Autoencoder-Based Anomaly Detection for Time Series Data in Complex Systems
2022 IEEE Asia Pacific Conference on Circuits and Systems (APCCAS)(2022)
摘要
In this paper, we present a new anomaly detection method for time-series data in complex systems such as power grid and cellular networks. The proposed anomaly detection method is developed following unsupervised learning, where an AutoEncoder based on Gated Recurrent Units (GRU-AE) is trained to reconstruct a time-series of interest, and anomalies are detected via detecting exceptionally large reconstruction errors. A multi-timestamp stacking method is adopted to reduce the number of time steps in the GRU-AE to facilitate the training of the model and a new training scheme with random shuffling is proposed to prevent overfitting. The proposed GRU-AE based detector is applied in multiple time scales to detect different types of anomalies. Numerical results obtained via time-series data from real cellular network demonstrate the performance of the proposed method.
更多查看译文
关键词
time series,anomaly detection,AutoEncoder,multi-timestamp stacking,random shuffling
AI 理解论文
溯源树
样例
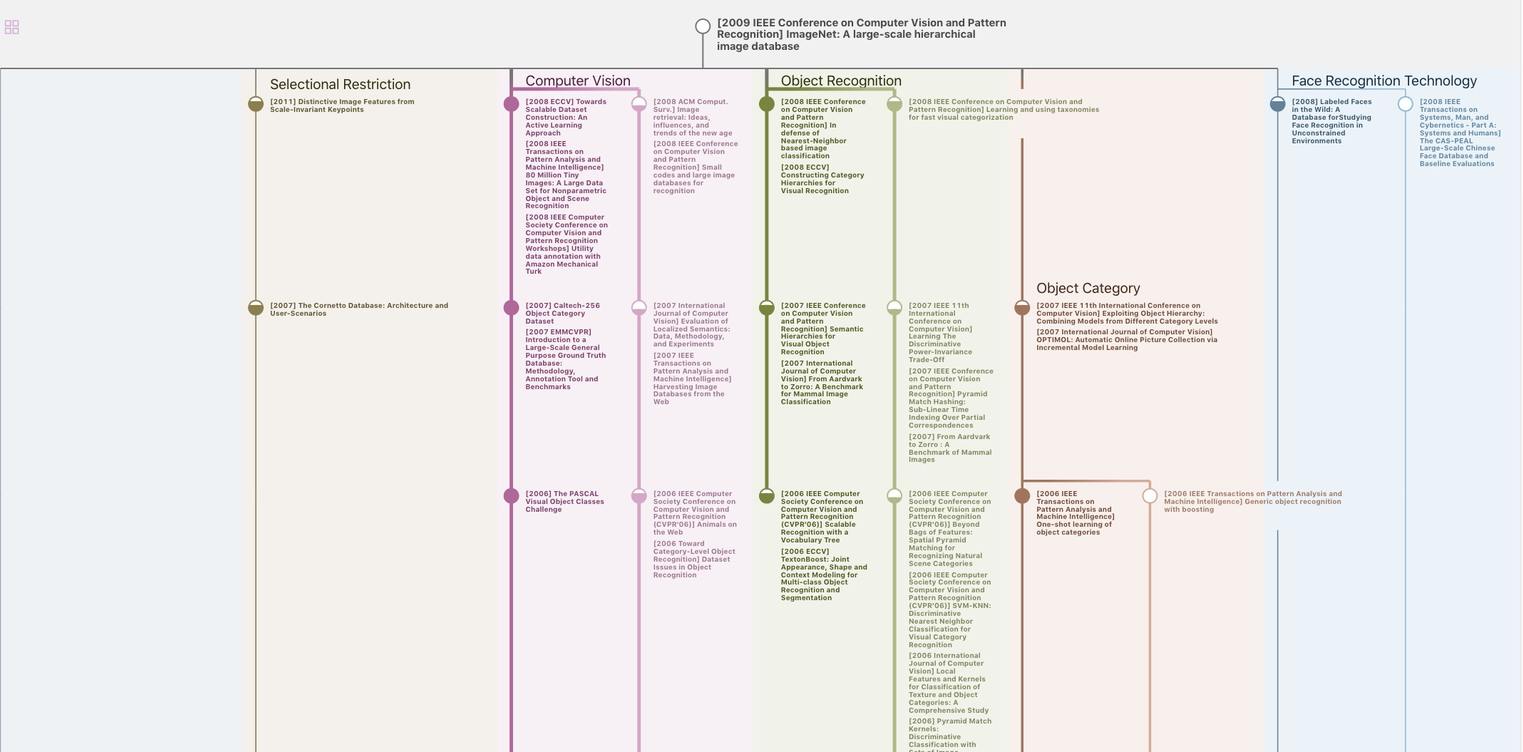
生成溯源树,研究论文发展脉络
Chat Paper
正在生成论文摘要