Distance-aware Multi-Agent Reinforcement Learning for Task Offloading in MEC Network.
2022 IEEE 24th Int Conf on High Performance Computing & Communications; 8th Int Conf on Data Science & Systems; 20th Int Conf on Smart City; 8th Int Conf on Dependability in Sensor, Cloud & Big Data Systems & Application (HPCC/DSS/SmartCity/DependSys)(2022)
摘要
Mobile Edge Computing (MEC) offers hugeous computing power and transmission capacity to allow various mobile terminal devices to offload their computation-intensive and delay-sensitive tasks to the nearby edge server. In this paper, we formulate the task offloading problem of multi-user and multi-server environment into multi-agent paradigm by describing each terminal device as an agent/ user. Multi-Agent Reinforcement Learning (MARL) is more applicable and effective to solve this problem in decentralized way other than in centralized way like single-agent methods. We propose a task offloading strategy named Distance-aware Multi-Agent Deep Deterministic Policy Gradient (DA-MA) and our system consists of mobile terminals, MEC servers, and various task sequences generated from different task arrival patterns. The optimization objective is to minimize the task completion delay under the condition of occupying transmission uplink exclusively to the same destination server. Each agent's partial observation includes its task features, offloading link's access state, the computational capacity of servers, and especially the distance to each MEC server. Finally, extensive experimental results show that our DA-MA algorithm has good convergence and it can achieve the better performance compared to other baselines. Furthermore, we depict cooperation among agents and among task priorities within whole offloading process in detail.
更多查看译文
关键词
task offloading,mobile edge computing,multiagent reinforcement learning,artificial intelligence
AI 理解论文
溯源树
样例
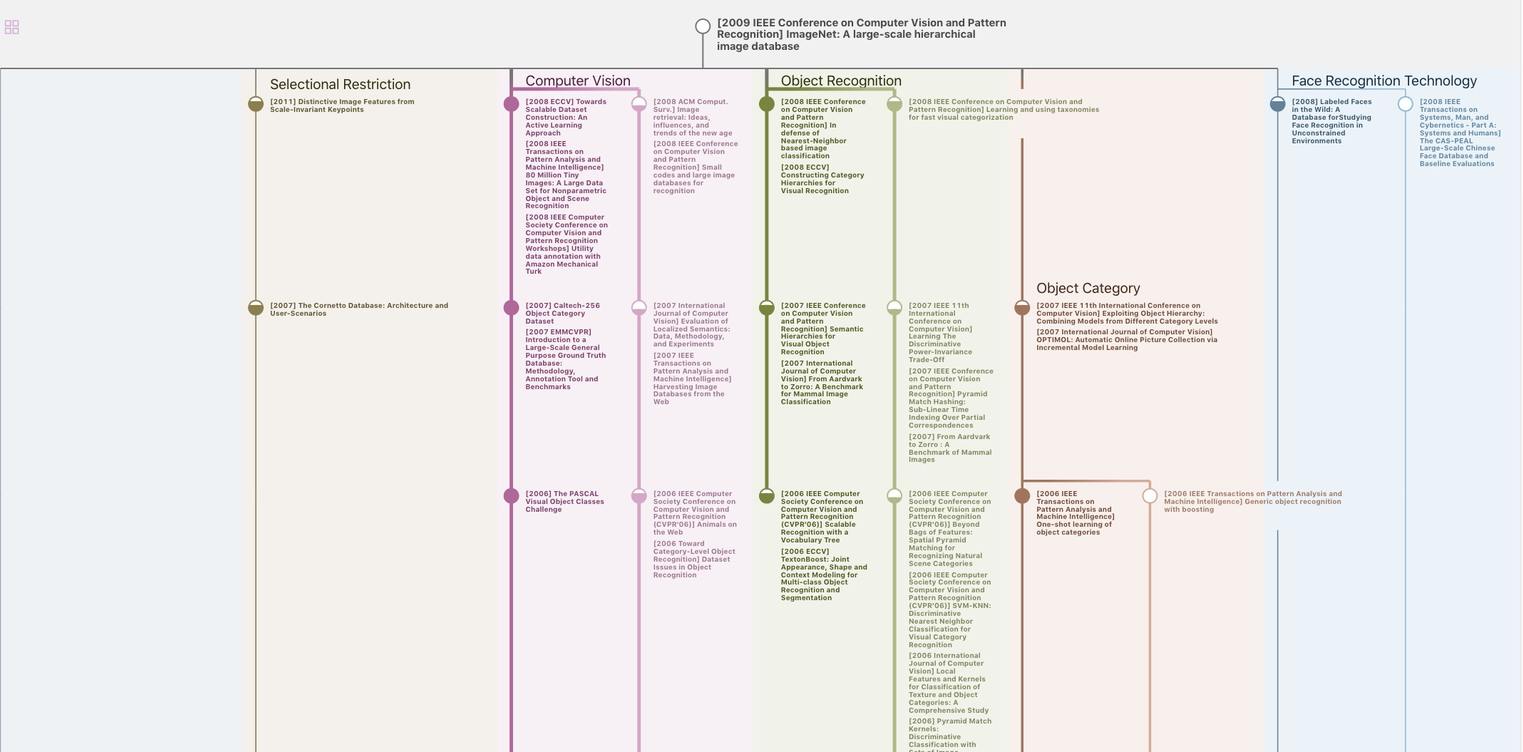
生成溯源树,研究论文发展脉络
Chat Paper
正在生成论文摘要