Interpretability of Hybrid Feature Using Graph Neural Networks from Mental Arithmetic Based EEG
2023 11th International Winter Conference on Brain-Computer Interface (BCI)(2023)
摘要
A high cognitive load could significantly impair problem-solving skills. Electroencephalogram (EEG)-based real-time assessment of mental workload is feasible, and graph neural networks (GNN) can classify brain activity patterns during cognitively demanding tasks with high accuracy. However, previous GNN studies pertaining to mental workload classification lack explainability. This study utilized a state-of-the-art GNN variant with GNNexplainer to find relevant connectivity during mental arithmetic (MA) tasks. In this endeavor, MA EEG recordings were retrieved from an openaccess database. The signals were transformed to graph data through the envelope correlation and power spectral density (PSD), and subjected to GNN with hierarchical graph pooling with a structure learning model to classify MA and baseline (BL). The model accuracy was $85.57 \pm 6.27$ and $96.26 \pm 4.14$% for the connectivity dataset and the PSD and the connectivity feature, respectively. Among the connections between nodes identified as important by GNNExplainer, two notable edge patterns were found as 1) from the left centro-parietal region to left frontal regions, and 2) the frontoparietal connection. The results indicate 1) the GNN model performance could be improved using the connectivity and PSD feature together, and 2) characteristic patterns of the connectome and PSD could be important for MA classification. The connectivity analysis by the ‘‘explainable’’ GNN model could be beneficial in future brain activity pattern studies.
更多查看译文
关键词
brain computer interface,mental arithmetic task,electroencephalography,conncetivity,graph neural networks,explainable artificial intelligence
AI 理解论文
溯源树
样例
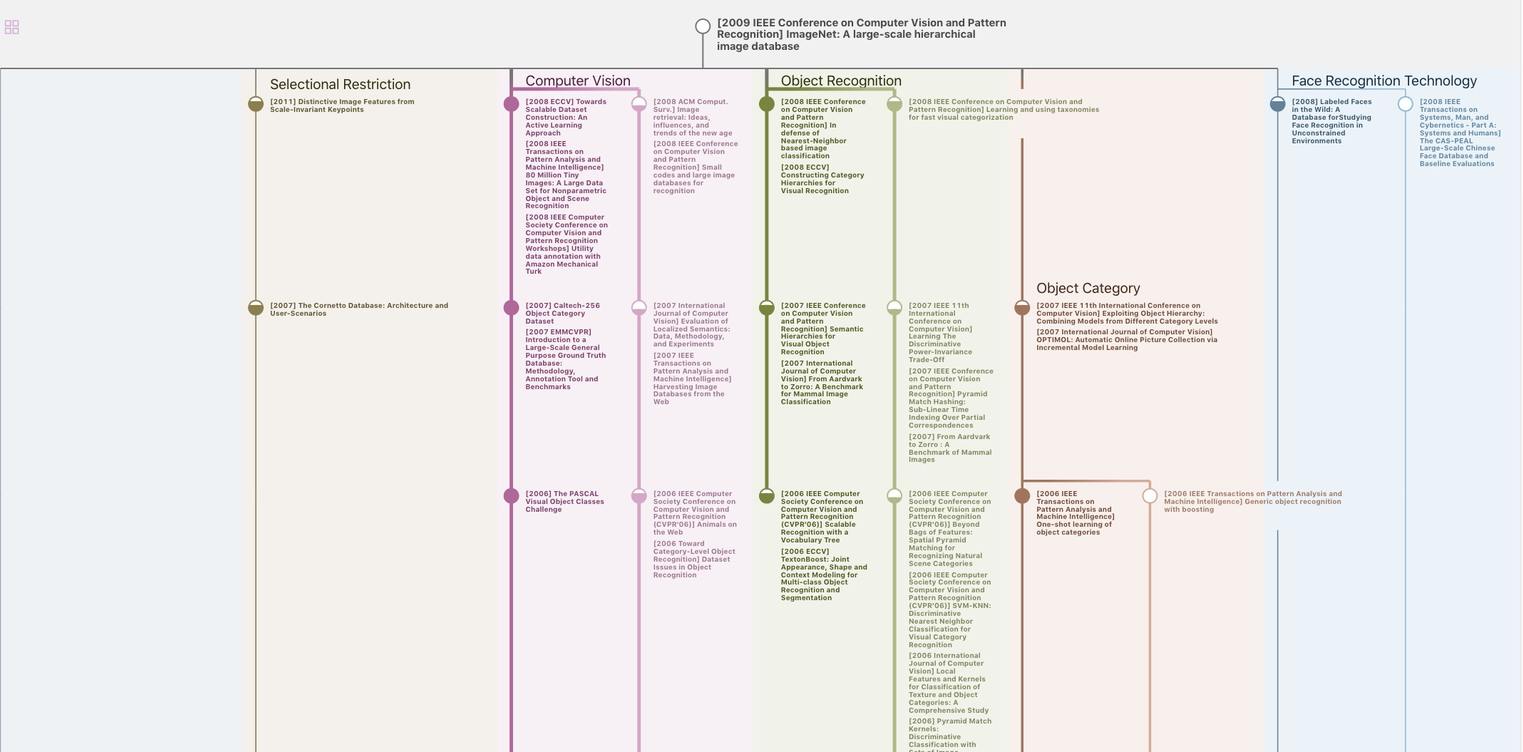
生成溯源树,研究论文发展脉络
Chat Paper
正在生成论文摘要