Retrospective Assessment of Deep Neural Networks for Skin Tumor Diagnosis
medrxiv(2020)
摘要
BACKGROUND The aim of this study was to validate the performance of algorithm () for the diagnosis of benign and malignant skin tumors.
METHODS With external validation dataset (43 disorders; 40,331 clinical images from 10,426 patients; January 1, 2008 – March 31, 2019), we compared the prediction of algorithm with the clinical diagnosis of 65 attending physicians at the time of biopsy request.
RESULTS For binary-task classification of determining malignancy, the AUC of the algorithm was 0.863(95% CI 0.852-0.875) with unprocessed clinical photographs. The sensitivity/specificity of the algorithm at the predefined high-sensitivity and high-specificity threshold were 79.1%(76.9-81.4)/76.9%(76.1-77.8) and 62.7%(59.9- 65.5)/90.0%(89.4-90.6), respectively. The sensitivity/specificity calculated by the clinical diagnosis of attending physicians were 88.1%/83.8%(Top-3) and 70.2%/95.6%(Top-1), which were superior to those of algorithm.
For multi-task classification, the mean Top-1,2,3 accuracies of the algorithm were 42.6±20.7%, 56.1±22.8%, 61.9±22.9%, and those of clinical diagnosis were 65.4±17.7%, 73.9±16.6%, 74.7±16.6%, respectively.
In the reader test with images from 30-patients batches, the sensitivity / specificity of the algorithm at the predefined threshold were 66.9%±30.2% / 87.4%±16.5%. The sensitivity / specificity derived from the first diagnosis of 44 the participants were 65.8%±33.3% / 85.7%±11.0%, which were comparable with those of the algorithm (Wilcoxon signed-rank test; P=0.61 / 0.097).
CONCLUSIONS Our algorithm could diagnose skin tumors at dermatologist-level when diagnosis was made solely with photographs, demonstrating its potential as a mass screening tool in telemedicine setting. However, due to limited data relevancy, the performance was inferior to that of actual medical examination. Clinical information should be integrated with imaging information to achieve more accurate predictions.
### Competing Interest Statement
The authors have declared no competing interest.
### Funding Statement
None
### Author Declarations
All relevant ethical guidelines have been followed; any necessary IRB and/or ethics committee approvals have been obtained and details of the IRB/oversight body are included in the manuscript.
Yes
All necessary patient/participant consent has been obtained and the appropriate institutional forms have been archived.
Yes
I understand that all clinical trials and any other prospective interventional studies must be registered with an ICMJE-approved registry, such as ClinicalTrials.gov. I confirm that any such study reported in the manuscript has been registered and the trial registration ID is provided (note: if posting a prospective study registered retrospectively, please provide a statement in the trial ID field explaining why the study was not registered in advance).
Yes
I have followed all appropriate research reporting guidelines and uploaded the relevant EQUATOR Network research reporting checklist(s) and other pertinent material as supplementary files, if applicable.
Yes
The images used to test the neural networks described in the manuscript are subject to privacy regulations and cannot be made available in totality. The test subset may be available upon a reasonable request and an approval of IRB of the originating university hospital.
更多查看译文
AI 理解论文
溯源树
样例
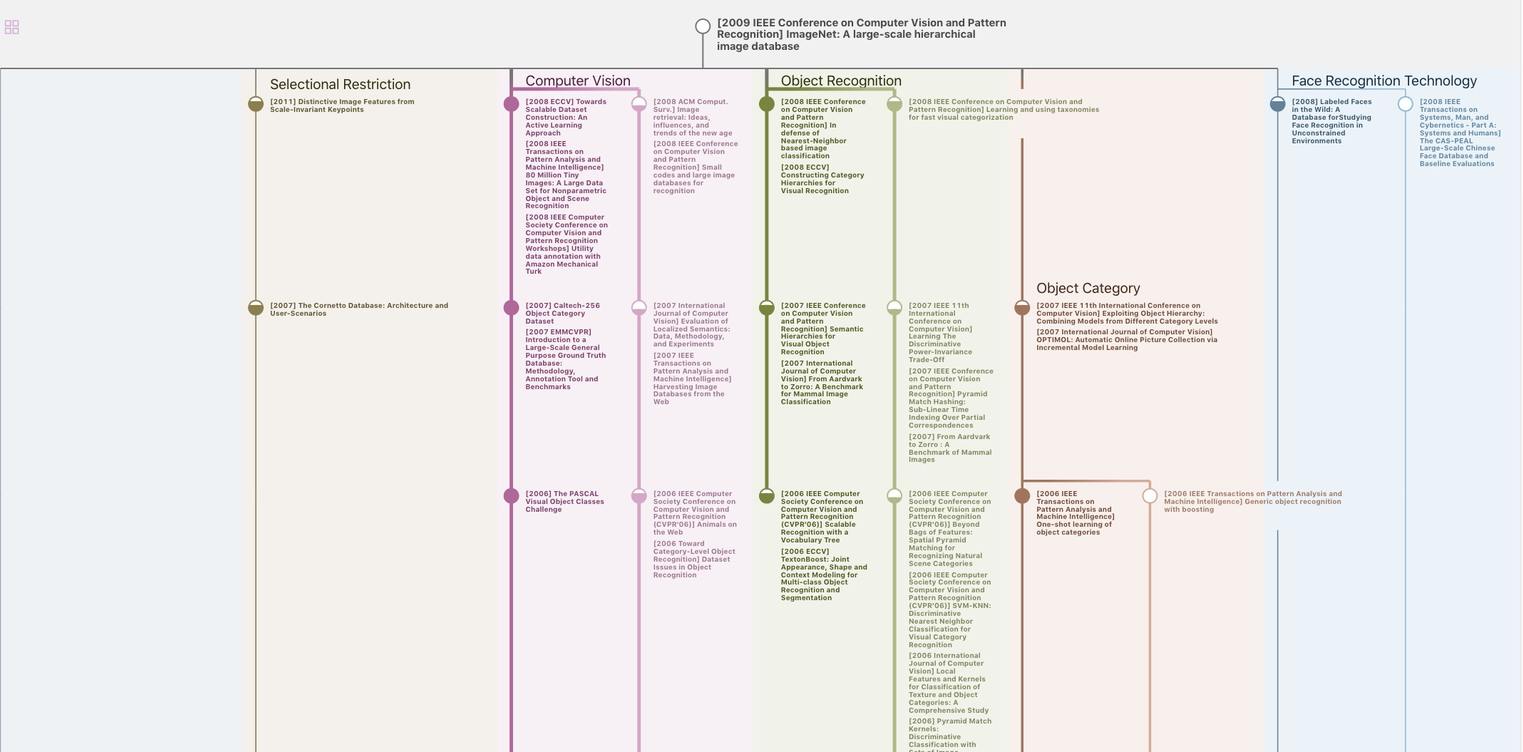
生成溯源树,研究论文发展脉络
Chat Paper
正在生成论文摘要