A milling tool wear monitoring method with sensing generalization capability
Journal of Manufacturing Systems(2023)
摘要
Tool wear monitoring is considered a core technology for next-generation computer numerical control machine tools. However, differences in the characteristics of acquired signals prevent the monitoring model from generalizing between sensing channels, which greatly limits the application in actual industrial environment. To overcome the shortcomings of existing methods, a tool condition monitoring method with sensing generalization capability is proposed. Firstly, the adaptive superposition function of multi-feature is established to automatically adjust the weight of each feature so that the domain features can be adaptively reinforced or penalized. After that, the reinforced time series data are fed into the proposed stacked bidirectional GRU model. The flank wear values are output through a fully connected layer to monitor the tool condition in real-time. Finally, the generalization capability of the proposed method between different types of sensing data is verified through a comparative study of the performance of local features, reinforced features and ARIMA model. The proposed feature reinforcement algorithm reduces the variation in recognition accuracy of different sensing signals by 76.28%, which ensures sensing generalization capability. The adaptive reinforcement and penalization of time series data resulted in a 59.41% improvement in the performance of local features. The average accuracy of the proposed model is 65.61% better than that of the ARIMA model. Thanks to the feature reinforcement algorithm and extracted fractal features, the proposed method is not only applicable to the machining of block-shaped workpieces but also suitable for the milling of thin-walled parts. This study provides a theoretical reference for the standardized application of tool wear monitoring in intelligent manufacturing systems.
更多查看译文
关键词
Tool condition monitoring,Deep learning,Feature reinforcement,Thin-walled parts milling,Stacked bidirectional GRU
AI 理解论文
溯源树
样例
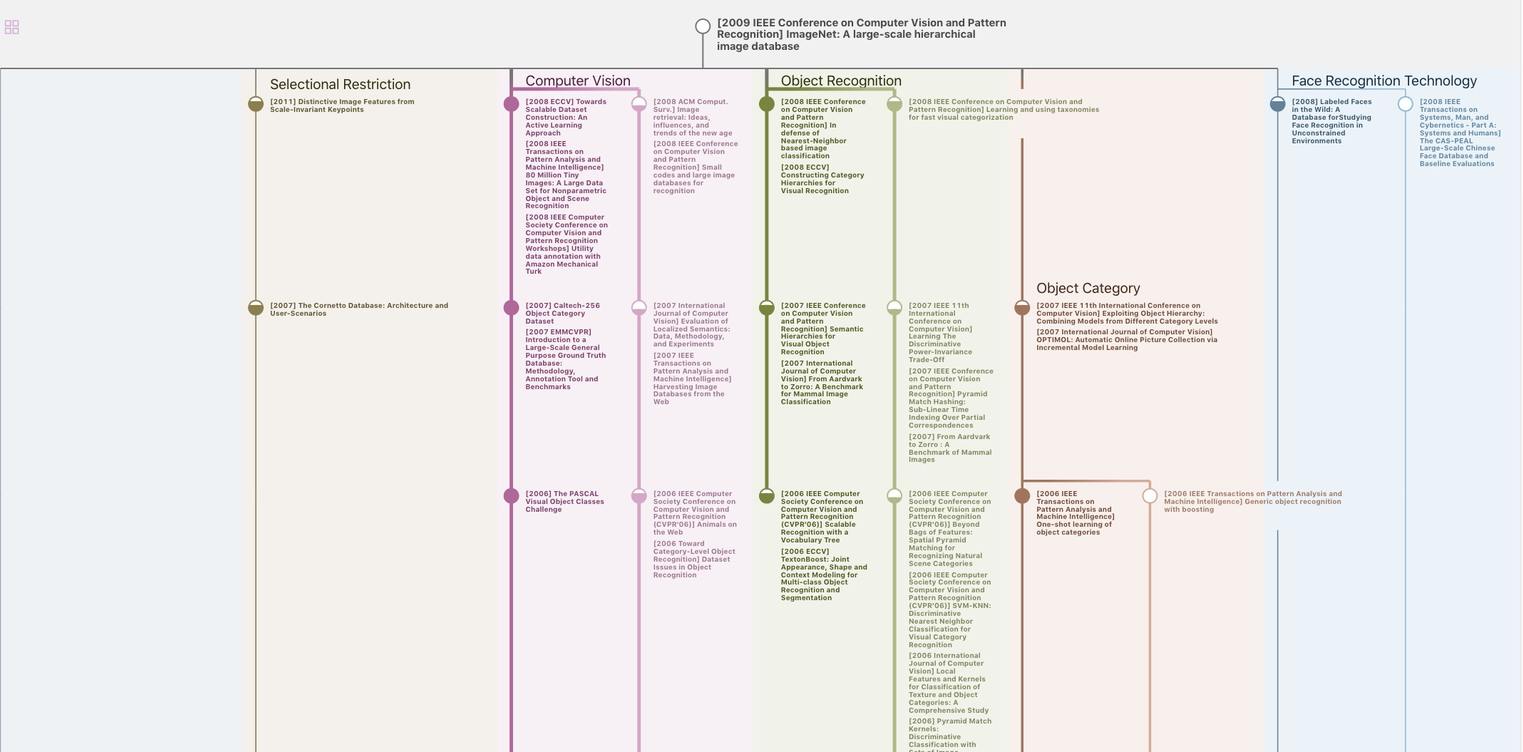
生成溯源树,研究论文发展脉络
Chat Paper
正在生成论文摘要