Deep Reinforcement Learning Algorithm for Latency-Oriented IIoT Resource Orchestration
IEEE Internet of Things Journal(2023)
摘要
Due to geographical factors and resource constraints, the traditional Internet architecture cannot meet the needs of the space–air–ground-integrated network (SAGIN) resource layout in the Industrial Internet of Things (IIoT) service. How to arrange network resources in SAGIN quickly and efficiently to meet the quality of service requirements of users has become a hot research topic in the industry. Based on the characteristics of SAGIN with multiple network segments, we convert the resource scheduling problem of SAGIN into a multidomain virtual network embedding (VNE) problem. This article proposes a latency-sensitive VNE algorithm based on deep reinforcement learning (DDRL-VNE) in the SAGIN environment. Unlike traditional latency optimization algorithms, we consider the effect of traffic size and hop count on latency when evaluating latency. We constructed a learning agent composed of a five-layer policy network and extracted a feature matrix as its training environment based on the network attributes of SAGIN. The node embedding is completed according to the probability that each node is embedded in the training, and then the breadth-first search strategy is used to complete the link embedding. The experimental results effectively illustrate the effectiveness of the algorithm in the SAGIN resource allocation problem.
更多查看译文
关键词
Deep reinforcement learning (DRL),Industrial Internet of Things (IIoT),latency,space–air–ground-integrated network (SAGIN)
AI 理解论文
溯源树
样例
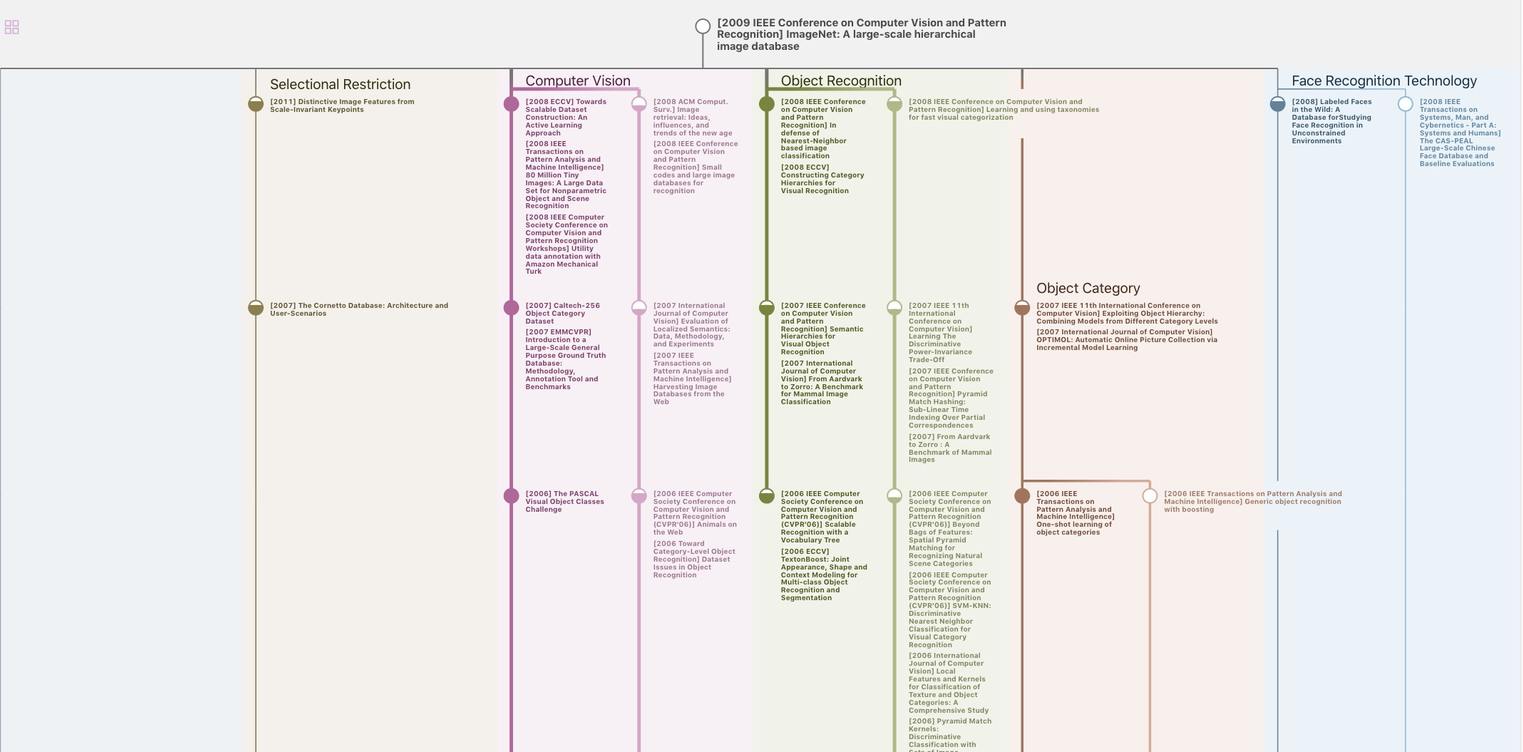
生成溯源树,研究论文发展脉络
Chat Paper
正在生成论文摘要