Star-Net: a Multi-Branch Convolutional Network for Multiple Source Image Segmentation
2022 16th International Conference on Signal-Image Technology & Internet-Based Systems (SITIS)(2022)
摘要
We present Star-Net, a multi-branch convolutional network architecture to deal with the multiple-source (multi-modal) image segmentation. It is composed of several satellite networks, one per source, connected in the corresponding layers through a central unit whose role is to calculate and assign the weights to the sources according to their relevance. In each layer of the network, the weights are different, case-specific and dynamically calculated. With this architecture, we reward the relevant sources, penalizing the less relevant ones. Star-Net takes into account the non-linear behaviour of the image interpretation, so as the active role of one source in a layer can be reduced in another, possibly growing up again in a following layer. When used in the field of multimodal Magnetic Resonance Imaging (MRI) segmentation, we have found that Star-Net is capable to speed up the training and improving the performance compared to traditional CNN architectures. Additionally and more importantly, it allows to perform case-specific analyses of network activation and increases network transparency.
更多查看译文
关键词
CNN,multiple source imaging,multimodal imaging,ensemble of CNNs,dense networks,network transparency,MRI
AI 理解论文
溯源树
样例
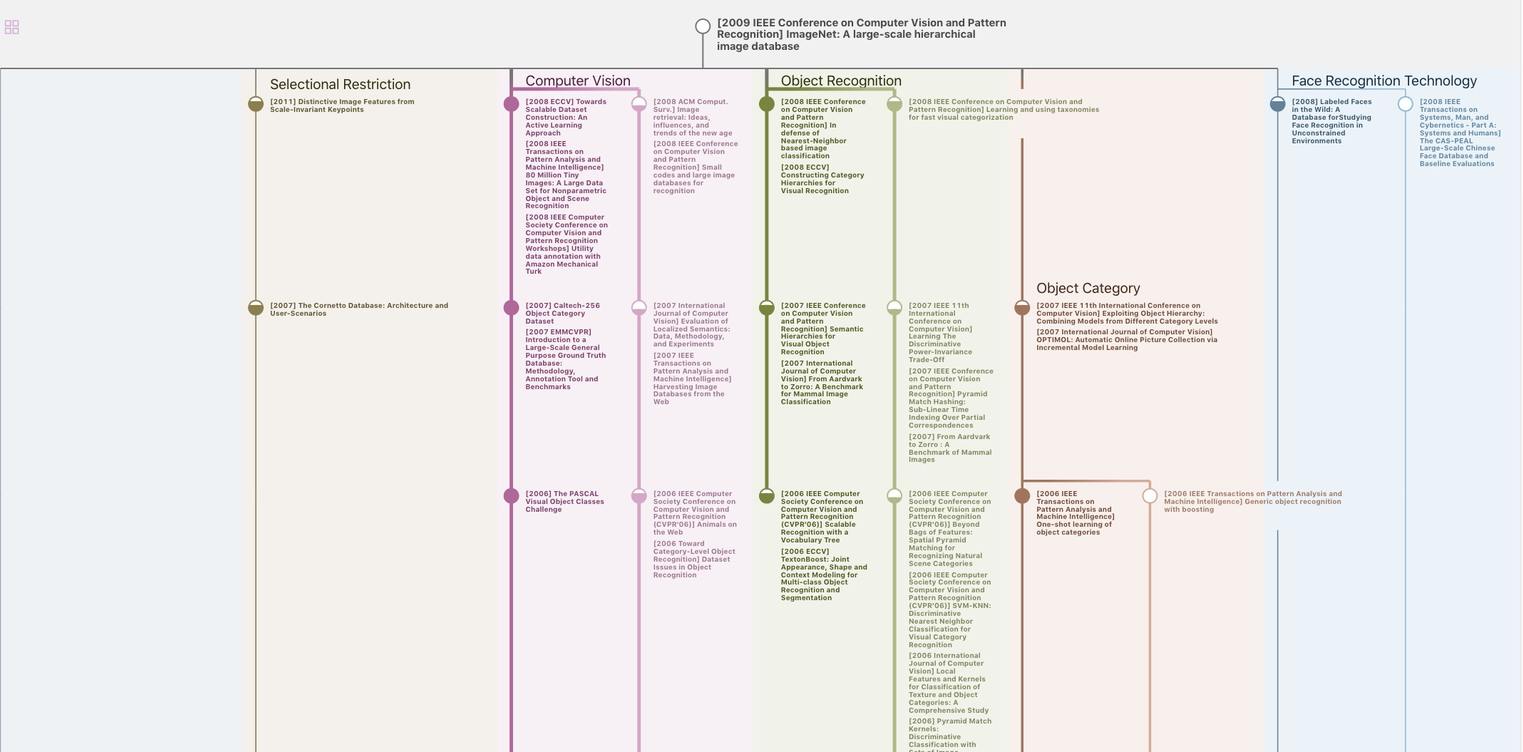
生成溯源树,研究论文发展脉络
Chat Paper
正在生成论文摘要