Feature Cloning and Feature Fusion Based Transportation Mode Detection Using Convolutional Neural Network
IEEE Transactions on Intelligent Transportation Systems(2023)
摘要
The smartphone-based sensors (including accelerometer, proximity, and gyroscope sensors) are ubiquitous and emerging mobility data sources that could be used for transportation modes (i.e. bus, train, car, walking, and stationary) detection. One of the important challenges in transportation modes detection is to build an appropriate model that can extract useful data from the sensor outputs and that can reduce misclassifications. Several factors make the feature modeling difficult including inappropriate sampling frequency of input signals, wavering behavior of devices (e.g. the changing orientation of a device relative to the human body), and continuous base vibration causing similar sensor outputs for both stationary and non-stationary states and related threshold values of velocity. This paper proposes novel approaches to address these challenges by developing a robust transportation mode detector based on a convolution neural network (CNN). The proposed robust detector develops a feature modeling technique by novel feature fusion and cloning techniques. Pre-trained features are constructed using a separate vanilla neural network (VNN) framework to extract the distinguishing components from the original features that are combined with the original and cloned features. The proposed feature fusion technique is successfully able to overcome the noise from the base vibration and the minimal informative outputs from the lower sampling frequency. This enables the CNN to be trained with more efficient and discriminative features that result in a better classification model. The proposed approaches have been validated using a large volume of mobile sensor data based on the movements of travelers. Different types of mobile sensors have been used to collect data including accelerometer, proximity, and gyroscope. Experimental results demonstrate that the proposed approaches can improve the performance of the detection engine significantly over conventional techniques and reduces the misclassification rate.
更多查看译文
关键词
Transportation mode,feature cloning,feature fusion,convolutional neural network,data augmentation
AI 理解论文
溯源树
样例
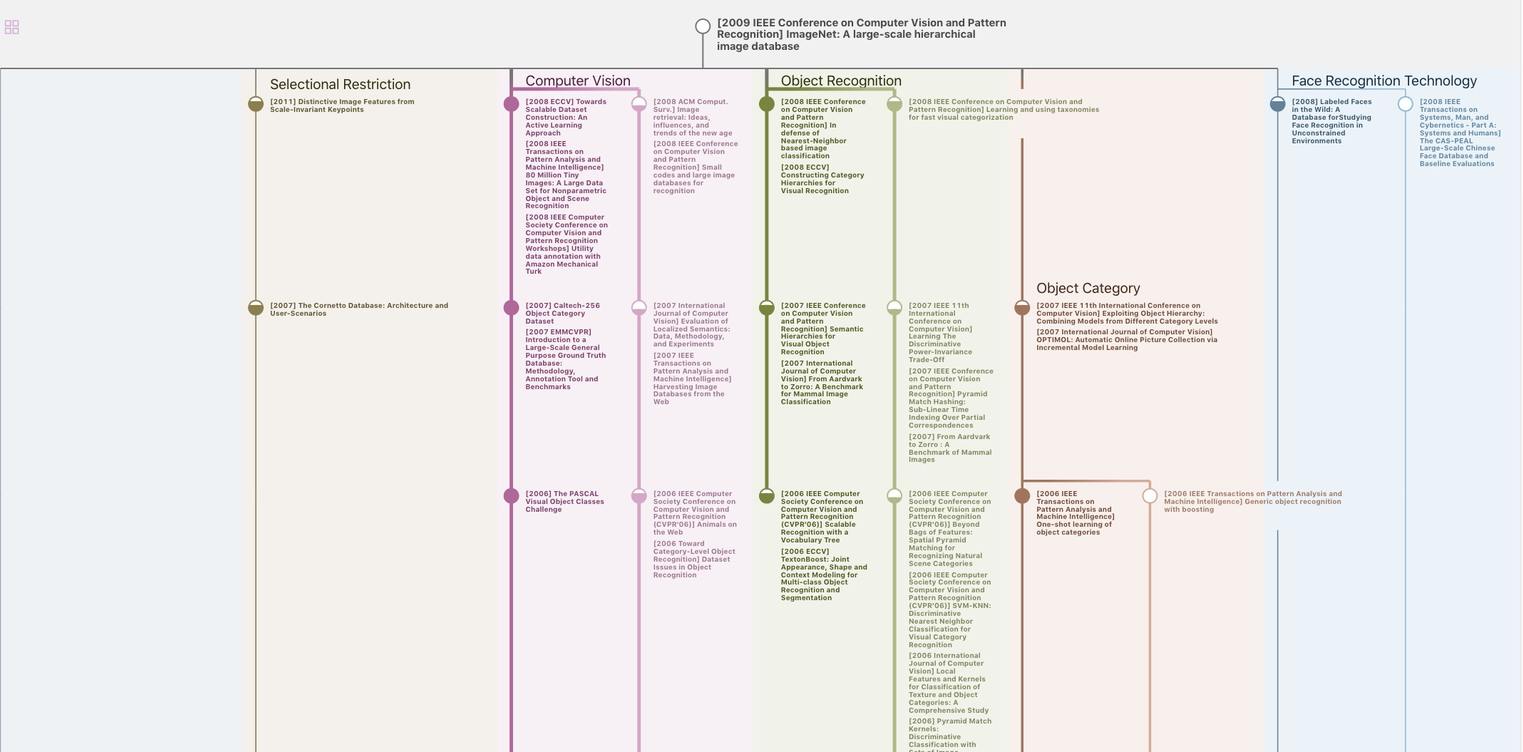
生成溯源树,研究论文发展脉络
Chat Paper
正在生成论文摘要