Deep learning–based diagnosis of osteoblastic bone metastases and bone islands in computed tomograph images: a multicenter diagnostic study
European radiology(2023)
摘要
Objective To develop and validate a deep learning (DL) model based on CT for differentiating bone islands and osteoblastic bone metastases. Materials and methods The patients with sclerosing bone lesions (SBLs) were retrospectively included in three hospitals. The images from site 1 were randomly assigned to the training (70%) and intrinsic verification (10%) datasets for developing the two-dimensional (2D) DL model (single-slice input) and “2.5-dimensional” (2.5D) DL model (three-slice input) and to the internal validation dataset (20%) for evaluating the performance of both models. The diagnostic performance was evaluated using the internal validation set from site 1 and additional external validation datasets from site 2 and site 3 . And statistically analyze the performance of 2D and 2.5D DL models. Results In total, 1918 SBLs in 728 patients in site 1 , 122 SBLs in 71 patients in site 2 , and 71 SBLs in 47 patients in site 3 were used to develop and test the 2D and 2.5D DL models. The best performance was obtained using the 2.5D DL model, which achieved an AUC of 0.996 (95% confidence interval [CI], 0.995–0.996), 0.958 (95% CI, 0.958–0.960), and 0.952 (95% CI, 0.951–0.953) and accuracies of 0.950, 0.902, and 0.863 for the internal validation set, the external validation set from site 2 and site 3 , respectively. Conclusion A DL model based on a three-slice CT image input (2.5D DL model) can improve the prediction of osteoblastic bone metastases, which can facilitate clinical decision-making. Key Points • This study investigated the value of deep learning models in identifying bone islands and osteoblastic bone metastases. • Three-slice CT image input (2.5D DL model) outweighed the 2D model in the classification of sclerosing bone lesions. • The 2.5D deep learning model showed excellent performance using the internal (AUC, 0.996) and two external (AUC, 0.958; AUC, 0.952) validation sets.
更多查看译文
关键词
Bone neoplasms,Computed tomography,Deep learning,Osteogenesis,X-ray
AI 理解论文
溯源树
样例
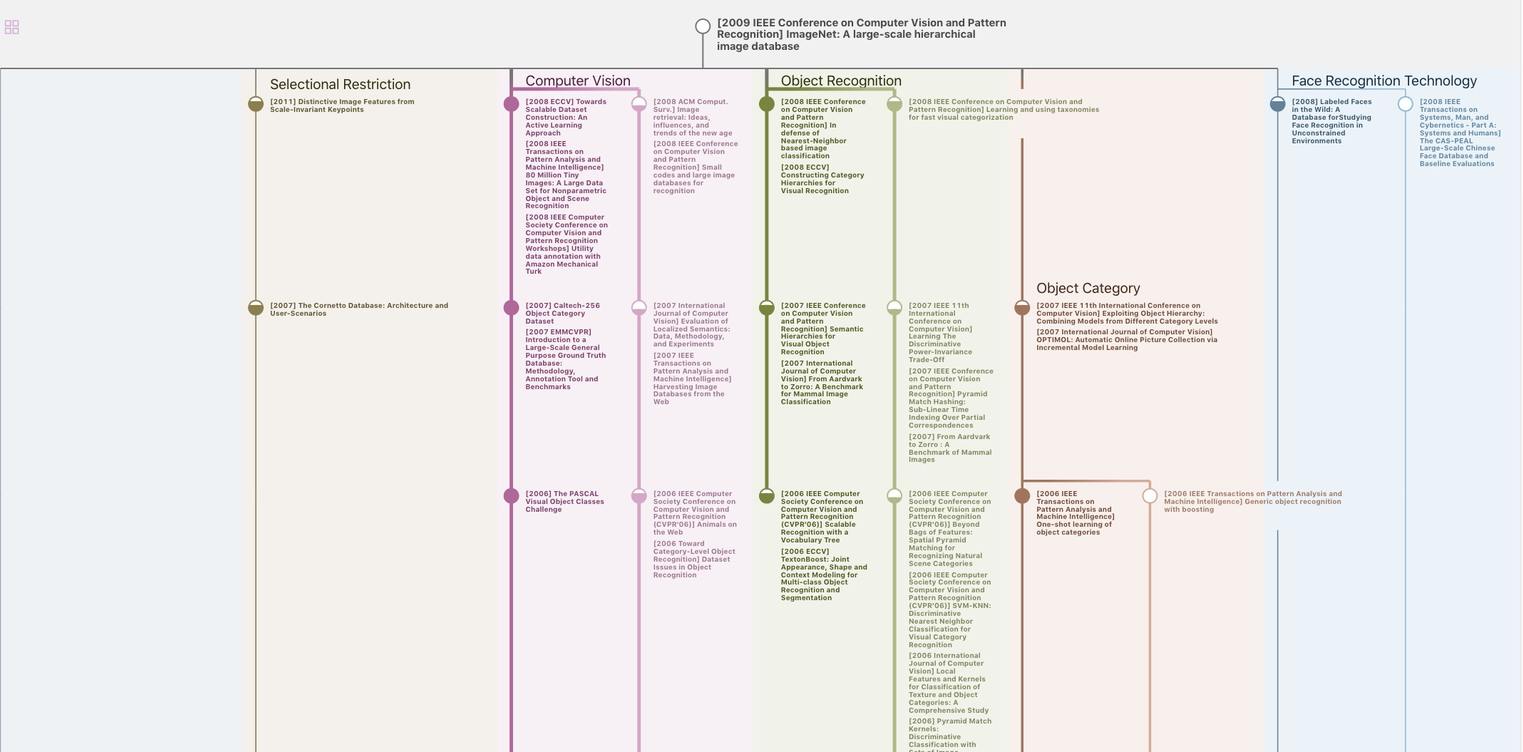
生成溯源树,研究论文发展脉络
Chat Paper
正在生成论文摘要