Interpreting learning models in manufacturing processes: Towards explainable AI methods to improve trust in classifier predictions
Journal of Industrial Information Integration(2023)
摘要
Smart manufacturing processes, building upon machine learning (ML) models could potentially reduce the pre-production testing and validation time for new processes. Beyond calculating accurate and reliable models, one critical challenge would be for users of these models (plant operators, engineers and technicians) to trust these models’ outputs. We propose to apply explainable AI methods to create trustworthy AI-based manufacturing systems. Consequently, these systems will be enriched with capabilities to explain their reasoning processes and outputs (e.g., predictions) automatically. This paper applies explainable AI methods to two problems in manufacturing: ultrasonic weld (USW) quality prediction and body-in-white (BIW) dimensional variability reduction. Class activation maps were computed to explain the effect of input signals and their patterns on the quality predictions of an ultrasonic weld yield by a neural network (good or bad). Contrastive gradient based saliency maps were also created to assess the robustness of this classifier. Furthermore, we explain a given connectionist network that predicts the dimensional quality of body-in-white framer points based on deviations in underbody points. Explaining these predictions help engineers understand which underbody points have more influence on deviations in the framer points. These two applications highlight the importance of explainable AI methods in the modern manufacturing industry.
更多查看译文
关键词
Explainable AI,Classifier learning systems,Ultrasonic weld process monitoring,Artificial intelligence quotient
AI 理解论文
溯源树
样例
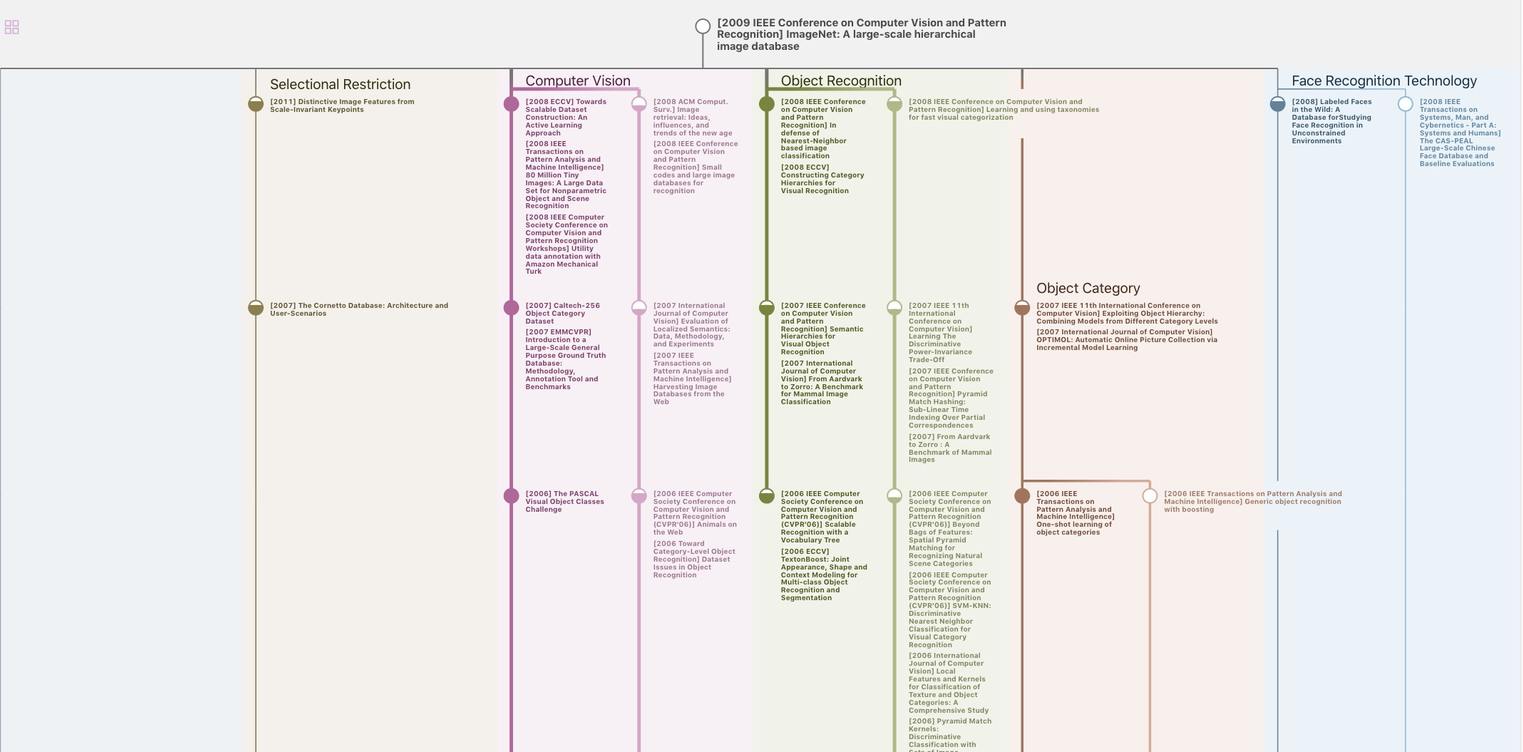
生成溯源树,研究论文发展脉络
Chat Paper
正在生成论文摘要