Machine learning based predictive model of early mortality in stage III and IV prostate cancer
medrxiv(2020)
摘要
Prostate cancer remains the third highest cause of cancer-related deaths. Metastatic prostate cancer could yield poor prognosis, however there is limited work on predictive models for clinical decision support in stage III and IV prostate cancer.
We developed a machine learning model for predicting early mortality in prostate cancer (survival less than 21 months after initial diagnosis). A cohort of 10,303 patients was extracted from the Surveillance, Epidemiology and End Results (SEER) program. Features were constructed in several domains including demographics, histology of primary tumor, and metastatic sites. Feature selection was performed followed by regularized logistic regression. The model was evaluated using 5-fold cross validation and achieved 75.2% accuracy with AUC 0.649. Of the 19 most predictive features, all of them were validated to be clinically meaningful for prediction of early mortality.
Our study serves as a framework for prediction of early mortality in patients with stage II and stage IV prostate cancer, and can be generalized to predictive modeling problems for other relevant clinical endpoints. Future work should involve integration of other data sources such as electronic health record and genomic or metabolomic data.
### Competing Interest Statement
The authors have declared no competing interest.
### Funding Statement
No external funding was received.
### Author Declarations
All relevant ethical guidelines have been followed; any necessary IRB and/or ethics committee approvals have been obtained and details of the IRB/oversight body are included in the manuscript.
Yes
All necessary patient/participant consent has been obtained and the appropriate institutional forms have been archived.
Yes
I understand that all clinical trials and any other prospective interventional studies must be registered with an ICMJE-approved registry, such as ClinicalTrials.gov. I confirm that any such study reported in the manuscript has been registered and the trial registration ID is provided (note: if posting a prospective study registered retrospectively, please provide a statement in the trial ID field explaining why the study was not registered in advance).
Yes
I have followed all appropriate research reporting guidelines and uploaded the relevant EQUATOR Network research reporting checklist(s) and other pertinent material as supplementary files, if applicable.
Yes
Public data was used.
更多查看译文
AI 理解论文
溯源树
样例
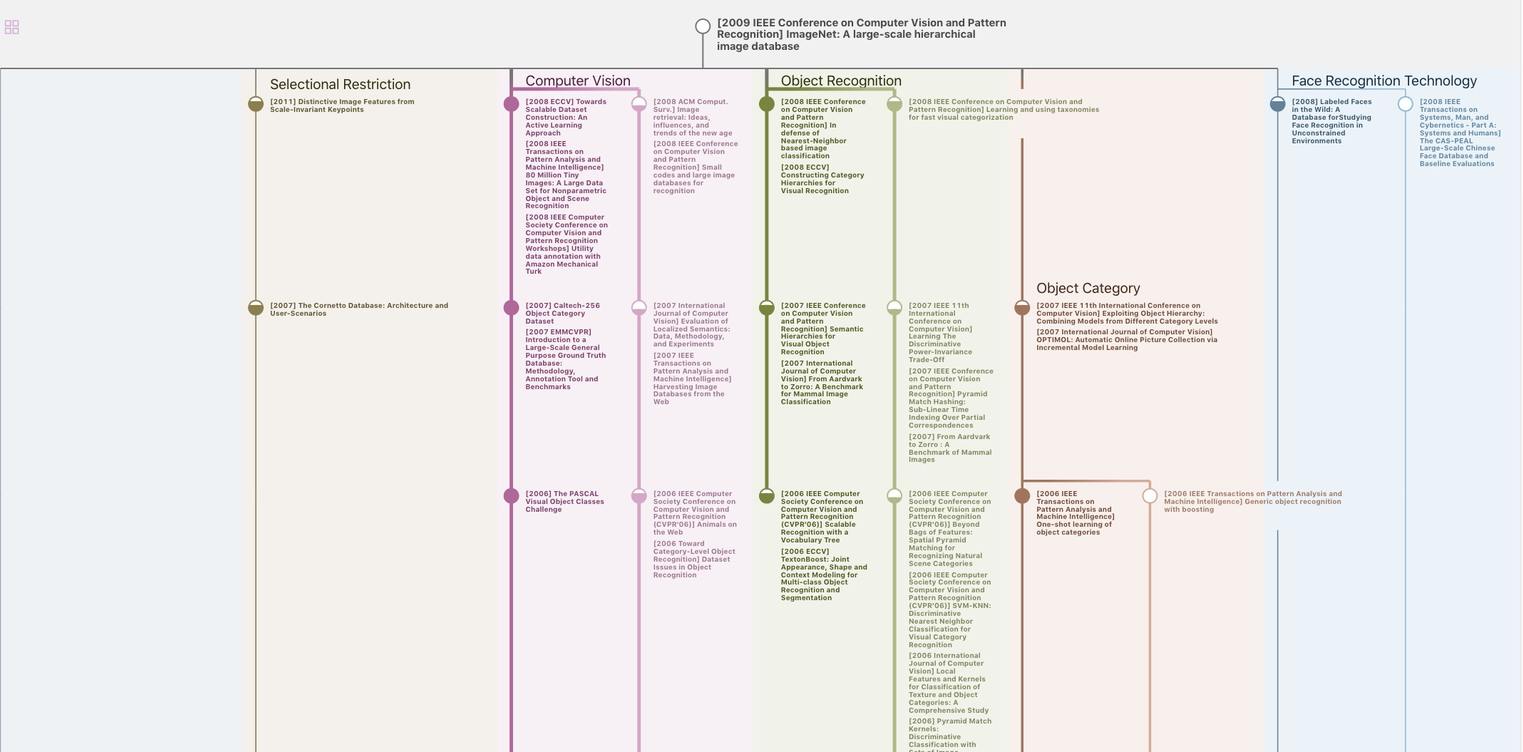
生成溯源树,研究论文发展脉络
Chat Paper
正在生成论文摘要