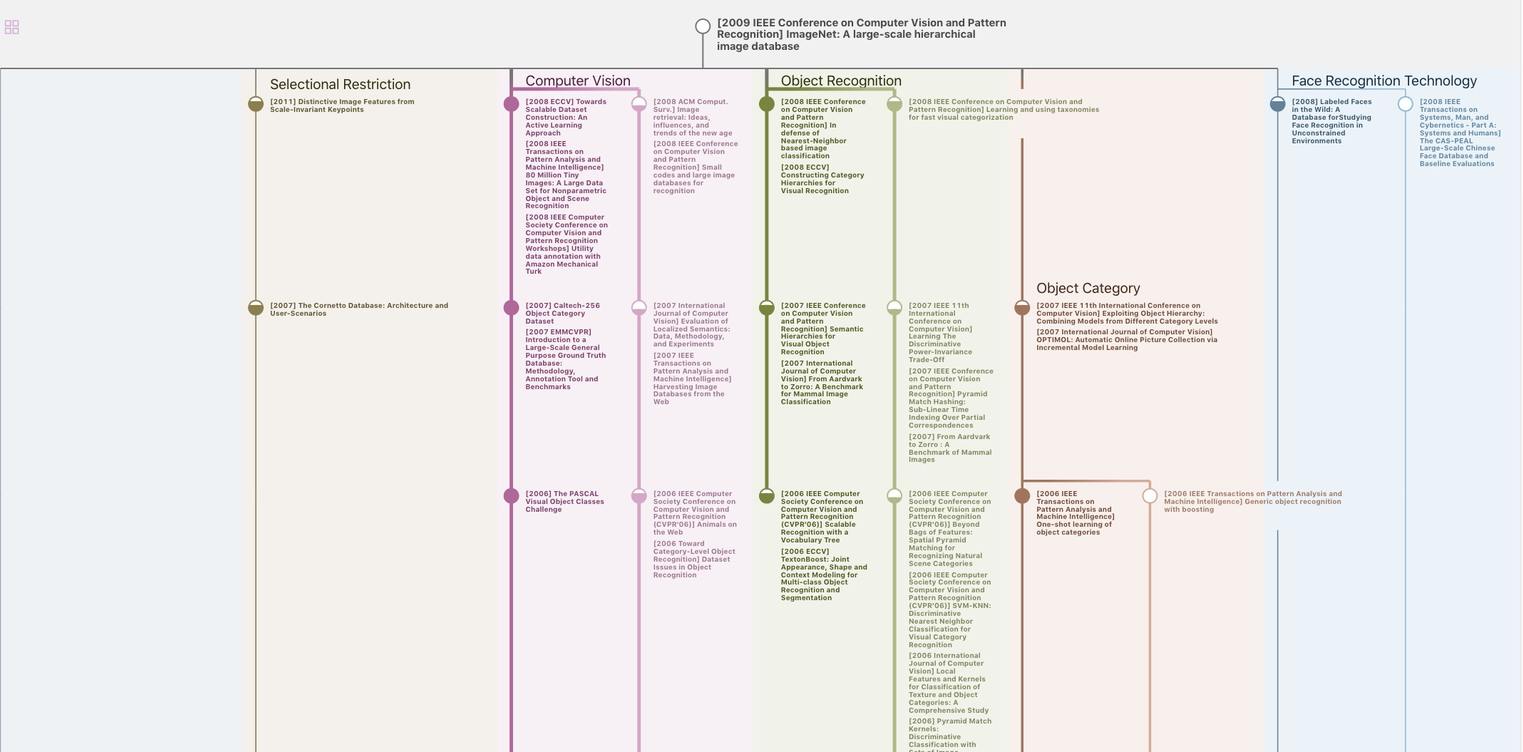
生成溯源树,研究论文发展脉络
Chat Paper
正在生成论文摘要