Bearing Fault Diagnosis Using Residual Network and Attention Mechanism
2022 IEEE 8th International Conference on Computer and Communications (ICCC)(2022)
摘要
Accurate fault diagnosis is the key to ensure the safe and operation of rotating machines. Accuracy of bearing fault diagnosis will be reduced in complex noise environments and variable load conditions. Therefore, this paper proposes a fault diagnosis method based on attention mechanism and residual neural network (FaultCNN). Firstly, a residual attention module is designed to extract the original fault features of the noise signals. Secondly, the ability to extract features of the residual module is further enhanced by adding an average pooling layer to the residual structure shortcut. To validate the diagnostic performance of the model, CWRU datasets are used for experimental verification. In the noise environment of
$\text{SNR}=-6 \text{dB}$
, the accuracy of model fault diagnosis is 94.23%. Compared to existing bearing fault diagnostic methods, FaultCNN has higher anti-noise performance and variable load adaptability.
更多查看译文
关键词
fault diagnosis,deep learning,residual neural network,attention mechanism,anti-noise
AI 理解论文
溯源树
样例
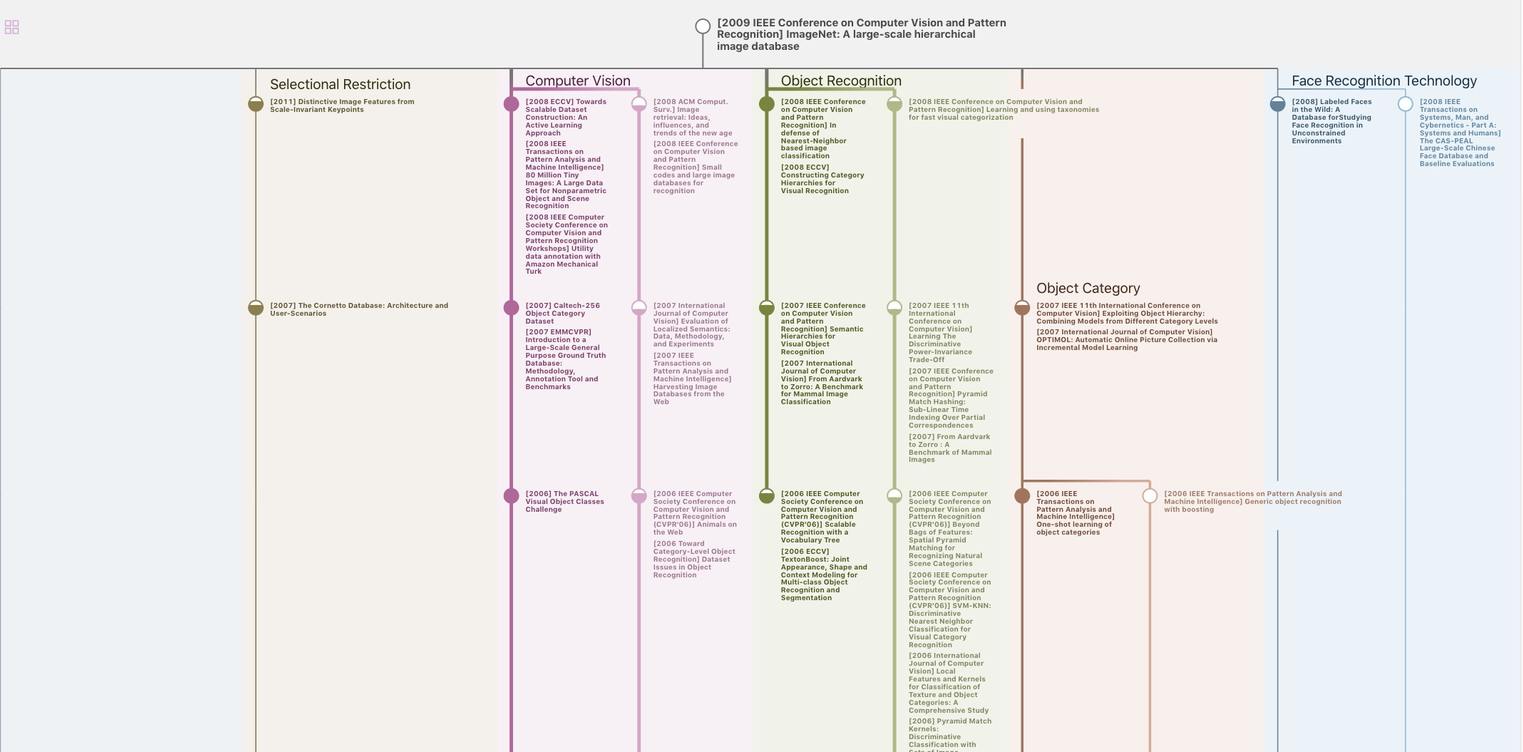
生成溯源树,研究论文发展脉络
Chat Paper
正在生成论文摘要