Net Load Forecast based on Behind-the-Meter Disaggregation of Smart Meter Data
2023 57th Annual Conference on Information Sciences and Systems (CISS)(2023)
摘要
As the penetration of behind-the-meter (BTM) rooftop solar energies continues to increase in power distribution systems, it is of paramount importance for load serving entities and system operators to forecast net loads in the system. In this paper, novel algorithms for training high-performance predictors for day-ahead net load forecasting are developed. Importantly, the overall method only utilizes metered net load data and does not require any monitoring data of solar generation. Methodologically, the net load data trace is disaggregated into estimated BTM solar and load traces, based on which separate predictors are then trained for solar generation and load forecasting exploiting their distinct natures, respectively. For solar generation forecasting, time data, weather forecast, and potentially solar irradiance forecast are used as input features of the predictor. For load forecasting, time data, weather forecast, and judiciously chosen load data in the recent past are used as input features of the predictor. The two predictors' outputs are combined to produce the final net load forecast. The developed method is comprehensively evaluated based on two real-world smart meter data sets from Ithaca, NY and Clifton park, NY, respectively. High accuracy of day-ahead net load forecast is demonstrated.
更多查看译文
AI 理解论文
溯源树
样例
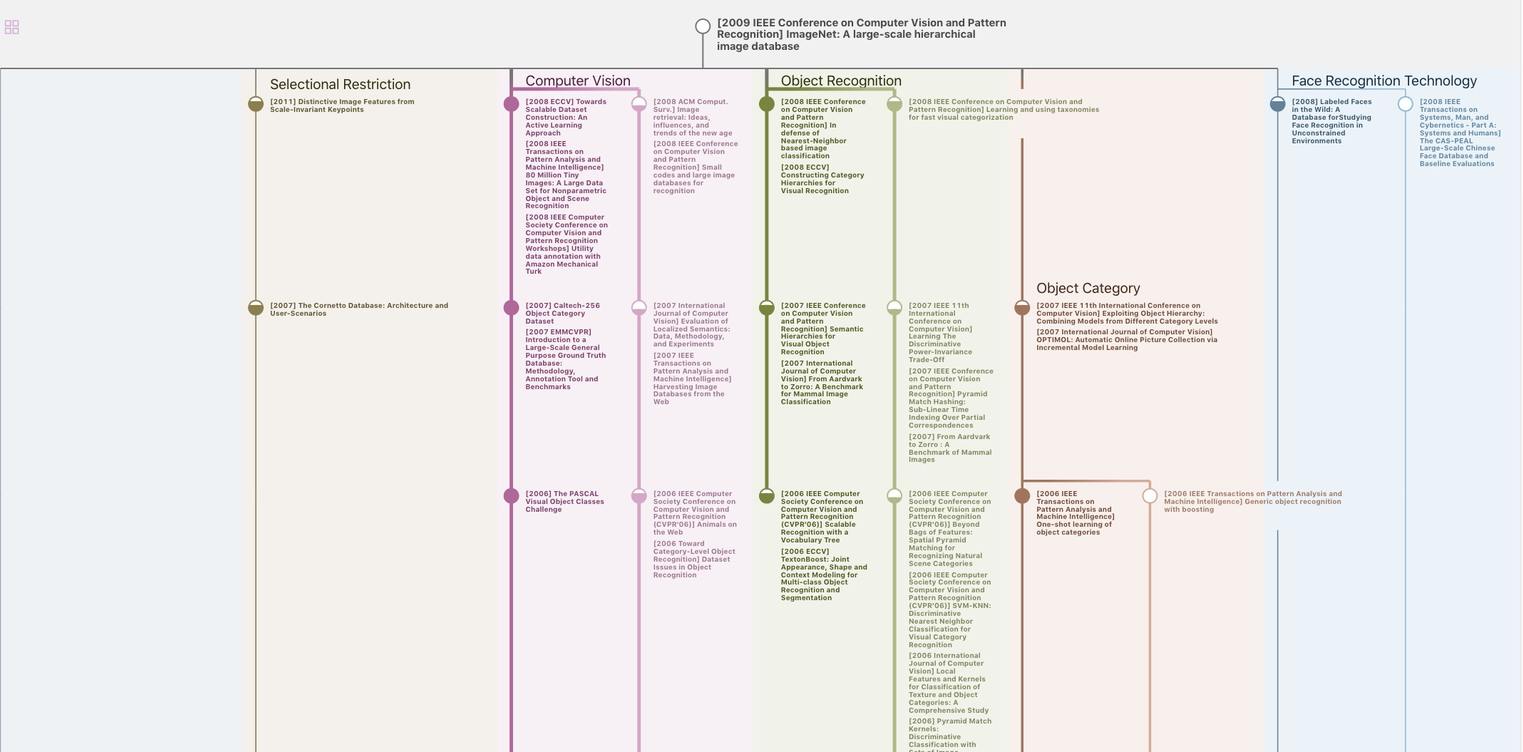
生成溯源树,研究论文发展脉络
Chat Paper
正在生成论文摘要