Sensitivity Adaptation of Lower-Limb Exoskeleton for Human Performance Augmentation Based on Deep Reinforcement Learning
IEEE Access(2023)
摘要
The lower-limb exoskeleton for human performance augmentation (LEHPA) in sensitivity amplification control (SAC) is vulnerable to model parameter uncertainties and unmodeled dynamics due to its large sensitivity to external disturbances resulting from the positive feedback by the inverse dynamic model of the exoskeleton. This paper firstly proposes to combine SAC with deep reinforcement learning (DRL) to reduce the dependence on the model accuracy and tackle the ever-changing human-exoskeleton interaction (HEI) dynamics. The sensitivity adjustment is interpreted as finding the optimal policy for a Markov Decision Process (MDP) and solved using deep reinforcement learning algorithms. To train the policy safely and efficiently, a multibody simulation environment is created to implement the training process, accompanied by a novel hybrid inverse-forward dynamics simulation method to carry out the simulation. For comparison purposes, the SAC controller is introduced as a benchmark. A novel performance evaluation method based on the HEI forces at the back, thighs, and shanks is proposed to evaluate the control effect of the trained SADRL controller quantitatively. The SADRL controller is compared with the SAC controller at five specified walking speeds, resulting in a lumped HEI force ratio as low as 0.54. The total decrease of HEI forces demonstrates the superior control effect of the SADRL strategy.
更多查看译文
关键词
Lower-limb exoskeleton for human performance augmentation,sensitivity amplification control,sensitivity adaptation,deep reinforcement learning
AI 理解论文
溯源树
样例
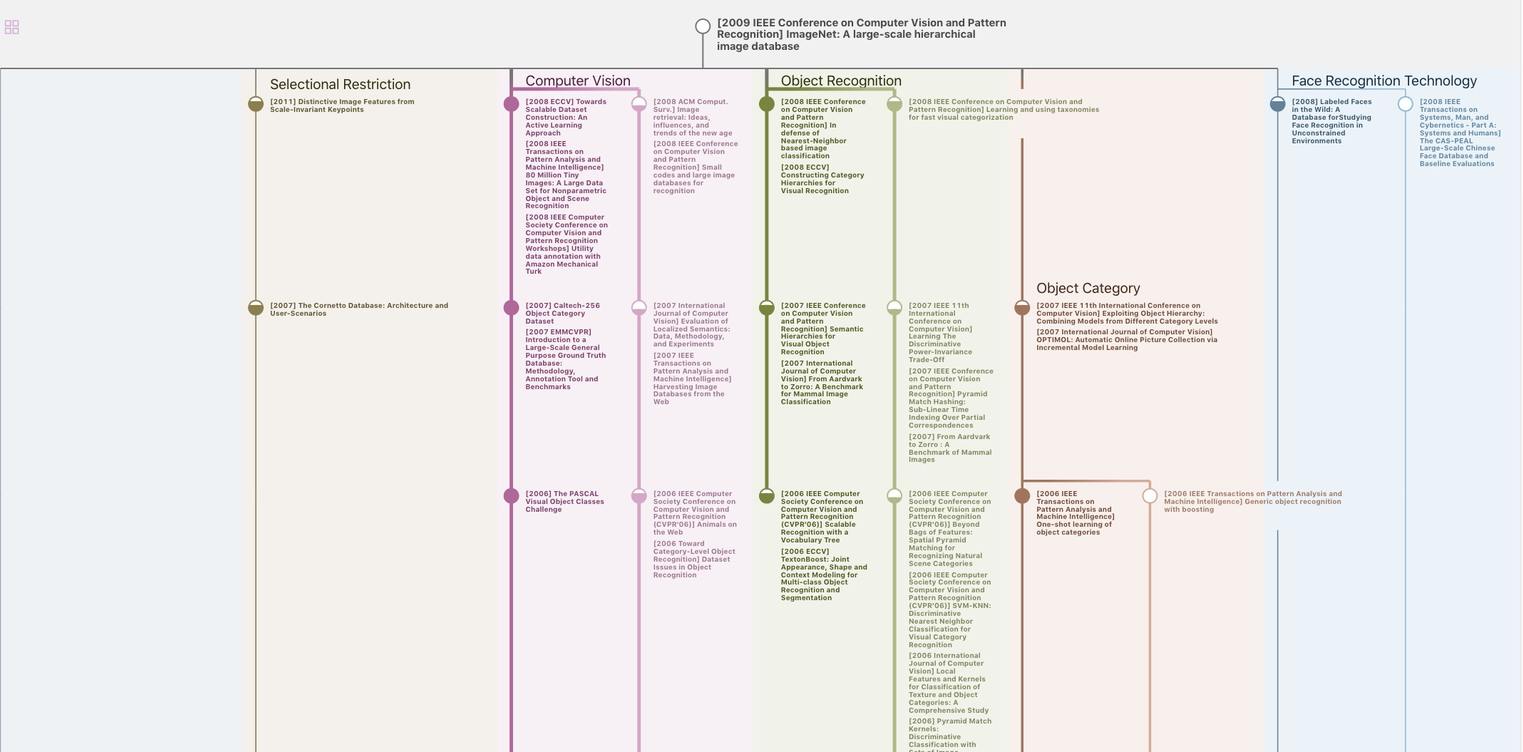
生成溯源树,研究论文发展脉络
Chat Paper
正在生成论文摘要