Pre-training on physical dynamics enhances indirect sensing in recurrent neural networks
2022 International Symposium on Micro-NanoMehatronics and Human Science (MHS)(2022)
摘要
A McKibben-type pneumatic artificial muscle (PAM), which can achieve a large contraction force, is a promising candidate for the actuation of soft robots. Currently, to control PAMs, many sensors are required for controlling factors such as the length and internal pressure of the PAM, and the load applied to it. To reduce the weight and cost of soft robots and keep their characteristics intact, it is important to reduce the number of sensors as much as possible. In this study, we propose a machine learning scheme that can accurately predict PAM length using a small number of sensors. This is realized through the pre-training on the physical property of PAM in recurrent neural networks under the reservoir computing framework. First, we removed the load and length sensors and predicted the length of the PAM using only the internal pressure and electrical resistance sensors. The load applied to the PAM greatly affected its length, so the prediction accuracy was low, and the normalized mean square error (NMSE), which is an index of error, was NMSE =0.220. However, when the load was predicted from the internal pressure and electrical resistance, the load could be predicted with a high accuracy, and when self-feedback from this emulated load was added into the reservoir, the prediction accuracy of the length was greatly improved (NMSE =0.0330). In the end, we removed the load, length, and internal pressure sensors, and we predicted the length using only the electrical resistance sensor. The load and internal pressure were predicted from the electrical resistance, and these predicted values were fed back to the reservoir and used to predict the length. When the length was finally predicted, the prediction accuracy was higher than when it was predicted from just the electrical resistance. The mechanisms that supported this improvement and the role of feedback in the reservoir are discussed in detail.
更多查看译文
AI 理解论文
溯源树
样例
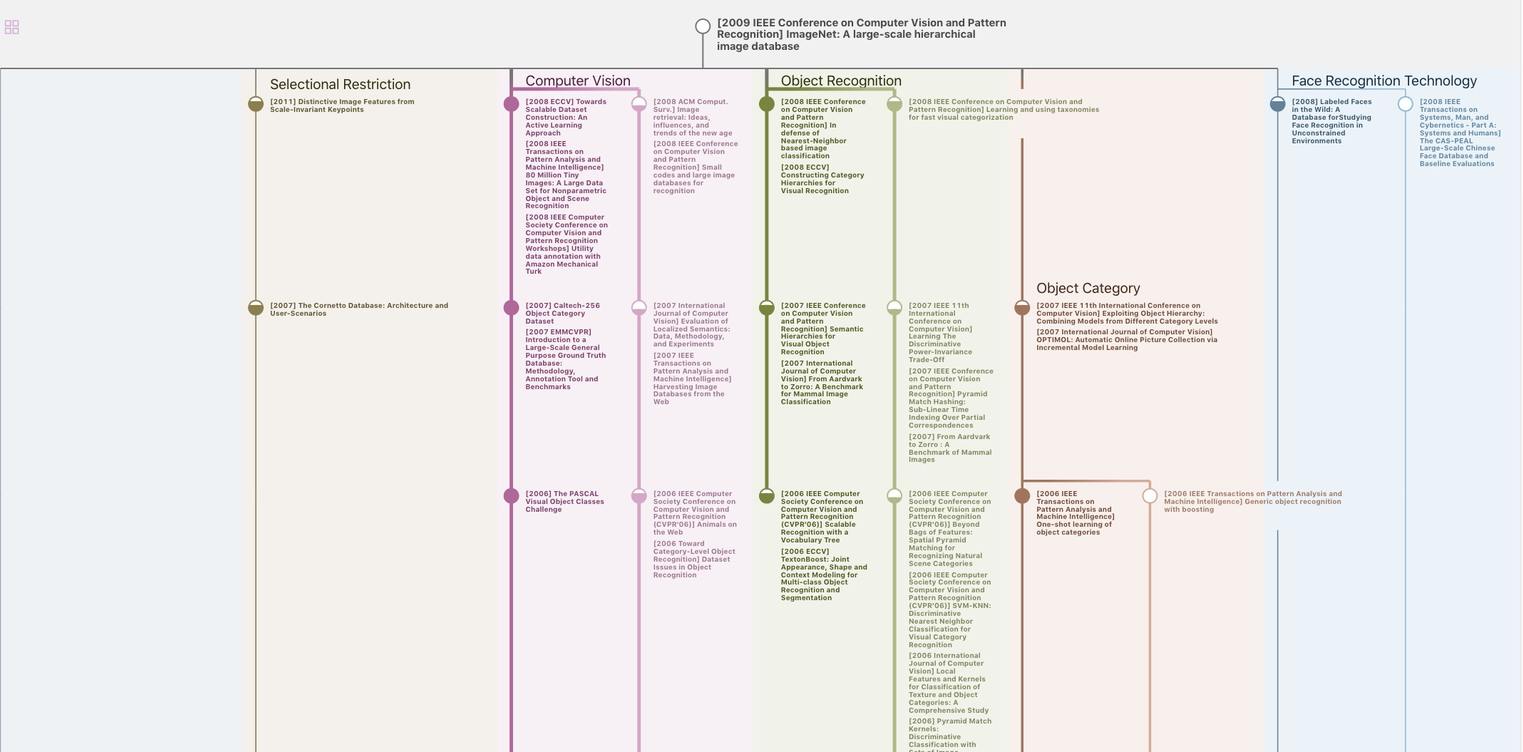
生成溯源树,研究论文发展脉络
Chat Paper
正在生成论文摘要