Mapping Canopy Height from ICESat-2 and Landsat-9 using Machine Learning in the Himalayan Corbett Tiger Reserve, India
2023 International Conference on Machine Intelligence for GeoAnalytics and Remote Sensing (MIGARS)(2023)
摘要
Canopy height (CH) is an important parameter for better managing forests, biomass assessment and biodiversity conservation. The study’s goal is the spatial mapping of CH by combining ICESat-2 and optical data information from Landsat-9 and Sentine1-2 using a support vector machine (SVM) and random forest (RF). Further, the most to least important predictors were identified for CH prediction. This assessment was performed in the Corbett Tiger reserve (CTR), Himalayan Uttrakhand state of India. The result showed that the mean CH in the CTR is 32.61 m. Root mean square error (RMSE) (5.339 m and 5.456 m), mean absolute error (MAE) (4.048 m and 4.166 m), and R-squared (R2) (0.552 and 0.531) were the optimal training values for SVM and RF, respectively. Models testing between observed and predicted CH showed the RMSE is 5.42 m and 5.53 m, MAE is 4.10 m and 4.20, and R2 is 0.55 and 0.53 for SVM and RF, respectively. Canopy profiles and metrics at percentiles height (PH) are dominant predictors. Landsat-8 derived vegetative indices (VI’s) have moderate importance. Such an integrated approach is helpful in managing CTR and CH mapping of other protected forests.
更多查看译文
关键词
canopy height,random forest,support vector machine,LiDAR,ICESat-2
AI 理解论文
溯源树
样例
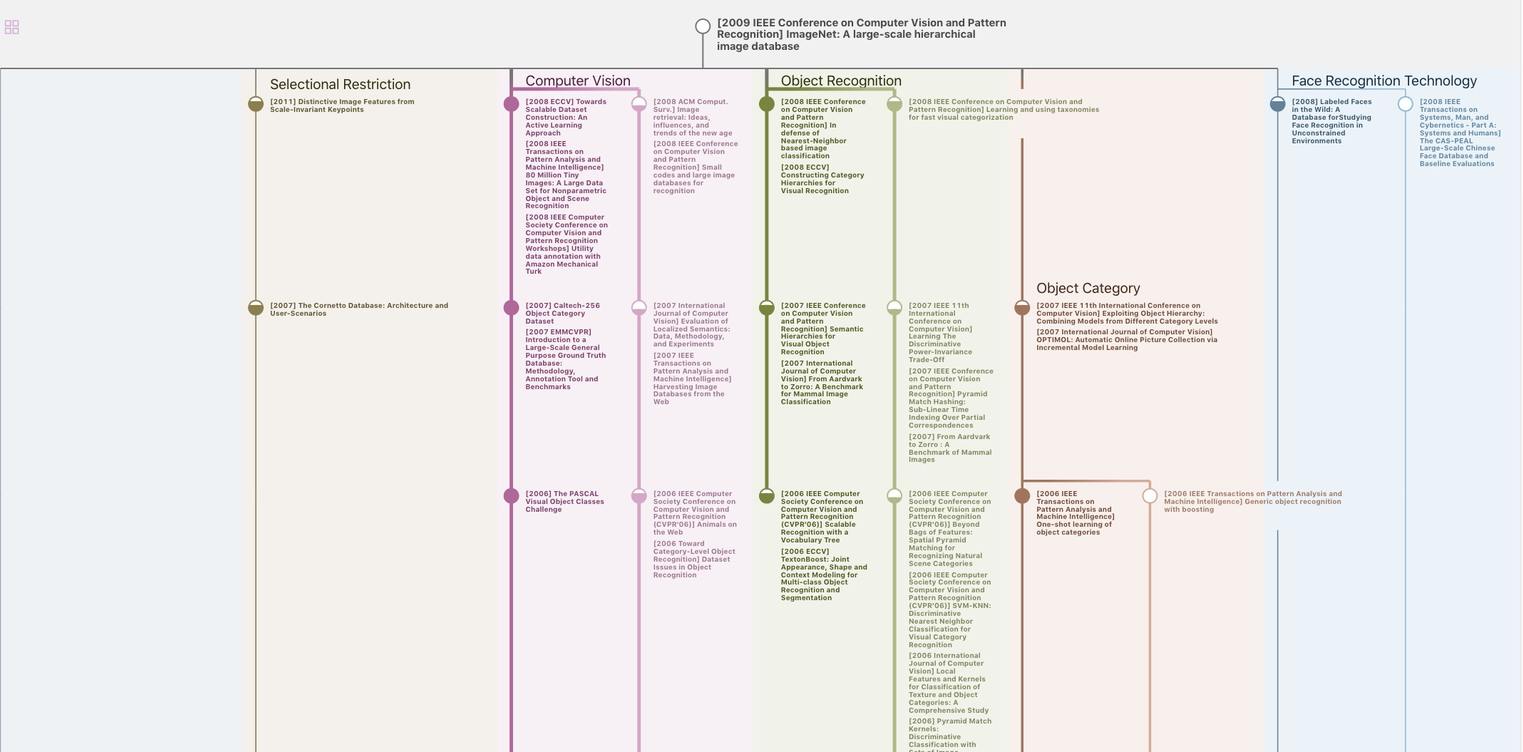
生成溯源树,研究论文发展脉络
Chat Paper
正在生成论文摘要