Group $\mathrm{L}_{1/2}$ Regularization for Filter Pruning of Convolutional Neural Networks
2022 4th International Conference on Frontiers Technology of Information and Computer (ICFTIC)(2022)
摘要
Group regularization method has been widely used for structured pruning of convolutional neural networks (CNNs). While the group lasso (GL) regularization can impose group level sparsity on redundant filters, it cannot impose strong sparsity on the redundant weights in the retained filters. This leads to the low weight sparsity of the retained filters and the inability to prune the retained filters with few redundant nonzero weights. In this paper, the group
$\mathbf{L}_{\mathbf{1}/\mathbf{2}}$
regularization
$(\mathbf{GL}_{\mathbf{1}/\mathbf{2}})$
is introduced into the loss function for pruning not only the redundant filters but also the redundant weights of the retained filters. The numerical experiments show that
$\mathbf{GL}_{\mathbf{1}/\mathbf{2}}$
can obtain better pruning performance with comparable to even better accuracy performance than GL. In addition, the empirical analysis in terms of regularization loss shows that
$\mathbf{GL}_{\mathbf{1}/\mathbf{2}}$
possesses more powerful punishment ability than GL.
更多查看译文
关键词
Convolutional neural networks,Group regularization,Group Lasso,Filter pruning
AI 理解论文
溯源树
样例
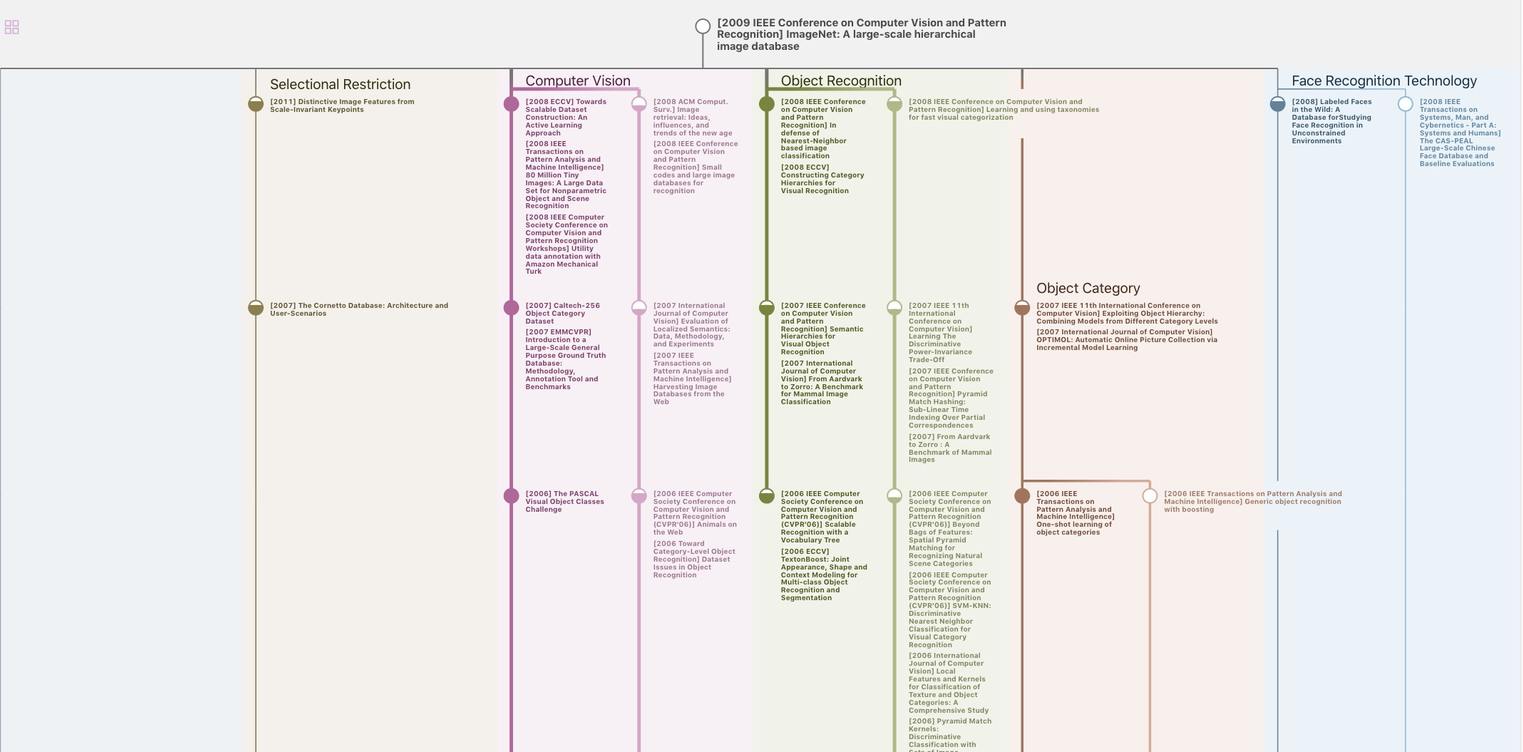
生成溯源树,研究论文发展脉络
Chat Paper
正在生成论文摘要