Non-intrusive Status Prediction of Residential Appliances using Supervised Learning Techniques
2023 6th International Conference on Energy Conservation and Efficiency (ICECE)(2023)
摘要
Recent demand side management (DSM) research have focused a lot of attention on energy conservation due to environmental concerns and growing energy costs. Several studies have shown that educating users about energy-hungry equipment can result in significant energy savings. In non-intrusive load monitoring (NILM), aggregate demand, often acquired from the smart meter, is used to calculate the electricity usage of the various appliances. Using aggregate consumer data from the smart meter, this study proposes six supervised learning algorithms (Decision Tree, Linear Discriminant, k-Nearest Neighbors, Quadratic Discriminant, Support Vector Machine, Ensemble) for predicting the working condition of various household appliances. The typical Pecan Street data is utilized to test the proposed methodologies, and several assessment measures are employed to compare the outcomes. K Nearest Neighbors and Support Vector Machine outperform other proposed algorithms, according on comparison results. This approach can help with energy prediction of individual loads based on aggregate demand, which is beneficial for getting consumers to adopt energy-saving measures.
更多查看译文
关键词
NILM,load disaggregation,energy conservation,supervised learning
AI 理解论文
溯源树
样例
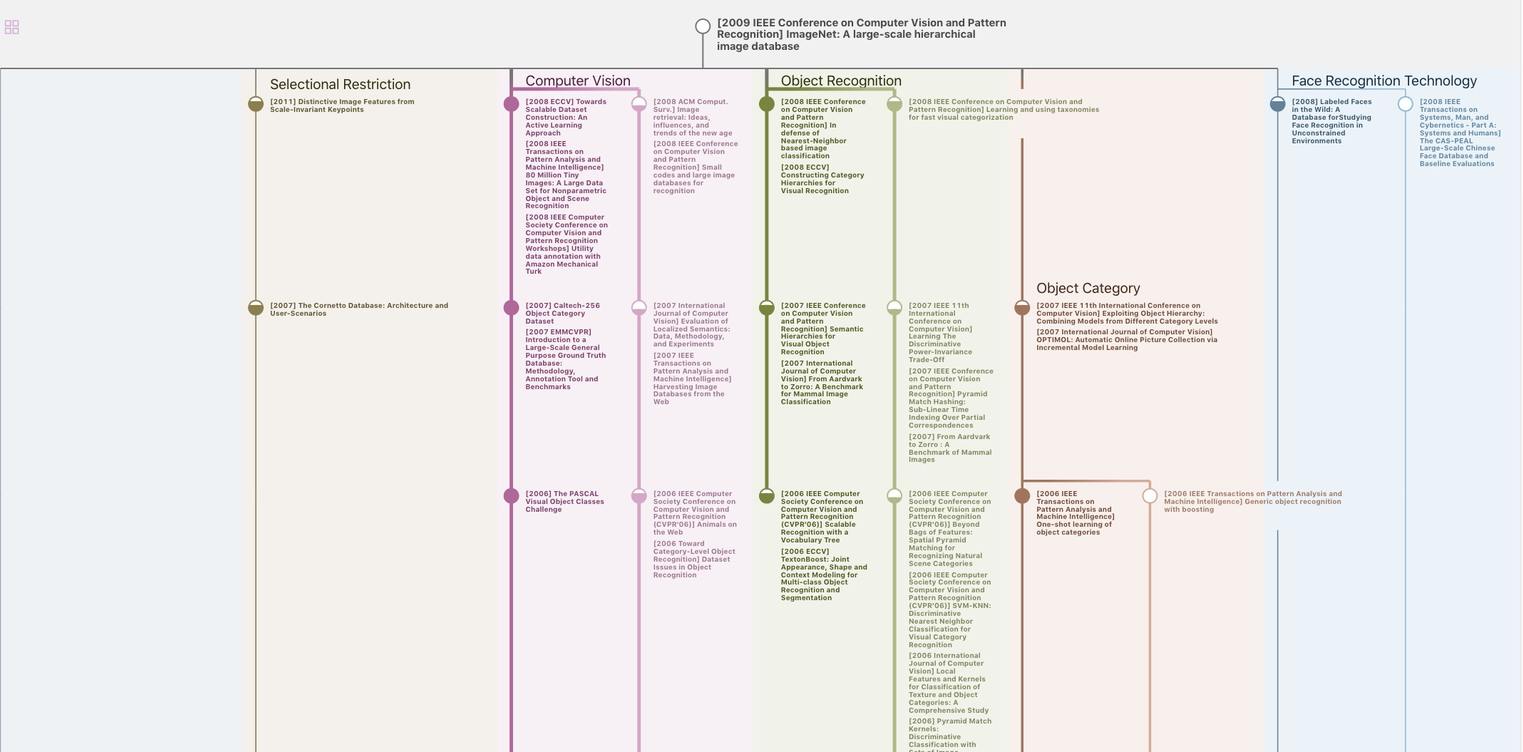
生成溯源树,研究论文发展脉络
Chat Paper
正在生成论文摘要