Post Hoc Evaluation of Probabilistic Model Forecasts: A COVID-19 Case Study
medrxiv(2021)
摘要
To combat the spread of coronavirus disease 2019 (COVID-19), decision-makers and the public may desire forecasts of the cases, hospitalizations, and deaths that are likely to occur. Thankfully, dozens of COVID-19 forecasting models exist and many of their forecasts have been made publicly available. However, there has been little published peer-reviewed information regarding the performance of these models and what is available has focused mostly on the performance of their central estimates (i.e., predictive performance). There has been little reported on the accuracy of their uncertainty estimates (i.e., probabilistic performance), which could inform users how often they would be surprised by observations outside forecasted confidence intervals. To address this gap in knowledge, we borrow from the literature on formally elicited expert judgment to demonstrate one commonly used approach for resolving this issue. For two distinct periods of the pandemic, we applied the Classical Model (CM) to evaluate probabilistic model performance and constructed a performance-weighted ensemble based on this evaluation. Some models which exhibited good predictive performance were found to have poor probabilistic performance, and vice versa. Only two of the nine models considered exhibited superior predictive and probabilistic performance. Additionally, the CM-weighted ensemble outperformed the equal-weighted and predictive-weighted ensembles. With its limited scope, this study does not provide definitive conclusions on model performance. Rather, it highlights the evaluation methodology and indicates the utility associated with using the CM when assessing probabilistic performance and constructing high performing ensembles, not only for COVID-19 modeling but other applications as well.
Significance Statement Coronavirus disease 2019 (COVID-19) forecasting models can provide critical information for decision-makers and the public. Unfortunately, little information on their performance has been published, particularly regarding the accuracy of their uncertainty estimates (i.e., probabilistic performance). To address this research gap, we demonstrate the Classical Model (CM), a commonly used approach from the literature on formally elicited expert judgment, which considers both the tightness of forecast confidence intervals and frequency in which confidence intervals contain the observation. Two models exhibited superior performance and the CM-based ensemble consistently outperformed the other constructed ensembles. While these results are not definitive, they highlight the evaluation methodology and indicate the value associated with using the CM when assessing probabilistic performance and constructing high performing ensembles.
### Competing Interest Statement
The authors have declared no competing interest.
### Funding Statement
Kyle J. Colonna's involvement was funded by the Harvard Population Health Sciences PhD scholarship. Roger M. Cooke's involvement was pro bono. John S. Evans' involvement was funded by the Department of Environmental Health and the Harvard Cyprus Initiative at the Harvard T.F. Chan School of Public Health.
### Author Declarations
I confirm all relevant ethical guidelines have been followed, and any necessary IRB and/or ethics committee approvals have been obtained.
Yes
The details of the IRB/oversight body that provided approval or exemption for the research described are given below:
No IRB/oversight body approval or exemption was necessary as the data is publicly available.
I confirm that all necessary patient/participant consent has been obtained and the appropriate institutional forms have been archived, and that any patient/participant/sample identifiers included were not known to anyone (e.g., hospital staff, patients or participants themselves) outside the research group so cannot be used to identify individuals.
Yes
I understand that all clinical trials and any other prospective interventional studies must be registered with an ICMJE-approved registry, such as ClinicalTrials.gov. I confirm that any such study reported in the manuscript has been registered and the trial registration ID is provided (note: if posting a prospective study registered retrospectively, please provide a statement in the trial ID field explaining why the study was not registered in advance).
Yes
I have followed all appropriate research reporting guidelines and uploaded the relevant EQUATOR Network research reporting checklist(s) and other pertinent material as supplementary files, if applicable.
Yes
Observed state COVID-19 mortality and case data was gathered from the Centers for Disease Control and Prevention (CDC) (43). State population and racial composition data was collected from one-year estimates from the Census Bureau's 2018 and 2019 American Community Survey (ACS) (44). For racial composition statistics and case rate data, please see SI appendix, Tables S3 and S4. Model forecasting data was gathered from the COVID-19 Forecast Hub's publicly available structured data storage repository on GitHub (8). For the model and ensemble predictions, their uncertainty distributions, and the subsequent observations of COVID-19 mortality, please see SI appendix, Tables S5 and S6.
