Heterogeneous Federated Learning for Balancing Job Completion Time and Model Accuracy
2022 IEEE 28th International Conference on Parallel and Distributed Systems (ICPADS)(2023)
摘要
Federated Learning (FL) is a secure distributed learning paradigm, which enables potentially a large number of devices to collaboratively train a global model based on their local dataset. FL exhibits two distinctive features in job requirement and client participation, where FL jobs may have different training criteria, and clients possess diverse device capabilities and data characteristics. In order to capture such heterogeneities, this paper proposes a new FL framework, Hca, which aims to strike a balance between the job completion time and model accuracy. Specifically, Hca builds upon a number of innovations in the following three phases: i) pre-estimation: we first derive the optimal set of parameters used in training in terms of the number of training rounds, the number of iterations and the number of participating clients in each round; ii) client selection: we design a novel device selection algorithm, which selects the most effective clients for participation based on both client historical contributions and data effectiveness; iii) model aggregation: we improve the classic FedAvg algorithm by integrating the model loss reduction in consecutive rounds as a weighted factor into aggregation computation. To evaluate the performance and effectiveness of Hca, we conduct theoretical analysis and testbed experiments over an FL platform FAVOR. Extensive results show that Hca can improve the job completion time by up to 34% and the model accuracy by up to 9.1%, and can reduce the number of communication rounds required in FL by up to 75% compared with two state-of-the-art FL frameworks.
更多查看译文
关键词
Federated Learning,Client Selection,Time and Accuracy Balancing
AI 理解论文
溯源树
样例
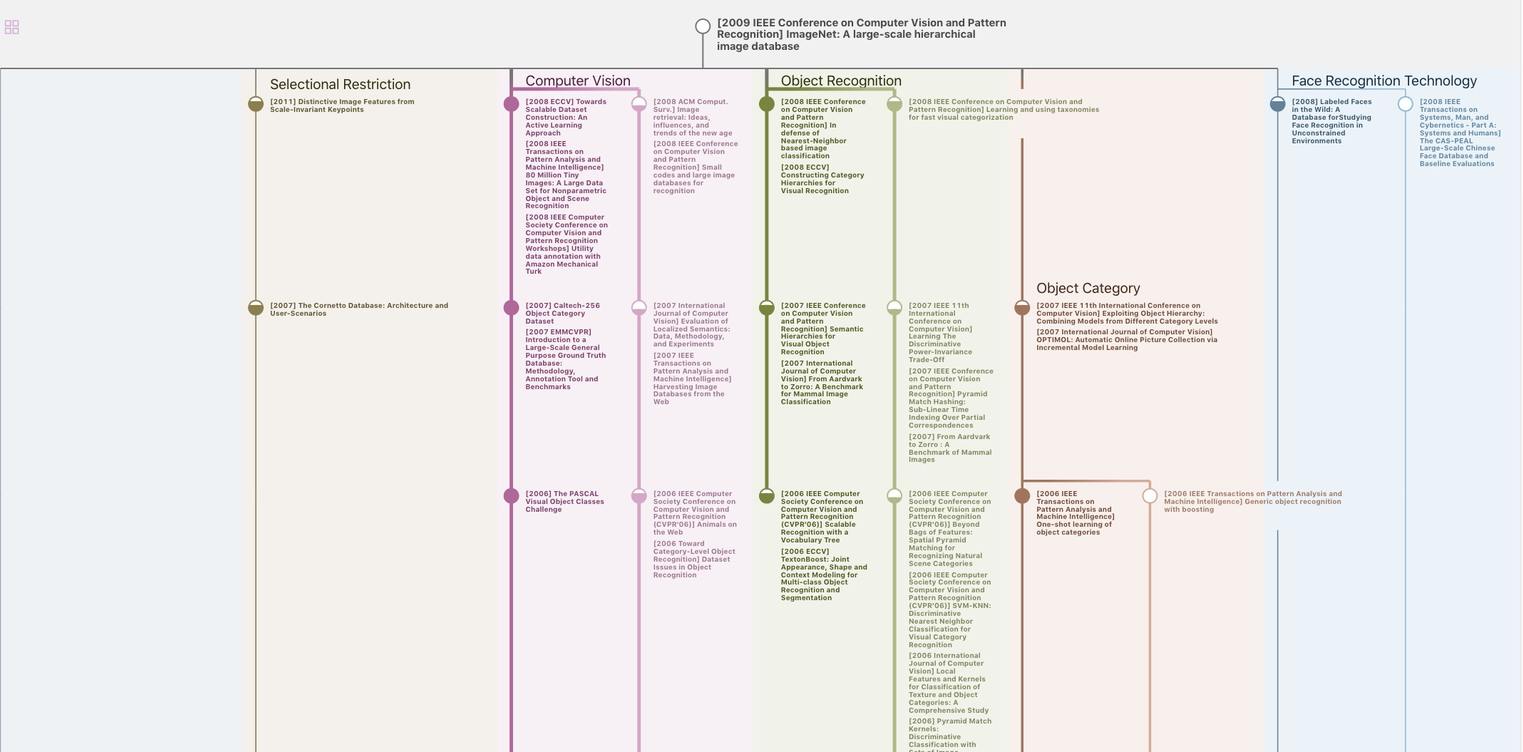
生成溯源树,研究论文发展脉络
Chat Paper
正在生成论文摘要