Computational Enhancements to the Mahalanobis-Taguchi System to Improve Fault Detection and Diagnostics
2023 Annual Reliability and Maintainability Symposium (RAMS)(2023)
摘要
SUMMARYFault detection and diagnostics (FDD) refers to identifying the abnormal operations of a machine and then determining the cause or causes of the fault. FDD is an important use case of Industrial Internet of Things (IIOT) technology. This paper introduces the Mahalanobis-Taguchi system (MTS) method and additional enhancements that apply to common data scenarios. Genichi Taguchi proposed the MTS method of diagnosing multivariate data. It has been successfully used for FDD and quality control in various industries, including electrical power, chemicals, and aerospace. The MTS method involves the following three steps.Step 1: The multivariate sensor data from a machine’s normal (or fault-free) operations are collected. The sensor variables are standardized, and the Mahalanobis distance (MD) of the observations from the center is calculated. These MD values define what is referred to as the Mahalanobis space of normal observations. The MTS method uses the Mahalanobis space as a reference scale for identifying outliers. This step can be considered the model training step.Step 2: New observations are scored by calculating their MD values. During scoring, the observations are first scaled using the mean and standard deviation of sensor variables by employing the machine’s normal operations data, which were collected in step 1. The MD computation in step 2 uses the correlation matrix of the normal operations data. An observation is an outlier, or the presence of a fault is indicated if that observation’s MD value is higher than the typical MD value of observations in normal or fault-free operations.Step 3: Fault diagnosis is performed by identifying important variables to improve model interpretability. A design-of-experiments approach is adopted for such identification by using orthogonal arrays and the signal-to-noise (S/N) ratio.In addition to these three steps, a data preprocessing step is added before training to enhance MTS capability in handling certain common data scenarios:Step 0: Three common data scenarios are addressed:•data that contain observations from both normal operations and faults•data that are multimodal or clustered•data with many correlated factors or data that have a singular covariance matrixIn this preprocessing step, we propose a robust outlier detection technique to handle data that contain observations from normal operations and faults. In the case of multimodal or clustered data, we apply a clustering method to address separate and distinct data distributions. We use a generalized inverse of the correlation matrix and implement a dimension reduction method for data with a high factor dimension, containing highly correlated factors or factors with a singular correlation matrix.Because the MTS method is an interpretable outlier detection technique, it is very attractive for FDD. This paper introduces the MTS method for FDD, discusses certain limitations of MTS, and offers enhancements to address those limitations.
更多查看译文
关键词
Mahalanobis-Taguchi System,Anomaly Detection,Fault Detection,Interpretable Results,Orthogonal Array
AI 理解论文
溯源树
样例
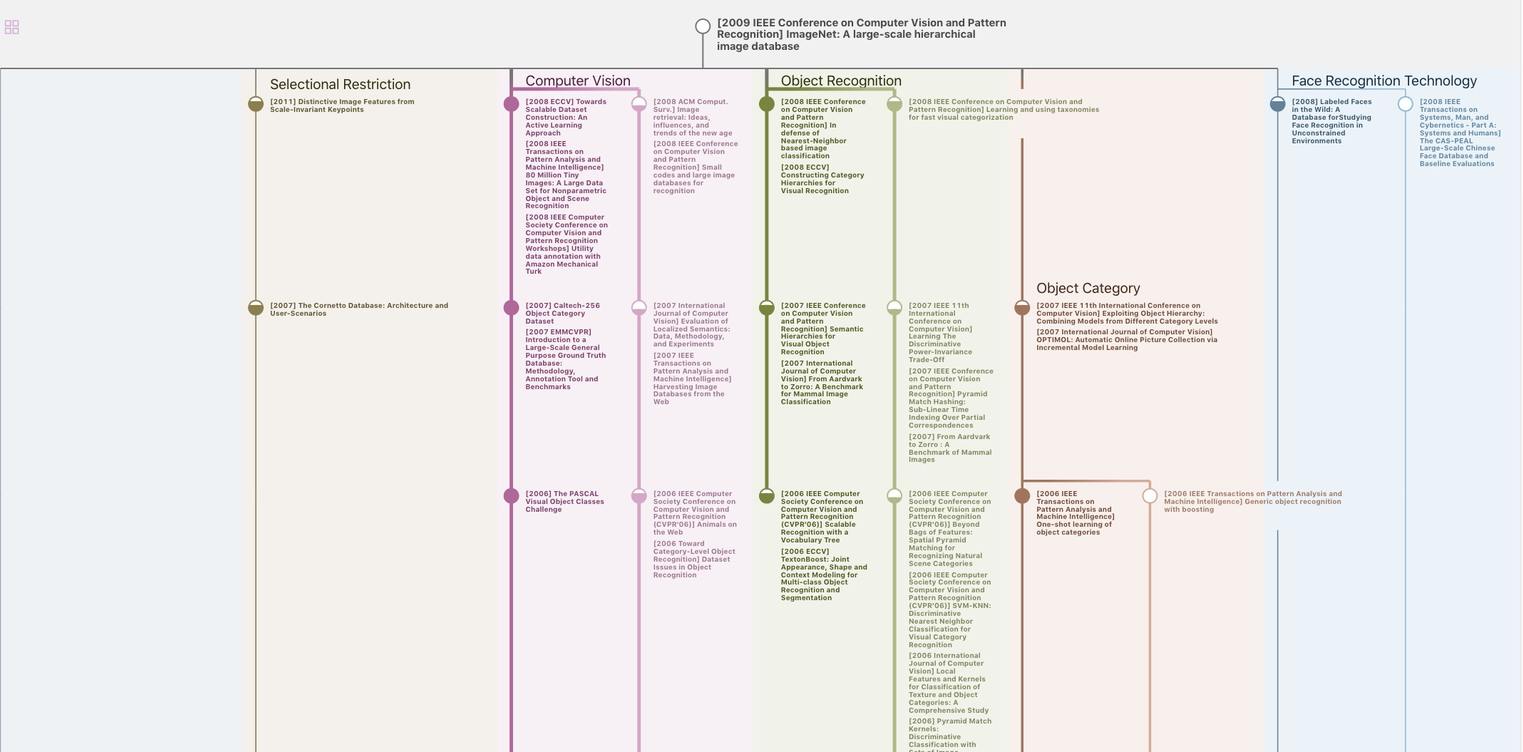
生成溯源树,研究论文发展脉络
Chat Paper
正在生成论文摘要