2D LiDAR Based Reinforcement Learning for Multi-Target Path Planning in Unknown Environment
IEEE Access(2023)
摘要
Global path planning techniques have been widely employed in solving path planning problems, however they have been found to be unsuitable for unknown environments. Contrarily, the traditional Q-learning method, which is a common reinforcement learning approach for local path planning, is unable to complete the task for multiple targets. To address these limitations, this paper proposes a modified Q-learning method, called Vector Field Histogram based Q-learning (VFH-QL) utilized the VFH information in state space representation and reward function, based on a 2D LiDAR sensor. We compared the performance of our proposed method with the classical Q-learning method (CQL) through training experiments that were conducted in a simulated environment with a size of 400 square pixels, representing a 20-meter square map. The environment contained static obstacles and a single mobile robot. Two experiments were conducted: experiment A involved path planning for a single target, while experiment B involved path planning for multiple targets. The results of experiment A showed that VFH-QL method had 87.06% less training time and 99.98% better obstacle avoidance compared to CQL. In experiment B, VFH-QL method was found to have an average training time that was 95.69% less than that of the CQL method and 83.99% better path quality. The VFH-QL method was then evaluated using a benchmark dataset. The results indicated that the VFH-QL exhibited superior path quality, with efficiency of 94.89% and improvements of 96.91% and 96.69% over CQL and SARSA in the task of path planning for multiple targets in unknown environments.
更多查看译文
关键词
RL,path planning,Q-learning,mobile robot
AI 理解论文
溯源树
样例
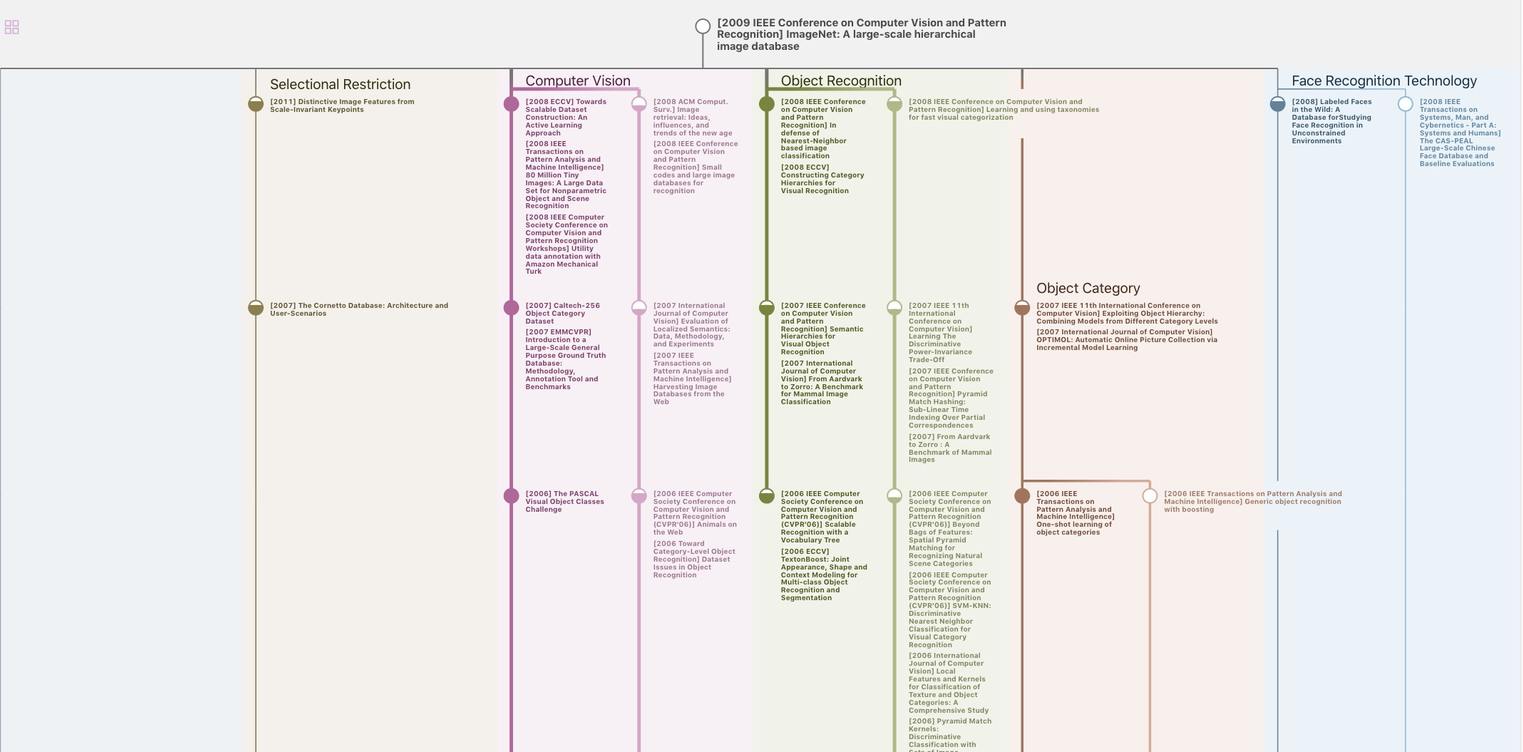
生成溯源树,研究论文发展脉络
Chat Paper
正在生成论文摘要