Machine Learning-based Electric Vehicle User Behavior Prediction
2023 IEEE IAS Global Conference on Renewable Energy and Hydrogen Technologies (GlobConHT)(2023)
摘要
Electric Vehicles (EVs) have grown rapidly over the past decade but the integration of large-scale electric vehicles into the power grid is still a challenge. Overloading and harmonic distortions because of EV charging may impact the grid stability and may create grid issues. Power industry needs to get ready for the EV roll-out. One of the key actions to prepare for greater EV penetration and mitigate grid issues is smart charge scheduling of EVs to manage the charging load efficiently. Charge scheduling of EVs highly depends on EV users' behavior, so prediction of users' behavior is critical. Two of the important parameters, i.e., energy consumption and session duration are predicted with the Mean Absolute Error (MAE) of 1.45 kWh and 66.5 minutes respectively. These predictions are based on weekly, monthly and yearly historical public charging dataset of Adaptive Charging Network (ACN) implemented at Caltech USA. Ensemble machine learning models with base models as Random Forest (RF), Support Vector Machine (SVM) and XGBoost are used to improve the results. In contrast with the existing literature, this work utilizes the predicted session duration and weather data from local weather station along with the nearby events data and public holidays. Prediction errors are reduced in comparison to existing works with the improved data processing pipeline and feature selection.
更多查看译文
关键词
Electric Vehicles (EVs),Machine Learning,Smart Charge Scheduling,Smart Grid,Charging behavior
AI 理解论文
溯源树
样例
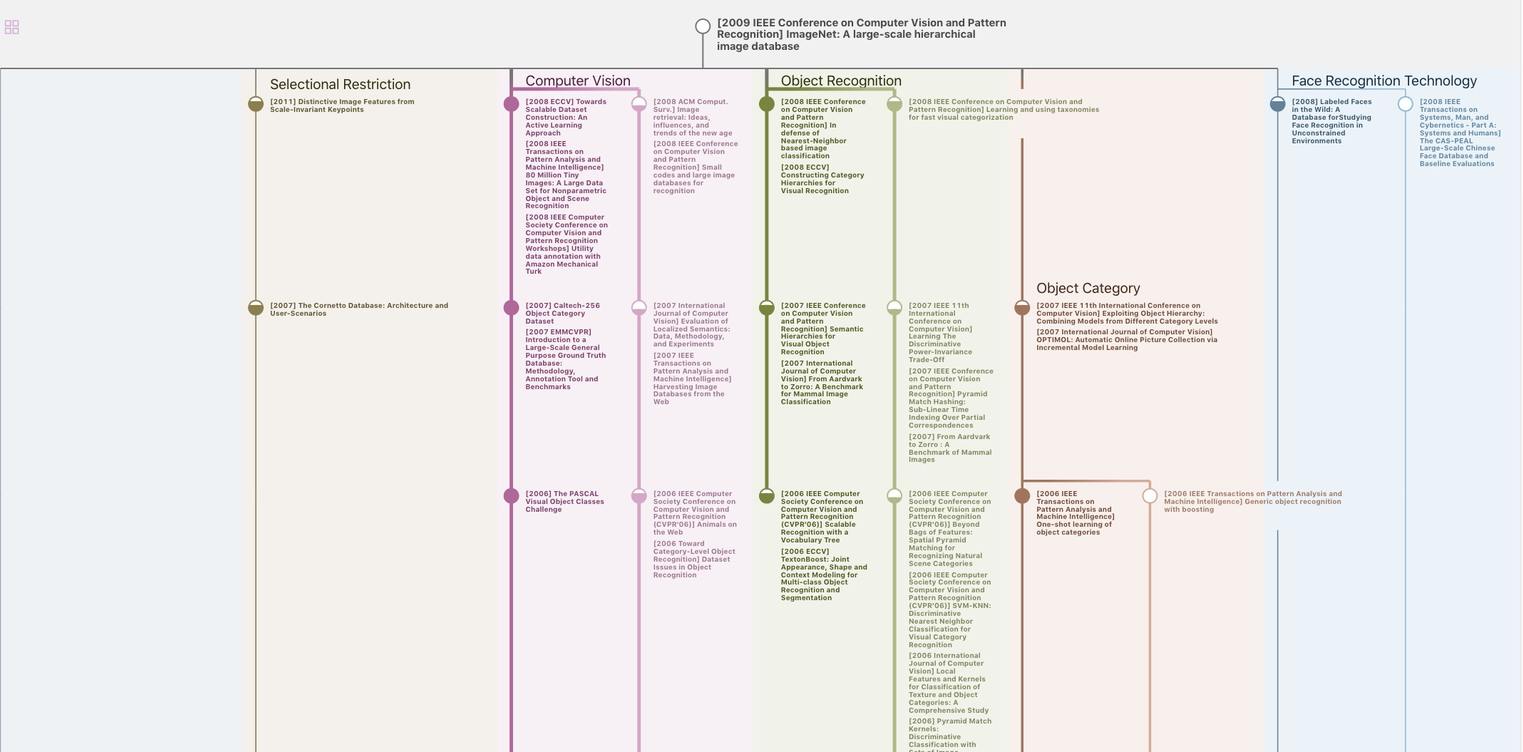
生成溯源树,研究论文发展脉络
Chat Paper
正在生成论文摘要