Prior skeleton based online deep reinforcement learning for coronary artery centerline extraction.
Proceedings of the Institution of Mechanical Engineers. Part H, Journal of engineering in medicine(2023)
摘要
Coronary centerline extraction is an essential technique for X-ray coronary angiography (XCA) image analysis, which provides qualitative and quantitative guidance for percutaneous coronary intervention (PCI). In this paper, an online deep reinforcement learning method for coronary centerline extraction is proposed based on the prior vascular skeleton. Firstly, with XCA image preprocessing (foreground extraction and vessel segmentation) results, the improved image thinning algorithm is used to rapidly extract the preliminary vascular skeleton network. On this basis, according to the spatial-temporal and morphological continuity of the angiography image sequence, the connectivity of different branches is determined using k-means clustering, and the vessel segments are then grouped, screened, and reconnected to obtain the aorta and its major branches. Finally, using the previous results as prior information, an online Deep Q-Network (DQN) reinforcement learning method is proposed to optimize each branch simultaneously. It comprehensively considers grayscale intensity and eigenvector continuity to achieve the combination of data-driven and model-driven without pre-training. Experimental results on clinical images and the third-party dataset demonstrate that the proposed method can accurately extract, restructure, and optimize the centerline of XCA images with a higher overall accuracy than the existing state-of-the-art methods.
更多查看译文
关键词
X-ray coronary angiography,centerline extraction,deep reinforcement learning,online optimization,prior information
AI 理解论文
溯源树
样例
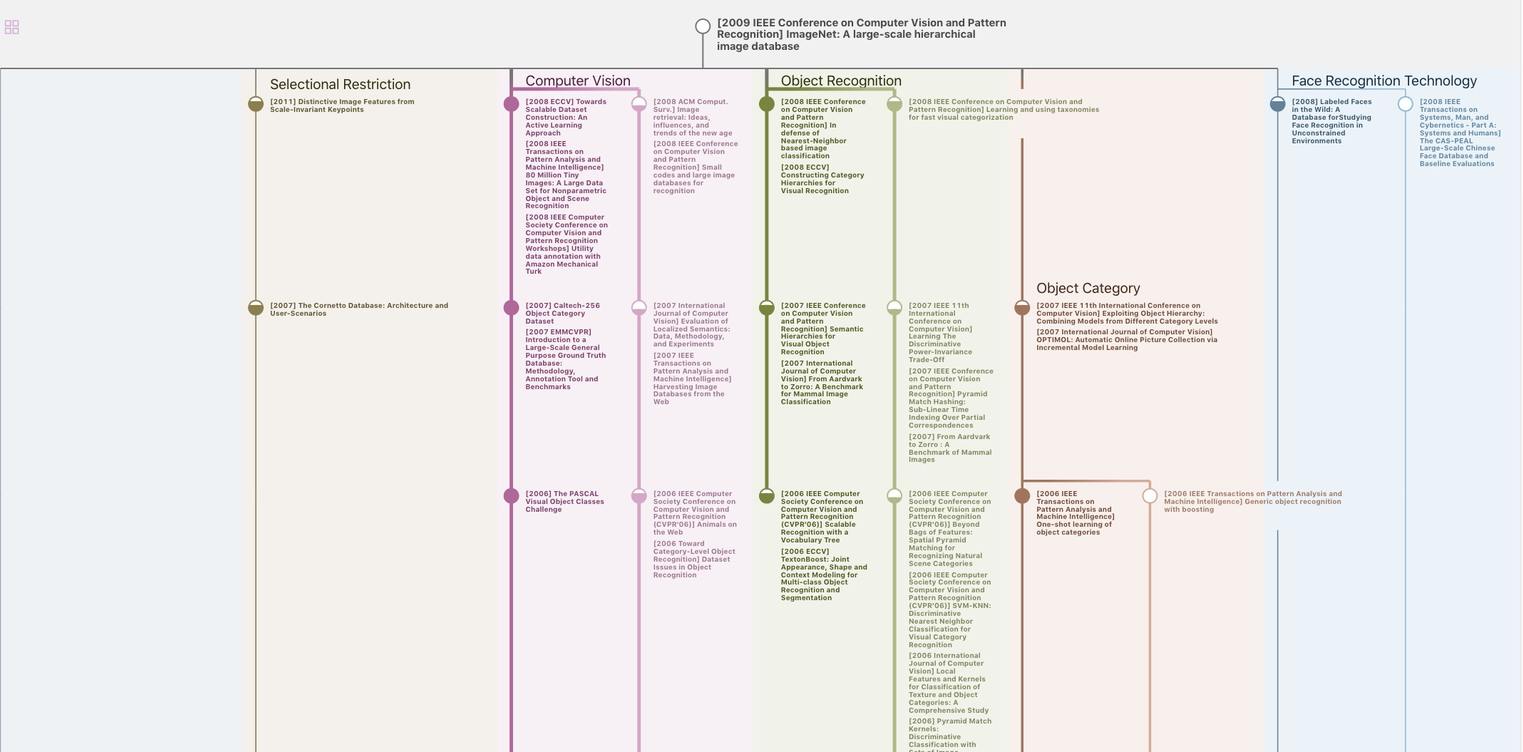
生成溯源树,研究论文发展脉络
Chat Paper
正在生成论文摘要