Social Network Data Publishing Model Satisfying Differential Privacy
2022 International Conference on Informatics, Networking and Computing (ICINC)(2022)
摘要
Social networks contain sensitive information, and direct press conferences disclose people’s privacy. The published social network data needs privacy protection. The currently released social network data has seriously damaged the network structure due to privacy protection. This paper proposes a social network graph publishing model that preserves the social network structure. This model builds an uncertainty graph based on privacy protection probability to generate social network data for publication. It is proved theoretically that the social network graph publishing model satisfies the definition of differential privacy. This paper uses three real social network datasets to evaluate the effectiveness of the proposed method, showing that the method effectively retains the network structure.
更多查看译文
关键词
Social network,differential privacy,uncertainty graph,Louvain community division
AI 理解论文
溯源树
样例
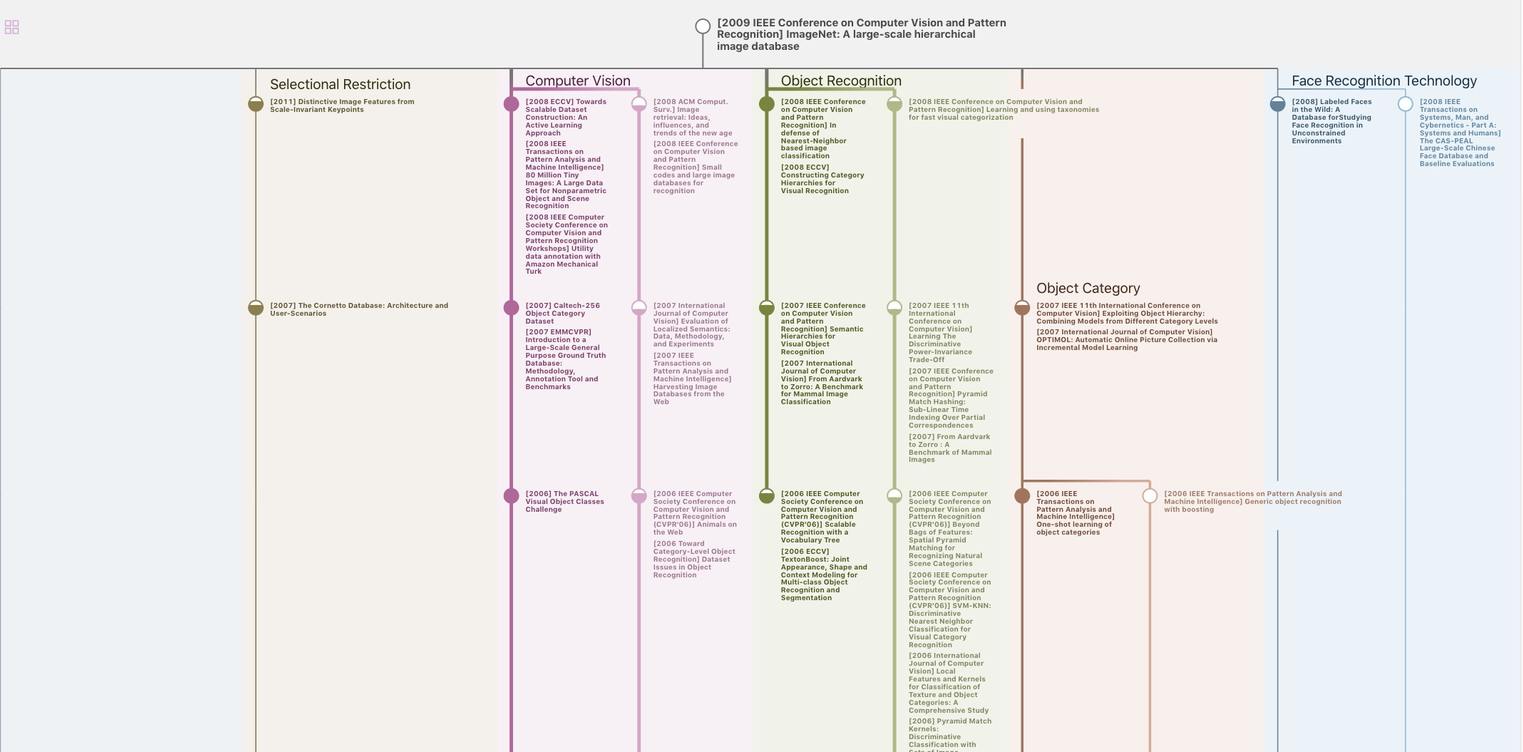
生成溯源树,研究论文发展脉络
Chat Paper
正在生成论文摘要