Feature Selection and Multi-task Learning for Pedestrian Crossing Prediction
2022 16th International Conference on Signal-Image Technology & Internet-Based Systems (SITIS)(2022)
摘要
Understanding pedestrians’ behaviour is a major challenge for autonomous vehicles in urban environments. An intelligent driving system needs to recognize intentions and anticipate future actions. For the task of pedestrian crossing prediction, we aim to predict the crossing of a pedestrian in traffic from visual features, such that an oncoming vehicle has sufficient time to react. In this work, we assess the efficacy of different input modalities such as human poses, bounding boxes, ego vehicle speed and image-based features for pedestrian crossing prediction. Our findings indicate that image-based features are less effective than suggested in the literature and that our newly generated human poses improve pedestrian crossing prediction. Furthermore, we present a neural network architecture based on recurrent units and multi-task learning which demonstrates that the joint training of multiple tasks has beneficial influence on the capability to identify crossing pedestrians. We evaluate our methods based on the public PIE and JAAD datasets, and generate models on par with state-of-the-art methods with a limited set of features.
更多查看译文
关键词
Pedestrian Crossing Prediction,Behaviour Analysis,Recurrent Neural Networks,Multi-task Learning
AI 理解论文
溯源树
样例
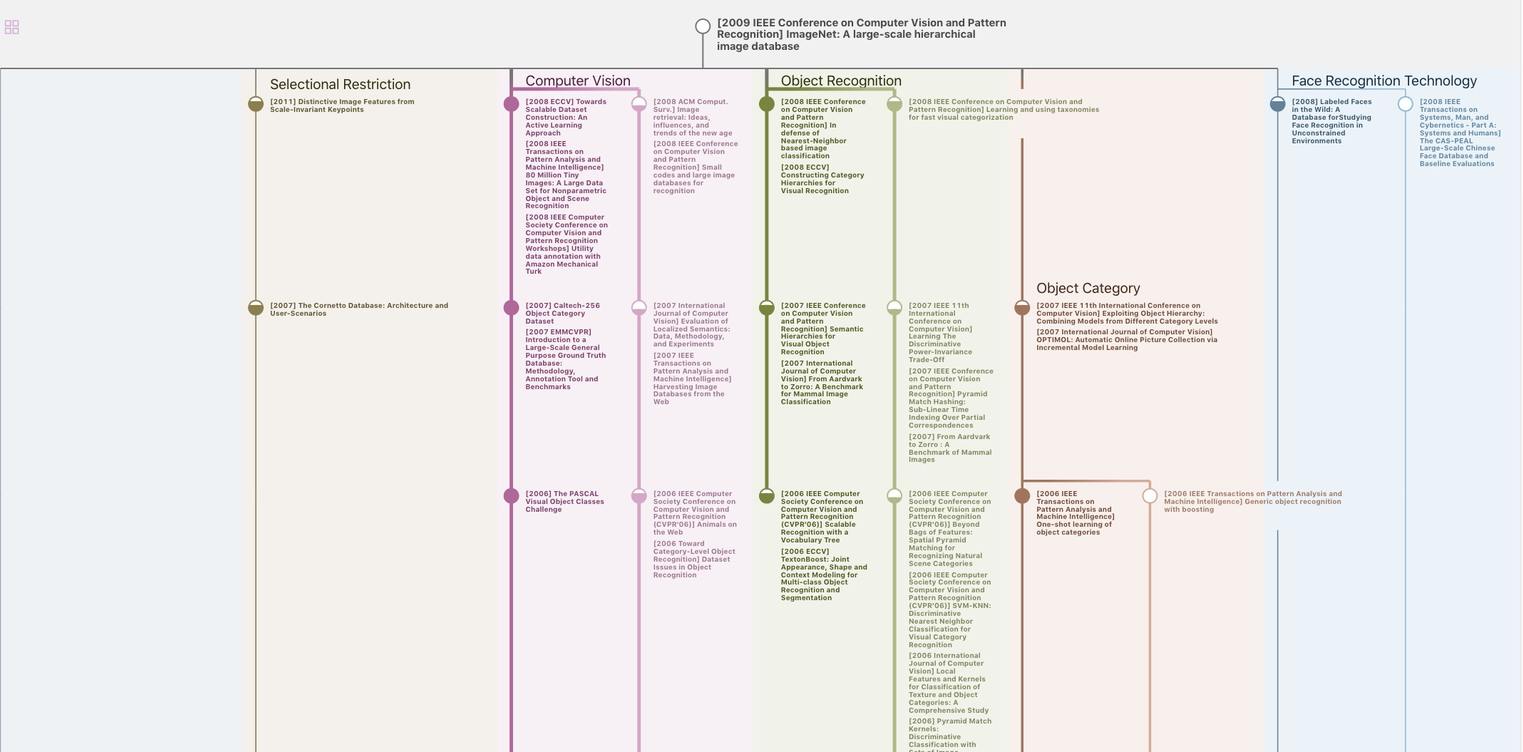
生成溯源树,研究论文发展脉络
Chat Paper
正在生成论文摘要