Twitter Sentimental Analysis using Machine Learning Approaches for SemeVal Dataset
2023 1st International Conference on Advanced Innovations in Smart Cities (ICAISC)(2023)
摘要
In recent years, Sentimental Analysis has enticed many researchers in this field. Due to the lack of suitable datasets, many scientists and researchers faced hindrances in their research. We used Semeval dataset because it’s the authentic dataset for computational sentimental Analysis. When it comes to natural catastrophes, political turmoil, and terrorism, social scientists and psychologists are interested in learning how individuals express their feelings and opinions. In this paper, we present the approach to the SemEva1-2017 dataset. As we know, with the advancement of technology, social media have established strong worldwide connectivity and information sharing. The wide use of social media and media-networking sites produced an unprecedented amount of data. Sharing information using these websites has become very common. To detect the triggering factors has become necessary to understand the behavioural and emotional state to avoid anti-social behaviour and extreme or impulsive responses. We reveal to identify the emotional textual data using different strategies. Classification of the Tweets according to the Sentimental Analysis has an important role in the social, economic, and political world s. The effective strategy for tackling and coping with it is to use computational techniques to identify the speech type. For feature extraction, we use a variety of machine learning classifiers. It’s crucial to detect related features in a text correctly. As a result, using and improving NLP approaches can aid in improved understanding and analysis of data. Briefly, we use an unsupervised. TF-IDF for the Feature Extraction to train the word Embedding Techniques that are tuned into transform training data and transformed Test data. The model is finally initialized using vectorization on Twitter sentiment analysis to train the latter. Then, transformed the model to create the transformed dataset. The major findings and outcomes of SemEva1-2017 on Identifying and Categorizing the Sentiments of the Language in social media of Twitter are presented and evaluated results based on applying different classifiers for Machine learning Modeling.
更多查看译文
关键词
TF-IDF,Sentimental Analysis,Vectorization,Word Embedding,NLP
AI 理解论文
溯源树
样例
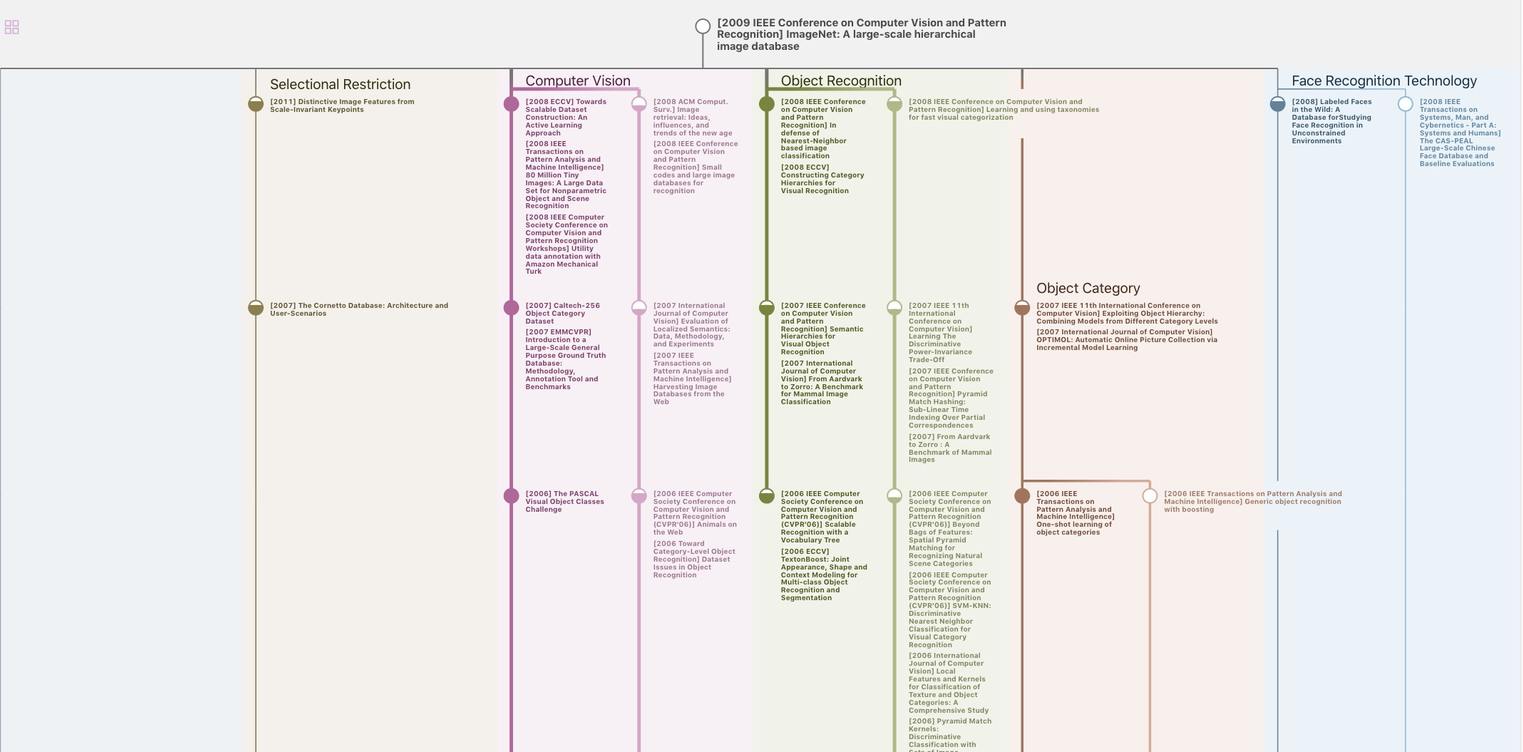
生成溯源树,研究论文发展脉络
Chat Paper
正在生成论文摘要