更多查看译文
关键词
probabilistic model forecasts
AI 理解论文
溯源树
样例
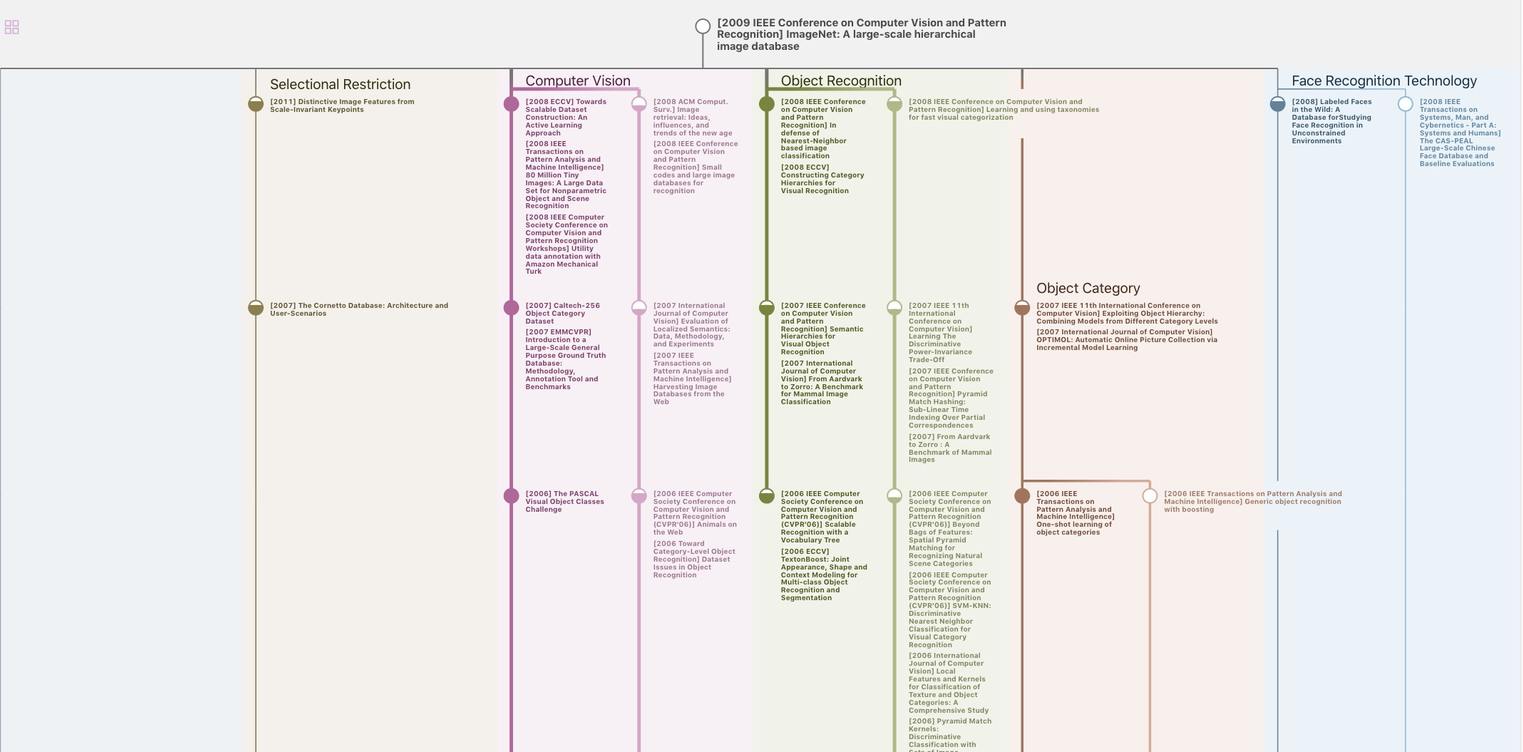
生成溯源树,研究论文发展脉络
Chat Paper
正在生成论文摘要