Maximal Fairness
CoRR(2023)
摘要
Fairness in AI has garnered quite some attention in research, and increasingly also in society. The so-called "Impossibility Theorem" has been one of the more striking research results with both theoretical and practical consequences, as it states that satisfying a certain combination of fairness measures is impossible. To date, this negative result has not yet been complemented with a positive one: a characterization of which combinations of fairness notions are possible. This work aims to fill this gap by identifying maximal sets of commonly used fairness measures that can be simultaneously satisfied. The fairness measures used are demographic parity, equal opportunity, false positive parity, predictive parity, predictive equality, overall accuracy equality and treatment equality. We conclude that in total 12 maximal sets of these fairness measures are possible, among which seven combinations of two measures, and five combinations of three measures. Our work raises interest questions regarding the practical relevance of each of these 12 maximal fairness notions in various scenarios.
更多查看译文
关键词
Fairness, Fairness in AI, Fairness definitions, confusion tables, Combining Fairness definitions
AI 理解论文
溯源树
样例
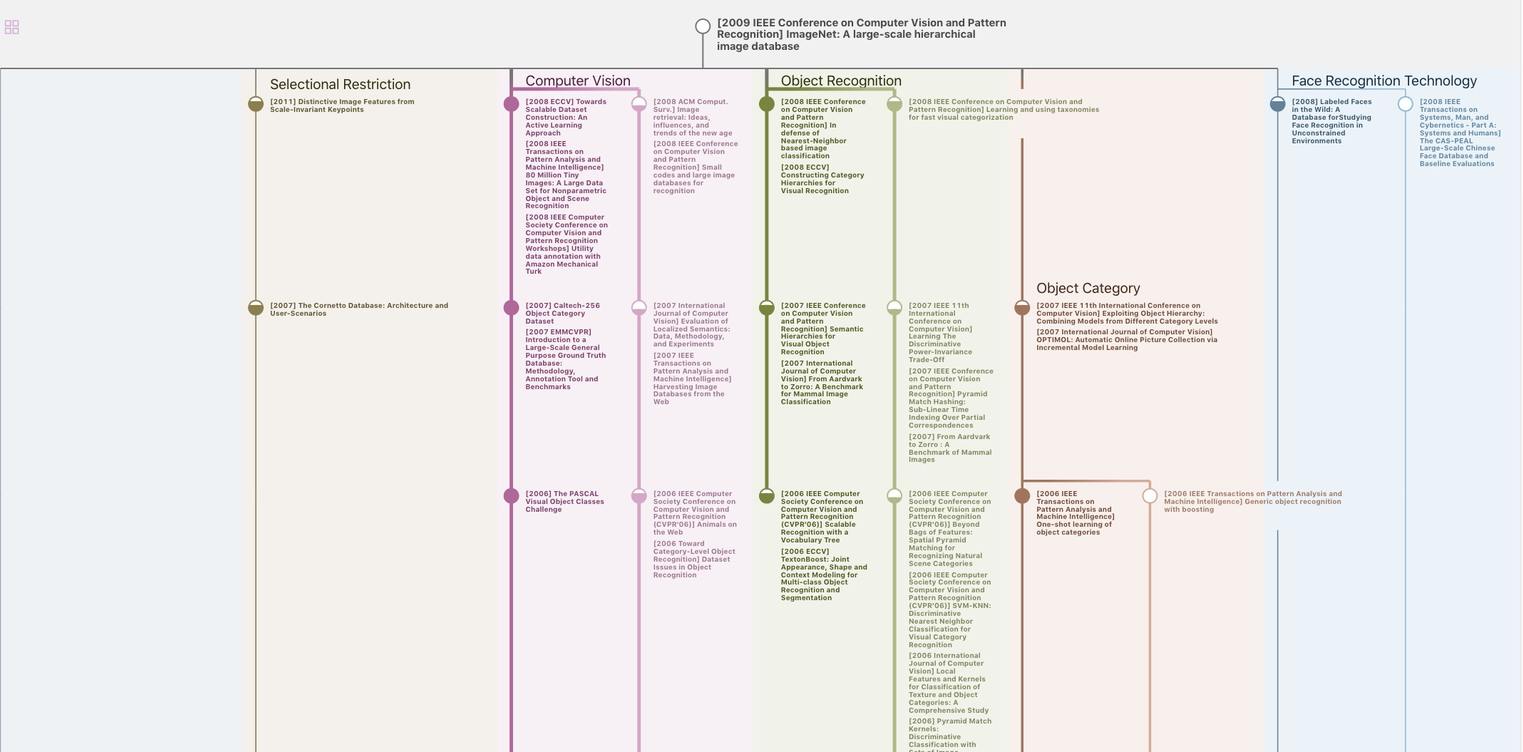
生成溯源树,研究论文发展脉络
Chat Paper
正在生成论文摘要