Driver’s Fatigue Classification based on Physiological Signals Using RNN-LSTM Technique
2022 IEEE-EMBS Conference on Biomedical Engineering and Sciences (IECBES)(2022)
摘要
One of the major reasons for road accidents is driver’s fatigue which causes several fatalities every year. Various studies on road accidents have proved that 20% of the accidents are caused mainly due to fatigue among drivers while driving. This paper presents the use of deep learning technique in classifying fatigue in drivers. By using deep neural networks, features are extracted automatically from preprocessed data of physiological signals such as electrocardiogram, heart rate, skin conductance response and body temperature. Public dataset HciLAB was used to train and validate the classification model. In this work, a comparative analysis of using Recurrent Neural Network - Long Short-term Memory (RNN-LSTM) deep learning architecture and the standard artificial neural network (ANN) was proposed and developed to classify fatigue based on the physiological features of the driver. The results revealed the superiority RNN-LSTM (98%) over standard ANN (80%), for driver fatigue classification. The proposed methods, based on RNN-LSTM deep learning architecture introduced elevated average accuracy in comparison with the standard artificial neural network.
更多查看译文
关键词
Using deep learning technique,the physiological signals could give better indication in fatigue detection among drivers.
AI 理解论文
溯源树
样例
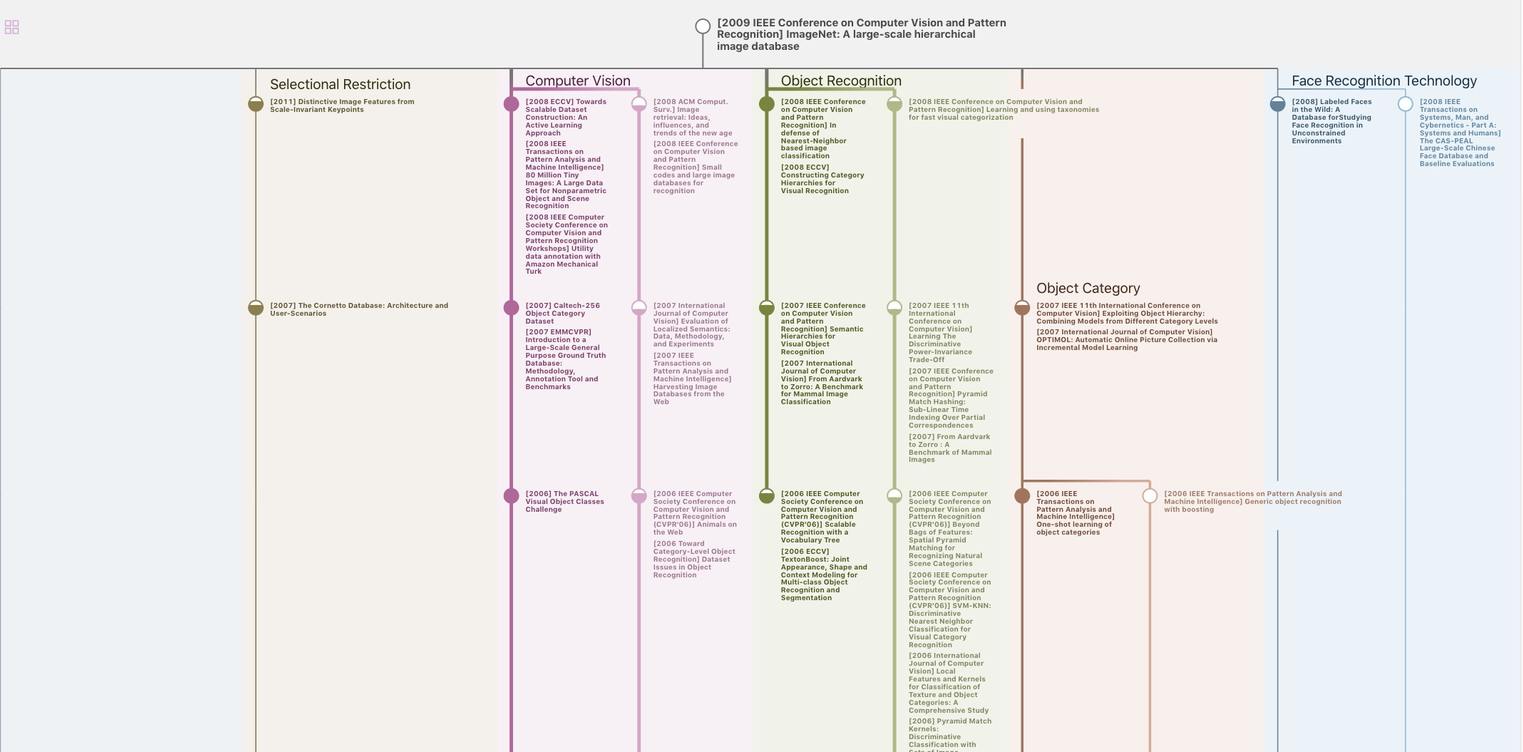
生成溯源树,研究论文发展脉络
Chat Paper
正在生成论文摘要