A Unified Deep Learning Diagnostic Architecture for Big Data Healthcare Analytics
2023 IEEE 15th International Symposium on Autonomous Decentralized System (ISADS)(2023)
摘要
Healthcare automation is evolving rapidly as can been seen in the recent popularity of e-health or digital health systems. The massive amount of health related data produced by these systems has given rise to the field of health informatics. The World Health organization (WHO) decomposes SMARThealth as Standards-based, Machine-readable, Adaptive, Requirementsbased, and Testable, and provides guidelines for digital health. Heterogeneous and big health data health that flows into the cloud requires considerations for uniformity of structure to allow for interoperability and generalizability for universal use and analysis. This research proposes a deep-learning architecture for disease diagnosis that considers Diabetes Mellitus (DM) as a case study. Three corpuses containing DM patient data are considered which are prepared and processed using extensive data warehousing techniques and labeled with ICD-10-CM diagnostic codes. Extraction of desired health data is through a unified data model for healthcare that is in compliance with HL7 FHIR v4.0 schema. Our contributions are two-fold: First, three big data cloud analytical models are proposed and validated on the unified corpora and second the maximum possible diseases specific to a single or multiple DM patients have been diagnosed with a 100% accuracy using deep multinomial/multi-label distribution learning (DMDL).
更多查看译文
关键词
deep learning,diagnostic architecture,healthcare analytics,HPC,big data
AI 理解论文
溯源树
样例
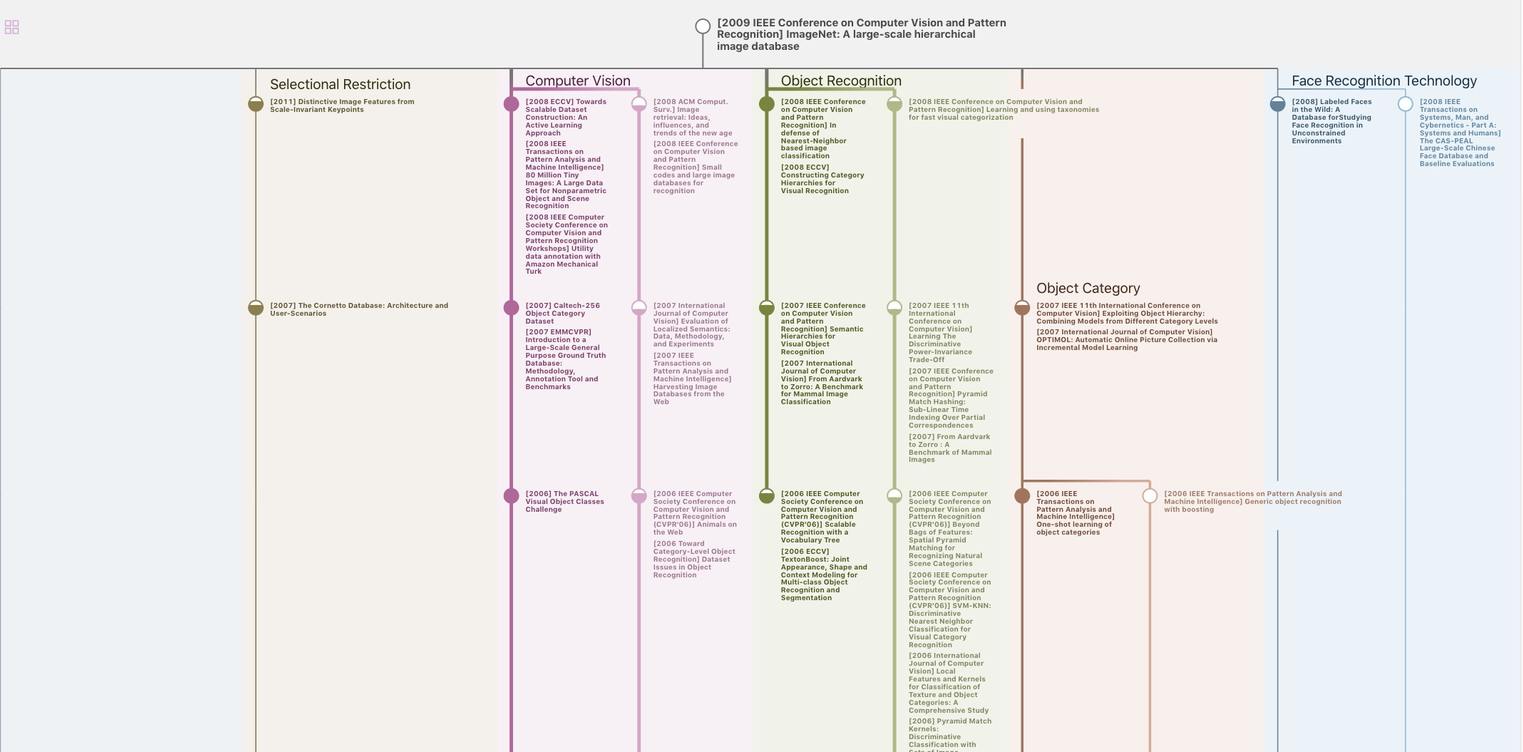
生成溯源树,研究论文发展脉络
Chat Paper
正在生成论文摘要