Optimising Faster R-CNN Training to Enable Video Camera Compression for Assisted and Automated Driving Systems
2022 2nd International Conference on Robotics, Automation and Artificial Intelligence (RAAI)(2022)
摘要
Advanced driving assistance systems based on only one camera or one RADAR are evolving into the current assisted and automated driving functions delivering SAE Level 2 and above capabilities. A suite of environmental perception sensors is required to achieve safe and reliable planning and navigation in future vehicles equipped with these capabilities. The sensor suite, based on several cameras, LiDARs, RADARs and ultrasonic sensors, needs to be adequate to provide sufficient (and redundant, depending on the level of driving automation) spatial and temporal coverage of the environment around the vehicle. However, the data amount produced by the sensor suite can easily exceed a few tens of Gb/s, with a single ‘average’ automotive camera producing more than 3 Gb/s. It is therefore important to consider leveraging traditional video compression techniques as well as to investigate novel ones to reduce the amount of video camera data to be transmitted to the vehicle processing unit(s). In this paper, we demonstrate that lossy compression schemes, with high compression ratios (up to 1:1,000) can be applied safely to the camera video data stream when machine learning based object detection is used to consume the sensor data. We show that transfer learning can be used to re-train a deep neural network with H.264 and H.265 compliant compressed data, and it allows the network performance to be optimised based on the compression level of the generated sensor data. Moreover, this form of transfer learning improves the neural network performance when evaluating uncompressed data, increasing its robustness to real world variations of the data.
更多查看译文
关键词
perception sensors,unmanned vehicles,assisted and automated driving,neural networks,intelligent systems,autonomous systems
AI 理解论文
溯源树
样例
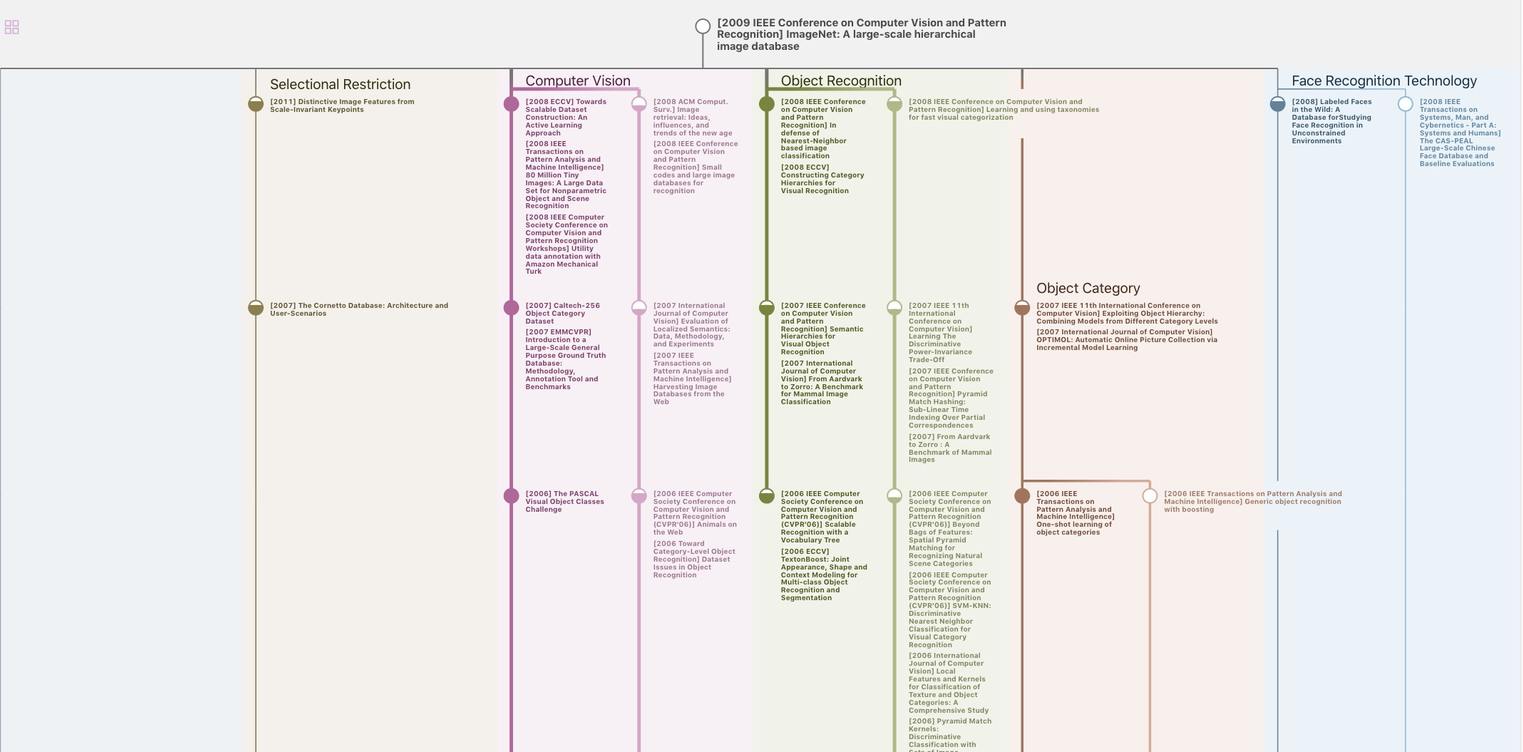
生成溯源树,研究论文发展脉络
Chat Paper
正在生成论文摘要