Dynamic event-triggered control for discrete-time nonlinear Markov jump systems using policy iteration-based adaptive dynamic programming
Nonlinear Analysis: Hybrid Systems(2023)
摘要
This paper investigates a dynamic event-triggered optimal control problem of discrete-time (DT) nonlinear Markov jump systems (MJSs) via exploring policy iteration (PI) adaptive dynamic programming (ADP) algorithms. The performance index function (PIF) defined in each subsystem is updated by utilizing an online PI algorithm, and the corresponding control policy is derived via solving the optimal PIF. Then, we adopt neural network (NN) techniques, including an actor network and a critic network, to estimate the iterative PIF and control policy. Moreover, the designed dynamic event-triggered mechanism (DETM) is employed to avoid wasting additional resources when the estimated iterative control policy is updated. Finally, based on the Lyapunov difference method, it is proved that the system stability and the convergence of all signals can be guaranteed under the developed control scheme. A simulation example for DT nonlinear MJSs with two system modes is presented to demonstrate the feasibility of the control design scheme.
更多查看译文
关键词
Markov jump systems,Neural network,Dynamic event-triggered mechanism,Policy iteration,Adaptive dynamic programming
AI 理解论文
溯源树
样例
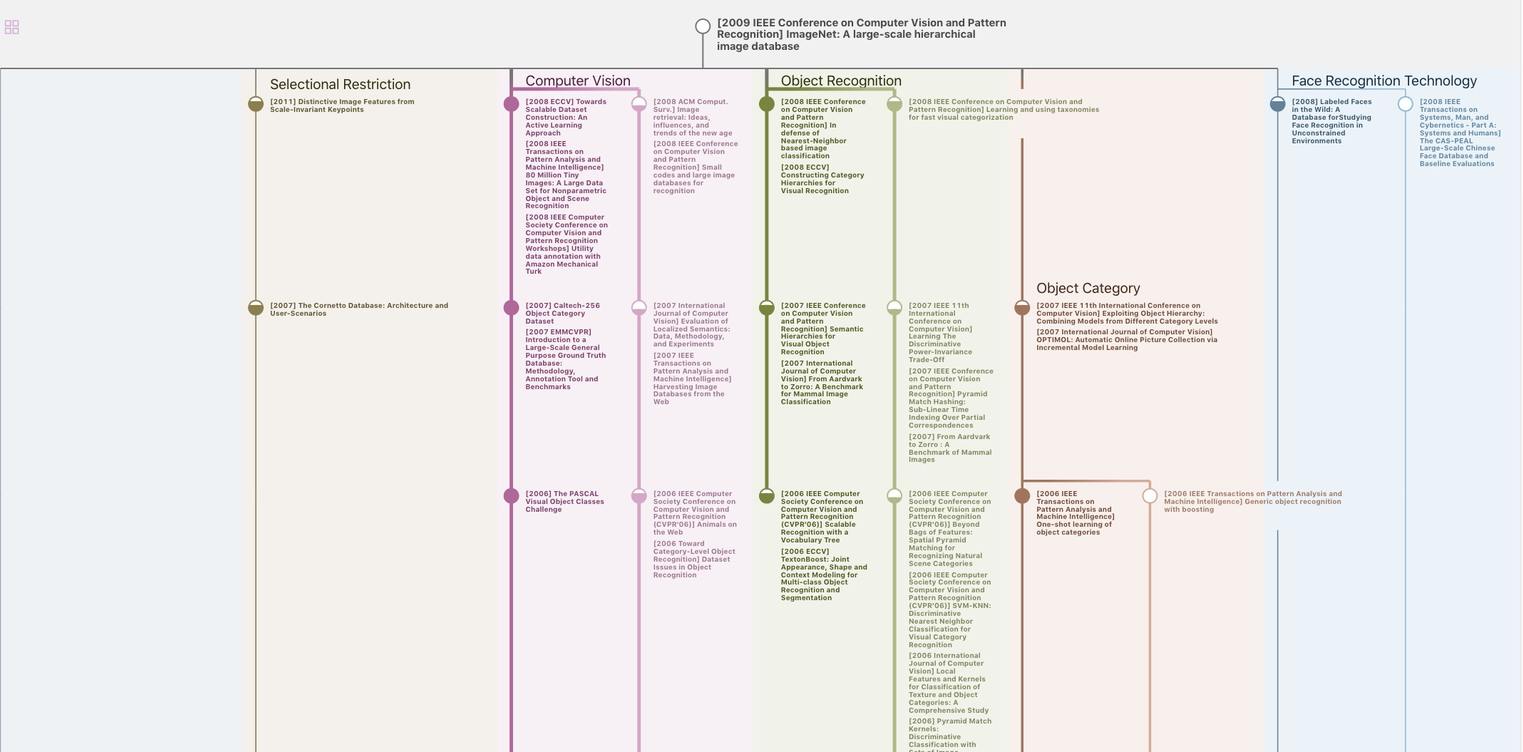
生成溯源树,研究论文发展脉络
Chat Paper
正在生成论文摘要