The potential for different computed tomography-based machine learning networks to automatically segment and differentiate pelvic and sacral osteosarcoma from Ewing's sarcoma
Quantitative Imaging in Medicine and Surgery(2023)
摘要
Background: This study aimed to explore optimal computed tomography (CT)-based machine learning and deep learning methods for the identification of pelvic and sacral osteosarcomas (OS) and Ewing's sarcomas (ES). Methods: A total of 185 patients with pathologically confirmed pelvic and sacral OS and ES were analyzed. We first compared the performance of 9 radiomics-based machine learning models, 1 radiomics- based convolutional neural networks (CNNs) model, and 1 3-dimensional (3D) CNN model, respectively. We then proposed a 2-step no-new-Net (nnU-Net) model for the automatic segmentation and identification of OS and ES. The diagnoses by 3 radiologists were also obtained. The area under the receiver operating characteristic curve (AUC) and accuracy (ACC) were used to evaluate the different models. Results: Age, tumor size, and tumor location showed significant differences between OS and ES (P<0.01). For the radiomics-based machine learning models, logistic regression (LR; AUC =0.716, ACC =0.660) performed best in the validation set. However, the radiomics-based CNN model had an AUC of 0.812 and ACC of 0.774 in the validation set, which were higher than those of the 3D CNN model (AUC =0.709, ACC = 0.717). Among all the models, the nnU-Net model performed best, with an AUC of 0.835 and an ACC of 0.830 in the validation set, which was significantly higher than the primary physician's diagnosis (ACCs ranged from 0.757 to 0.811) (P<0.01). Conclusions: The proposed nnU-Net model could be an end-to-end, non-invasive, and accurate auxiliary diagnostic tool for the differentiation of pelvic and sacral OS and ES.
更多查看译文
关键词
Radiomics,deep learning,classification,osteosarcomas,Ewing's sarcomas (ES)
AI 理解论文
溯源树
样例
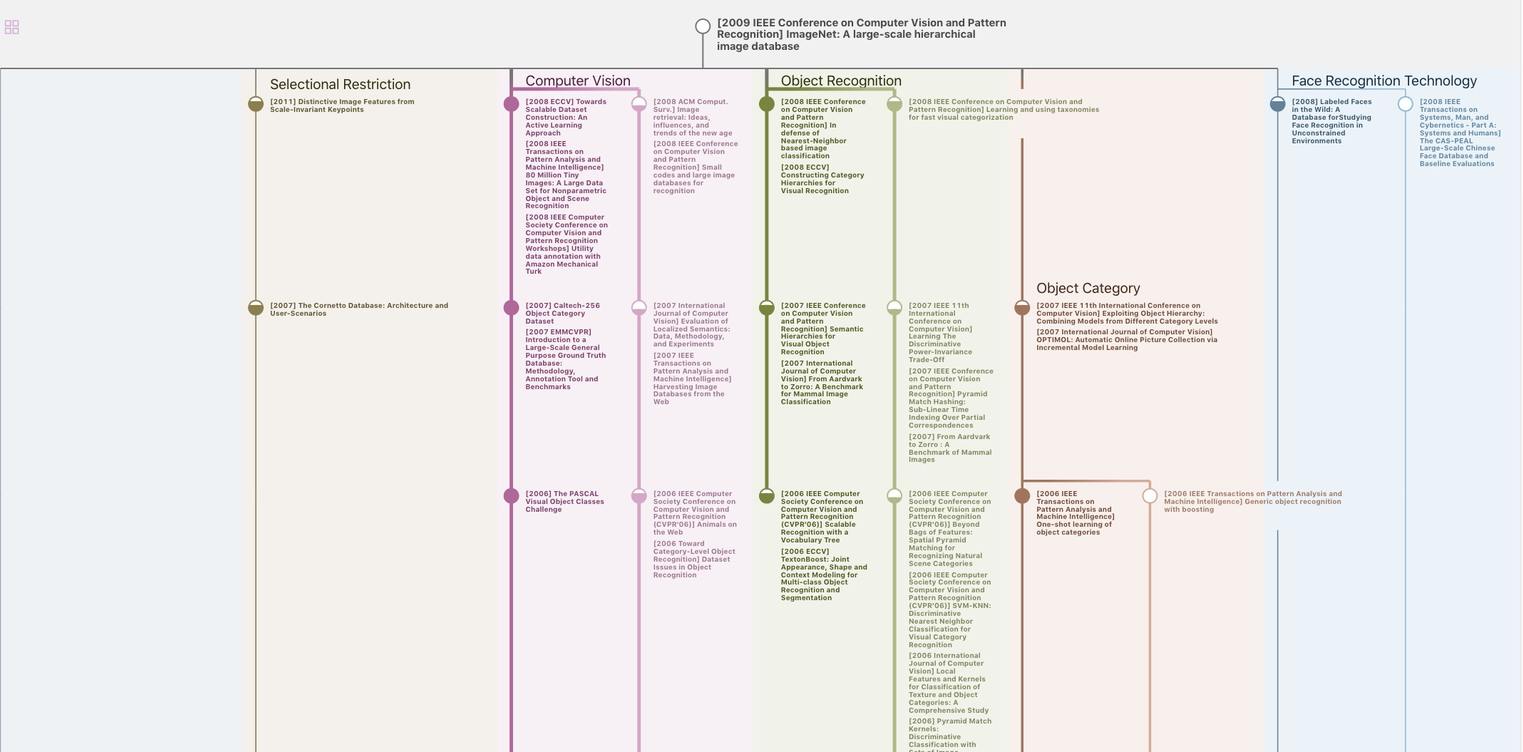
生成溯源树,研究论文发展脉络
Chat Paper
正在生成论文摘要