IEMT: Inequality-Based Metamorphic Testing for Autonomous Driving Models
2022 IEEE 22nd International Conference on Software Quality, Reliability, and Security Companion (QRS-C)(2022)
摘要
In the last ten years, the development of deep learning has promoted the progress of autonomous driving. Several major manufacturers, including Google, Tesla, Baidu, Audi, etc., are building and actively testing self-driving cars. However, the safety of autonomous driving still raises concerns. Recent research has used metamorphic testing to evaluate the robustness of autonomous driving models, but metamorphic relations defined during the test are basically based on equality, and there are very few inequality-based metamorphic relations. Our goal is to provide more inequality-based metamorphic relations to efficiently detect autonomous driving model violations. IEMT proposes additional inequality-based metamorphic relations and compares the robustness of autonomous driving models based on different neural network models. The experimental results show that the metamorphic relations we proposed can detect inconsistent behaviors of the driving model quite efficiently.
更多查看译文
关键词
Metamorphic testing,Deep neural networks,Autonomous driving model
AI 理解论文
溯源树
样例
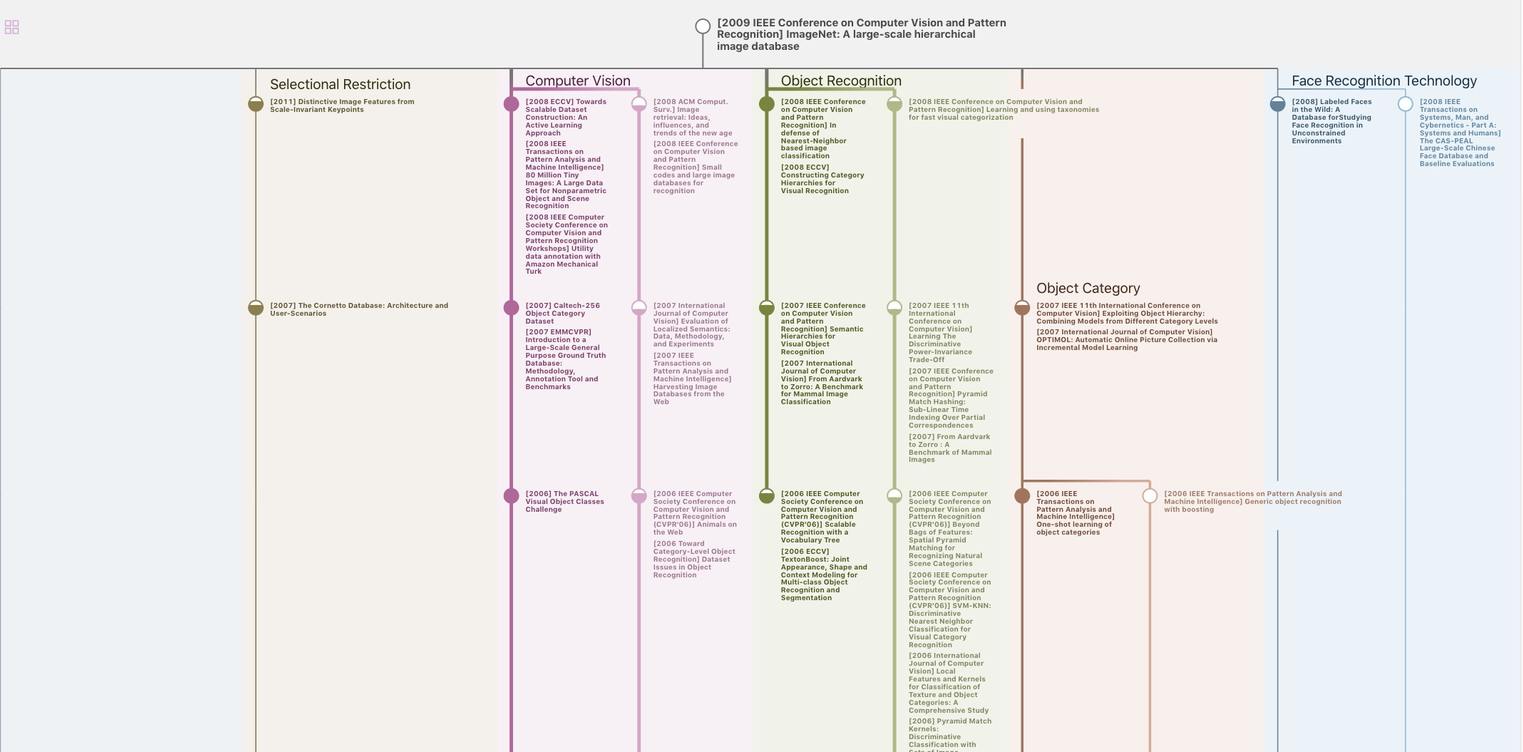
生成溯源树,研究论文发展脉络
Chat Paper
正在生成论文摘要