An attention-based bilinear feature extraction mechanism for fine-grained laser welding molten pool/keyhole defect recognition
Journal of Manufacturing Processes(2023)
摘要
Vision-based molten pool/keyhole (MPK) defect recognition is essential for online monitoring of laser welding quality. However, the visual differences corresponding to different MPK states are extremely small, which especially brings great challenges to the recognition of defect subclasses. In this paper, an attention-based bilinear feature extraction mechanism is developed for recognizing fine-grained laser welding MPK defects. First, first-order linear features of MPK image are extracted through a skeleton network. Second, spatial attention and channel attention are designed to extract first-order functional features from the first-order linear features of the MPK image, and the attention mechanism explicitly models the network's ability to learn discriminative location and attribute features. Third, second-order bilinear features are extracted from first-order functional features of the MPK image by a bilinear confluence operation and used for fine-grained MPK image recognition. Finally, three laser welding MPK image datasets with different fine-grained degrees are constructed, and the proposed method is comprehensively tested for ablation and comparison. The results show that the proposed method has an accuracy of 97.45 % for the recognition of 9 types of laser welding MPK defects.
更多查看译文
关键词
Laser welding,Computer vision,Defect recognition,Deep learning,Fine-grained
AI 理解论文
溯源树
样例
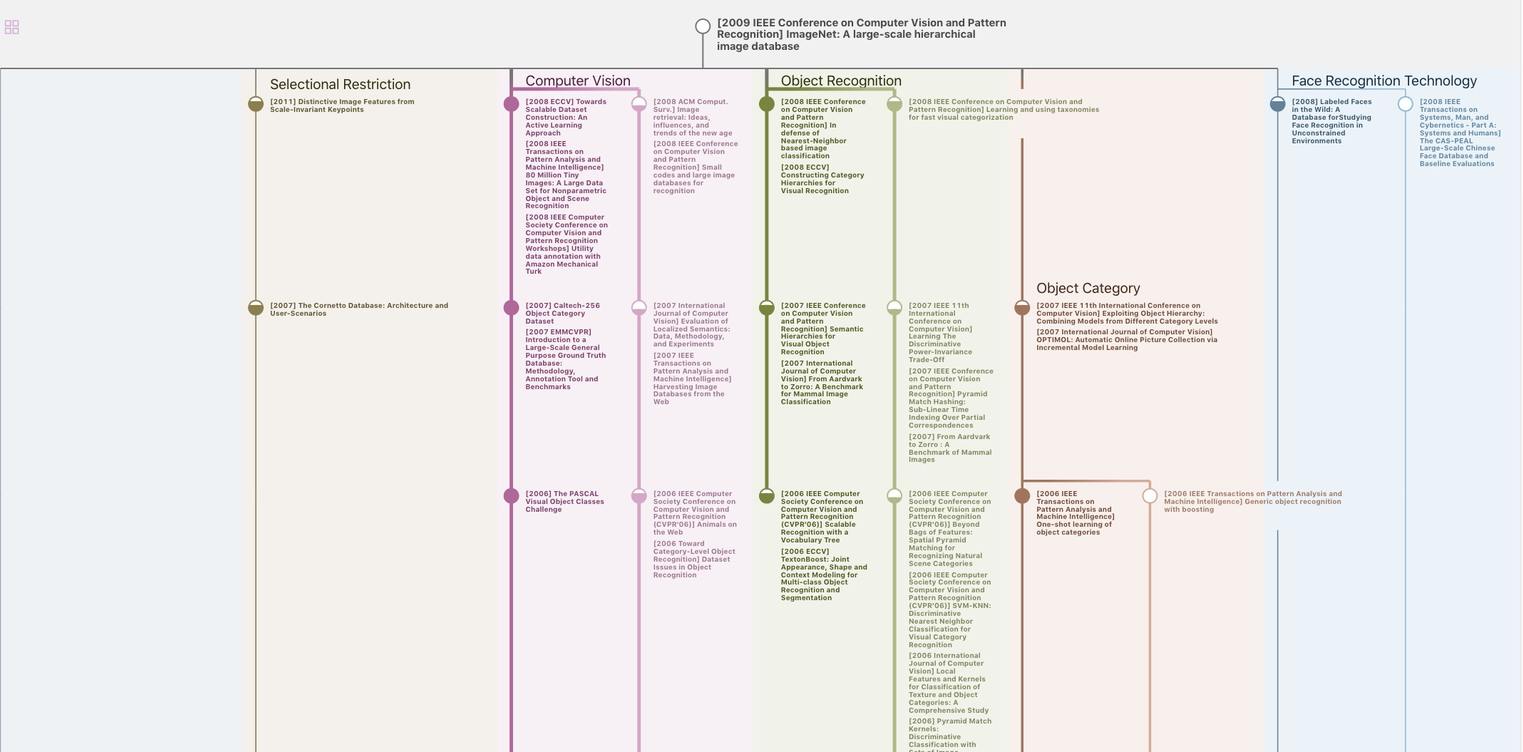
生成溯源树,研究论文发展脉络
Chat Paper
正在生成论文摘要