Stochastic Mean-Shift for Speaker Clustering
2023 57th Annual Conference on Information Sciences and Systems (CISS)(2023)
摘要
This work is a continuation of our previous work on short segments speaker clustering. We have shown that mean-shift clustering algorithm with probabilistic linear discriminant analysis (PLDA) score as the similarity measure, can be a good approach for this task. While the standard mean-shift clustering algorithm is a deterministic algorithm, in this work we suggest a stochastic version to train the mean-shift. The quality of the clustering is measured by the value K, which is a geometric mean of average cluster purity (ACP) and average speaker purity (ASP). We test the proposed algorithm in the range of 3 to 60 speakers and show that it outperforms the deterministic mean-shift in all cases.
更多查看译文
关键词
Speaker clustering,mean-shift,stochastic mean-shift,stochastic training,PLDA
AI 理解论文
溯源树
样例
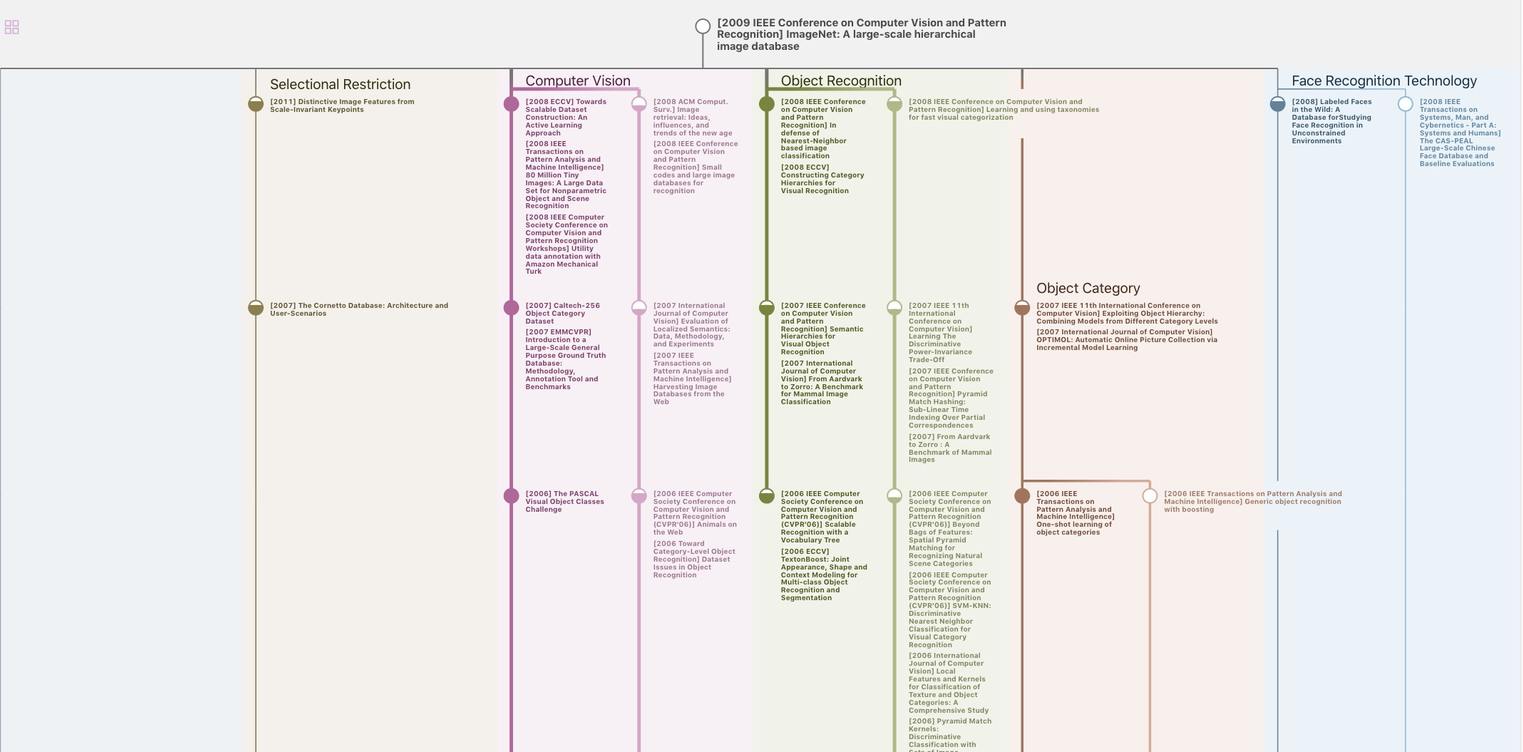
生成溯源树,研究论文发展脉络
Chat Paper
正在生成论文摘要