Combining Stochastic Explainers and Subgraph Neural Networks can Increase Expressivity and Interpretability
CoRR(2023)
摘要
Subgraph-enhanced graph neural networks (SGNN) can increase the expressive power of the standard message-passing framework. This model family represents each graph as a collection of subgraphs, generally extracted by random sampling or with hand-crafted heuristics. Our key observation is that by selecting "meaningful" subgraphs, besides improving the expressivity of a GNN, it is also possible to obtain interpretable results. For this purpose, we introduce a novel framework that jointly predicts the class of the graph and a set of explanatory sparse subgraphs, which can be analyzed to understand the decision process of the classifier. We compare the performance of our framework against standard subgraph extraction policies, like random node/edge deletion strategies. The subgraphs produced by our framework allow to achieve comparable performance in terms of accuracy, with the additional benefit of providing explanations.
更多查看译文
关键词
stochastic explainers,subgraph neural networks
AI 理解论文
溯源树
样例
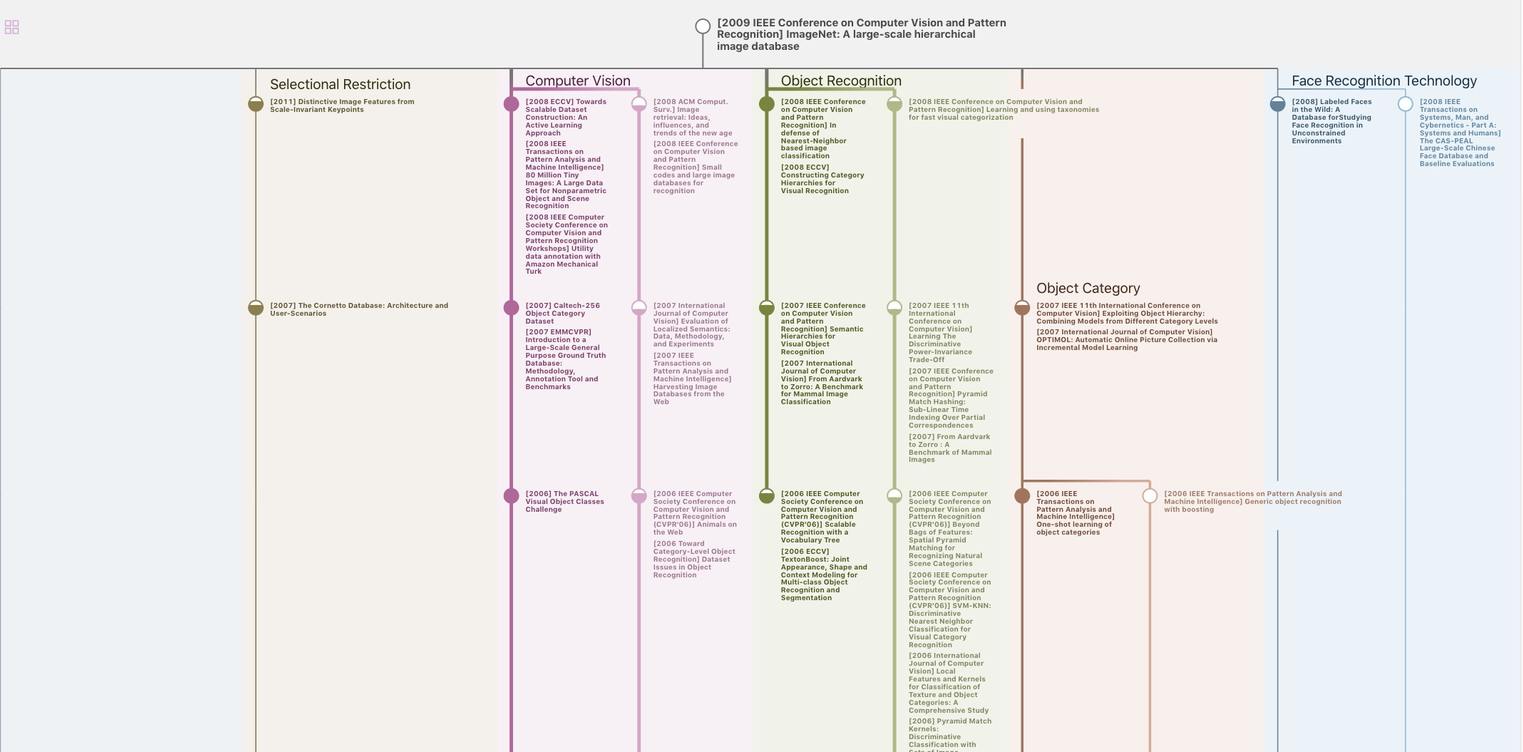
生成溯源树,研究论文发展脉络
Chat Paper
正在生成论文摘要