Radio Frequency Energy Harvesting-Based Self-Powered Dairy Cow Behavior Classification System
IEEE Sensors Journal(2023)
摘要
Dairy cow behavior is closely related to the cow’s health and physiological status. Automatic diagnosis and precision dairy farming are achieved by intelligent behavior recognition. We propose a self-powered system to classify various dairy cow behaviors based on acceleration data to obtain a novel and effective solution for dairy cow behavior monitoring. A neck-mounted sensor tag integrated with an accelerometer is used to record dairy cow behavior. The sensor tag operates without any batteries. Energy is harvested from radio frequency (RF) waves at the ultrahigh-frequency band of 915 MHz emitted by a reader. The device can initiate receiving power for conversion at input powers as low as −8 dBm. The maximum achievable power conversion efficiency (PCE) is 54.5%. The sensor tag consumes only
$53 \mu \text{A}$
of low average current with a sampling frequency of 10 Hz to acquire acceleration data and backscatter them to the reader. Dairy cow behaviors were observed and recorded for 14 days, with three behaviors selected for the following classification: standing, walking, and grazing. Two deep learning models are developed to classify these behaviors, including a 1-D convolutional neural network (1D-CNN) and long short-term memory (LSTM). The collected data were divided into 12-s windows, which were used as input to classifiers. The walking behavior is most accurately recognized in both models (95.56% with 1D-CNN and 92.15% with LSTM), and the best classification accuracy of 94.35% is achieved from the 1D-CNN model.
更多查看译文
关键词
Accelerometer,collar-mounted device,convolutional neural network (CNN),deep learning,long short-term memory (LSTM),radio frequency energy harvesting (RFEH),smart dairy farming
AI 理解论文
溯源树
样例
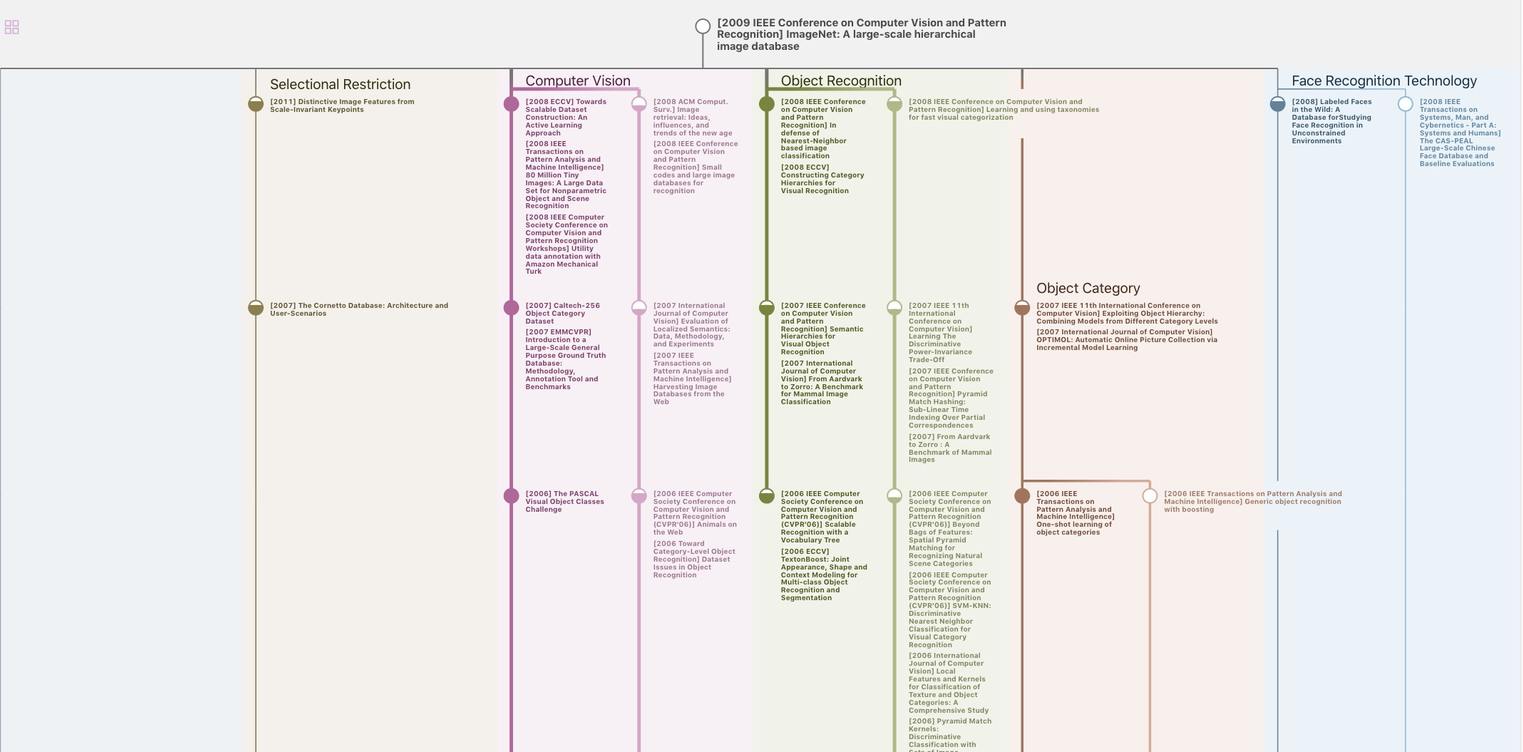
生成溯源树,研究论文发展脉络
Chat Paper
正在生成论文摘要