Measuring Re-identification Risk.
CoRR(2023)
摘要
Compact user representations (such as embeddings) form the backbone of personalization services. In this work, we present a new theoretical framework to measure re-identification risk in such user representations. Our framework, based on hypothesis testing, formally bounds the probability that an attacker may be able to obtain the identity of a user from their representation. As an application, we show how our framework is general enough to model important real-world applications such as the Chrome's Topics API for interest-based advertising. We complement our theoretical bounds by showing provably good attack algorithms for re-identification that we use to estimate the re-identification risk in the Topics API. We believe this work provides a rigorous and interpretable notion of re-identification risk and a framework to measure it that can be used to inform real-world applications.
更多查看译文
关键词
risk,re-identification
AI 理解论文
溯源树
样例
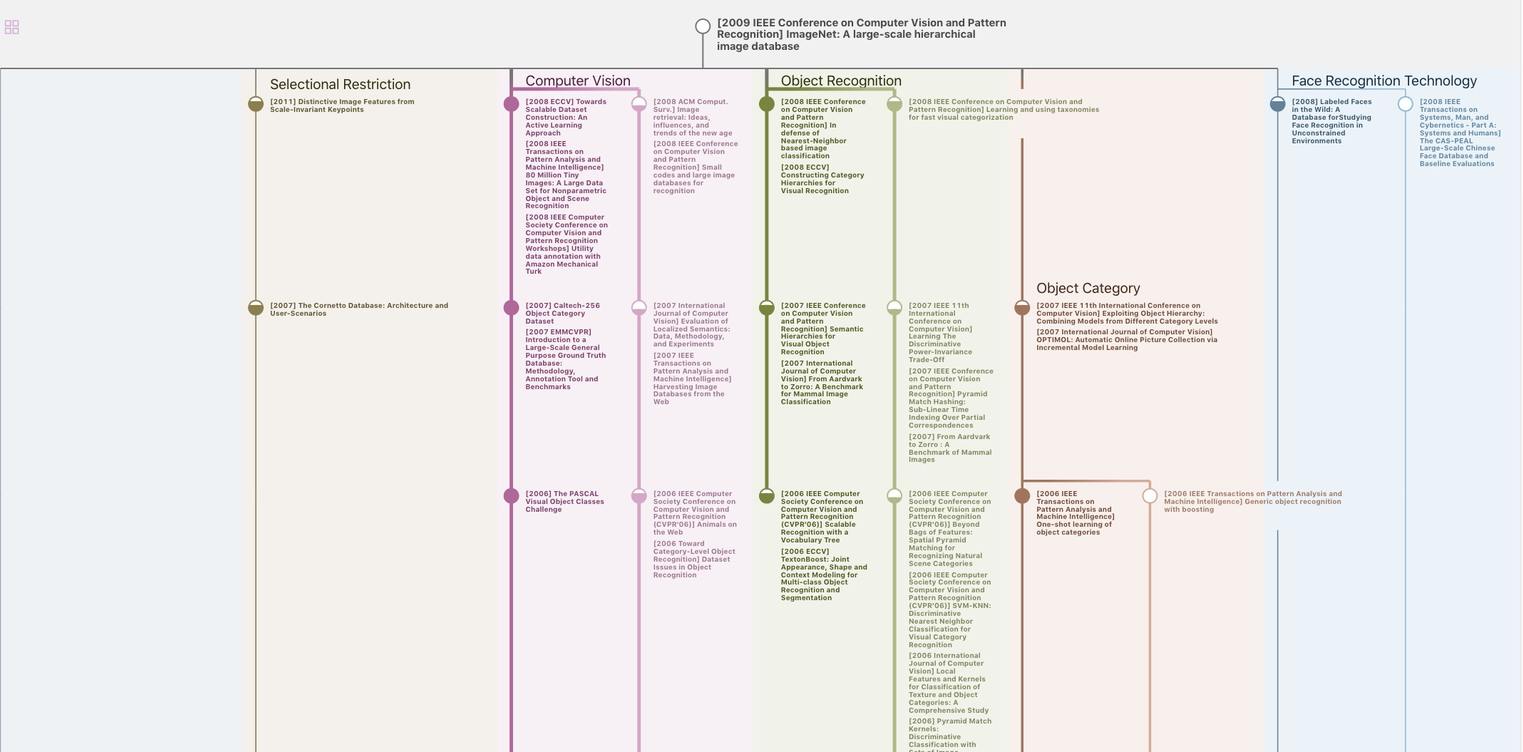
生成溯源树,研究论文发展脉络
Chat Paper
正在生成论文摘要