Towards an Improved Hyperdimensional Classifier for Event-Based Data
2023 57th Annual Conference on Information Sciences and Systems (CISS)(2023)
摘要
Hyperdimensional Computing (HDC) is an emerging neuroscience-inspired framework wherein data of various modalities can be represented uniformly
$\text{in}$
high-dimensional space as long, redundant holographic vectors. When equipped with the proper Vector Symbolic Architecture (VSA) and applied to neuromorphic hardware, HDC-based networks have been demonstrated to be capable of solving complex visual tasks with substantial energy efficiency gains and increased robustness to noise when compared to standard Artificial Neural Networks (ANNs). HDC has shown potential to be used with great efficacy for learning based on spatiotemporal data from neuromorphic sensors such as the Dynamic Vision Sensor (DVS), but prior work has been limited in this arena due to the complexity and unconventional nature of this type of data as well as difficulty choosing the appropriate VSA to hypervectorize spatiotemporal information. We present a bipolar HD encoding mechanism designed for encoding spatiotemporal data, which captures the contours of DVS-generated time surfaces created by moving objects by fitting to them local surfaces which are individually encoded into HD vectors and bundled into descriptive high-dimensional representations. We conclude with a sketch of the structure and training/inference pipelines associated with an HD classifier, predicated on our proposed HD encoding scheme, trained for the complex real-world task of pose estimation from event camera data.
更多查看译文
AI 理解论文
溯源树
样例
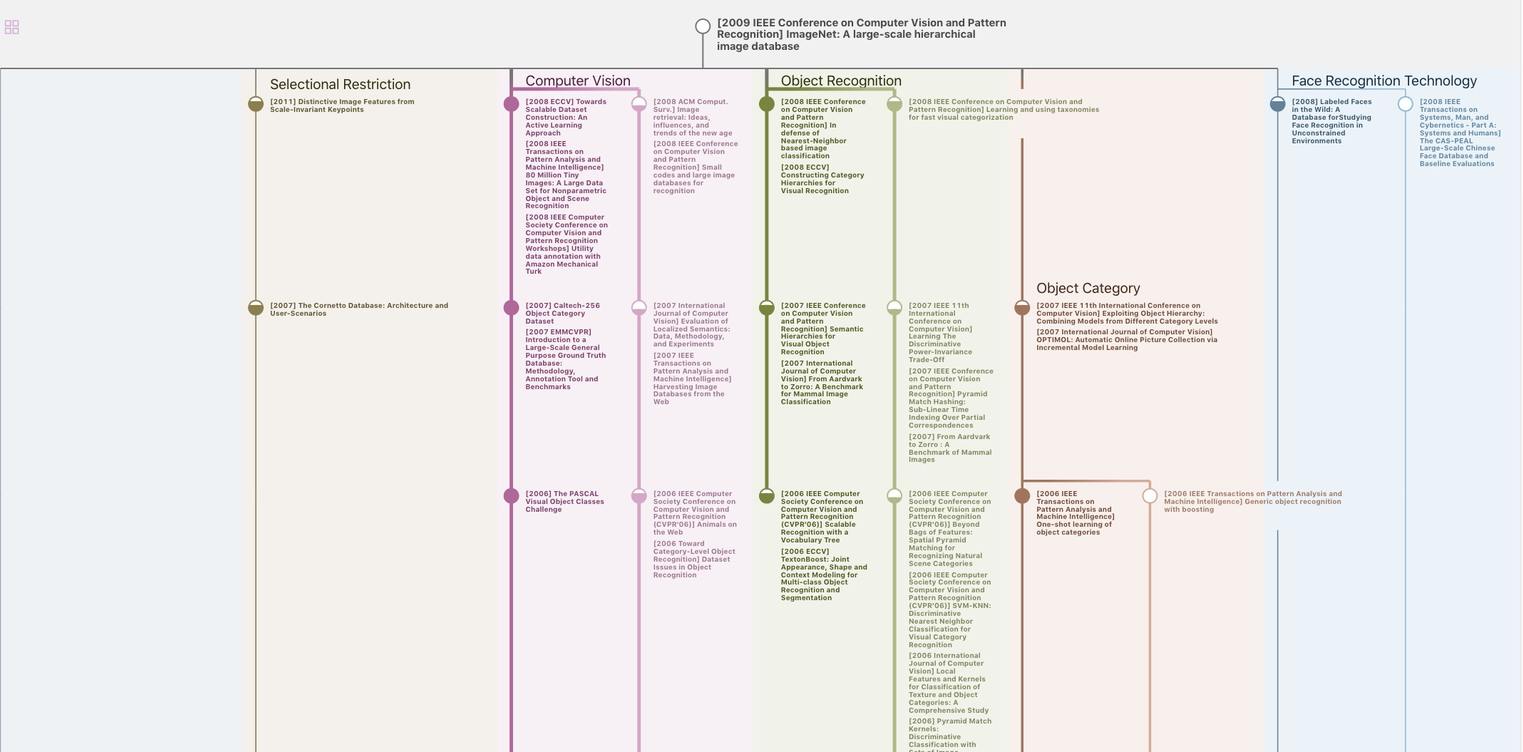
生成溯源树,研究论文发展脉络
Chat Paper
正在生成论文摘要