Hybrid Post-Training Quantization for Super-Resolution Neural Network Compression
IEEE Signal Processing Letters(2023)
摘要
Quantization is a widely adopted technique to reduce the storage cost of neural networks. However, existing methods primarily focus on minimizing the quantization error of neural network parameters without considering the correlation between the quantization error and performance of quantized neural networks. Motivated by this consideration, we propose a hybrid post-training quantization (HPTQ) method for super-resolution neural networks. Layer-wise quantization and piecewise quantization are integrated based on error sensitivity and the quantization error of parameters. In HPTQ, we utilize Taylor expansion to demonstrate that the performance distortion of quantized neural networks is a weighted average of parameter quantization errors with respect to gradients. To reduce the quantization error, we apply uniform and clustered quantization to parameters in dense and sparse regions, respectively. Furthermore, we allocate larger bit-widths to layers with higher error sensitivity indicated by gradients. Numerical experiments show that the super-resolution neural networks perform better under the proposed quantization approach compared to existing quantization methods.
更多查看译文
关键词
Layer-wise quantization,neural network compression,piecewise quantization,super-resolution neural network
AI 理解论文
溯源树
样例
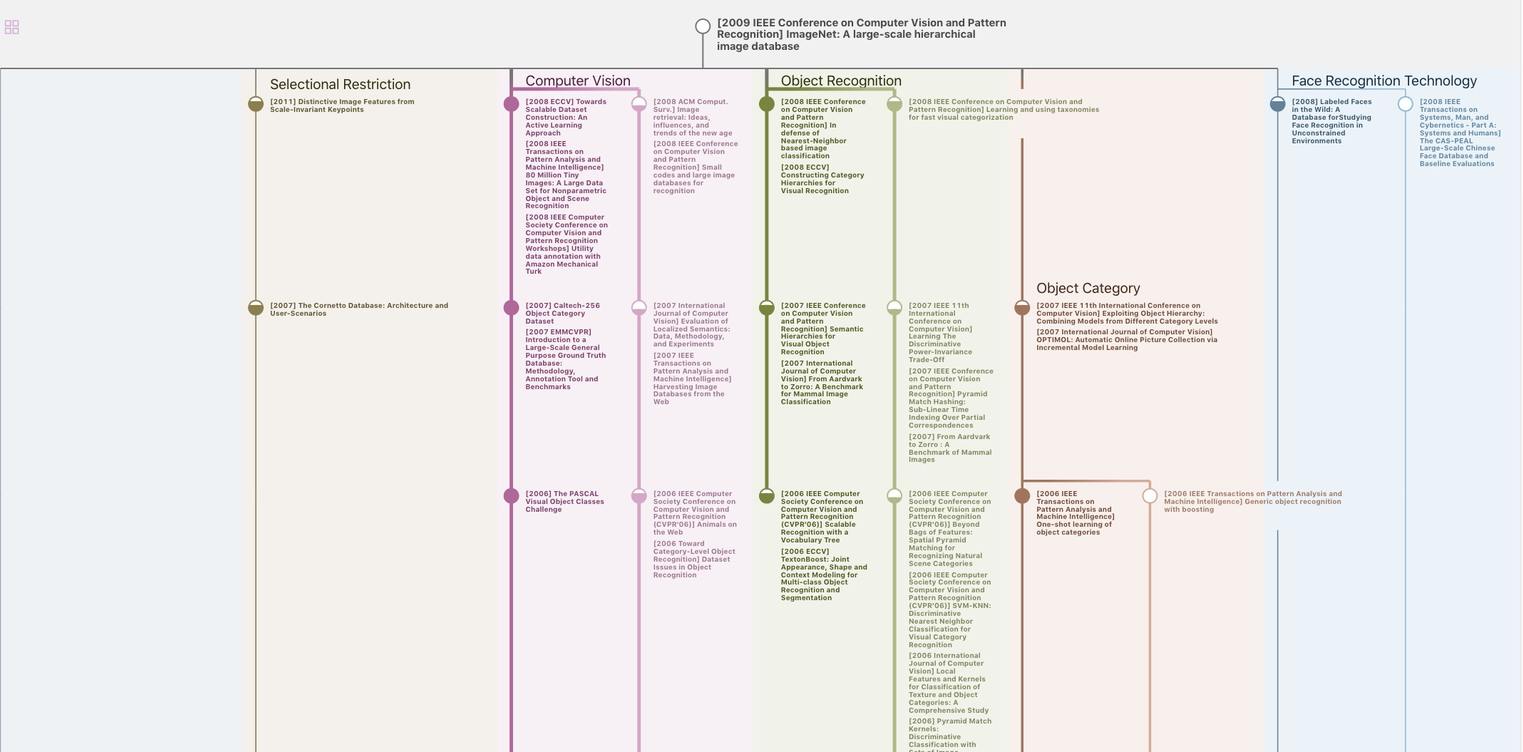
生成溯源树,研究论文发展脉络
Chat Paper
正在生成论文摘要