Learning Accurate Performance Predictors for Ultrafast Automated Model Compression
CoRR(2023)
摘要
In this paper, we propose an ultrafast automated model compression framework called SeerNet for flexible network deployment. Conventional non-differen-tiable methods discretely search the desirable compression policy based on the accuracy from exhaustively trained lightweight models, and existing differentiable methods optimize an extremely large supernet to obtain the required compressed model for deployment. They both cause heavy computational cost due to the complex compression policy search and evaluation process. On the contrary, we obtain the optimal efficient networks by directly optimizing the compression policy with an accurate performance predictor, where the ultrafast automated model compression for various computational cost constraint is achieved without complex compression policy search and evaluation. Specifically, we first train the performance predictor based on the accuracy from uncertain compression policies actively selected by efficient evolutionary search, so that informative supervision is provided to learn the accurate performance predictor with acceptable cost. Then we leverage the gradient that maximizes the predicted performance under the barrier complexity constraint for ultrafast acquisition of the desirable compression policy, where adaptive update stepsizes with momentum are employed to enhance optimality of the acquired pruning and quantization strategy. Compared with the state-of-the-art automated model compression methods, experimental results on image classification and object detection show that our method achieves competitive accuracy-complexity trade-offs with significant reduction of the search cost. Code is available at https://github.com/ZiweiWangTHU/SeerNet .
更多查看译文
关键词
Automated model compression,Performance predictor,Compression policy optimization,Uncertainty estimation,Evolutionary search
AI 理解论文
溯源树
样例
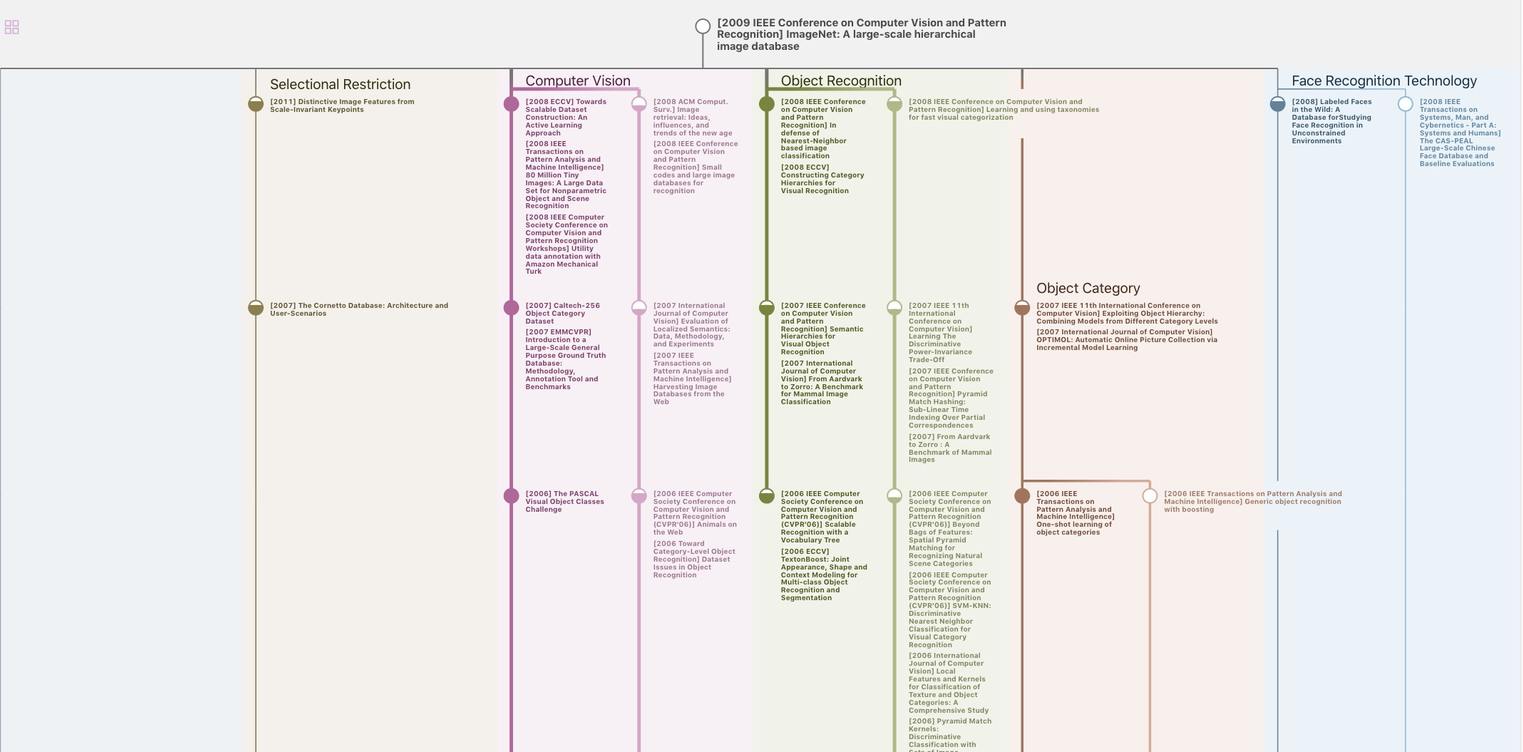
生成溯源树,研究论文发展脉络
Chat Paper
正在生成论文摘要