Convex Dual Theory Analysis of Two-Layer Convolutional Neural Networks With Soft-Thresholding
IEEE TRANSACTIONS ON NEURAL NETWORKS AND LEARNING SYSTEMS(2024)
摘要
Soft-thresholding has been widely used in neural networks. Its basic network structure is a two-layer convolution neural network with soft-thresholding. Due to the network's nature of nonlinear and nonconvex, the training process heavily depends on an appropriate initialization of network parameters, resulting in the difficulty of obtaining a globally optimal solution. To address this issue, a convex dual network is designed here. We theoretically analyze the network convexity and prove that the strong duality holds. Extensive results on both simulation and real-world datasets show that strong duality holds, the dual network does not depend on initialization and optimizer, and enables faster convergence than the state-of-the-art two-layer network. This work provides a new way to convexify soft-thresholding neural networks. Furthermore, the convex dual network model of a deep soft-thresholding network with a parallel structure is deduced.
更多查看译文
关键词
Convex optimization,nonconvexity,soft-thresholding,strong duality
AI 理解论文
溯源树
样例
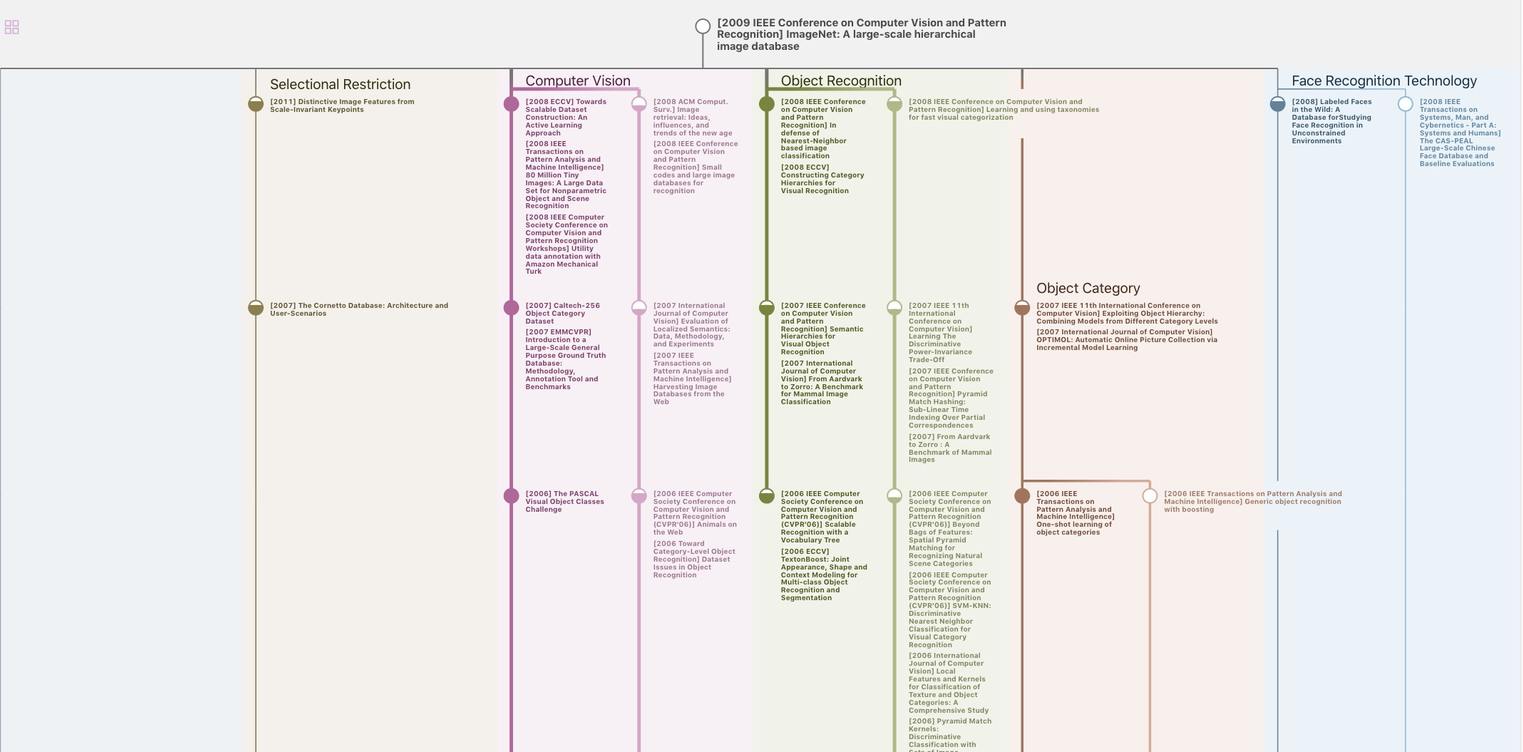
生成溯源树,研究论文发展脉络
Chat Paper
正在生成论文摘要