An accurate approach of device-free localization with attention empowered residual network
Applied Soft Computing(2023)
摘要
Device-free localization (DFL) has been recognized as an emerging technology in the Internet of Things (IoT) field. Based on soft computing techniques, e.g., cloud systems, neural networks, wireless sensor networks, etc., DFL can locate the targets that do not carry any equipment. A crucial problem for the DFL is how to extract significant patterns from weak signal variations in order to achieve high accuracy and robustness. To solve this issue, we transform the DFL procedure into a classification task. In this paper, a channel-dependent attention empowered residual network (CA-ResNet) with three residual layers is designed for precise localization by extracting underlying signal features. The CA-ResNet takes the advantages of the residual connection and the attention mechanism in feature extraction, which is expected to have a high level of robustness in the face of extreme noise and abnormal circumstances for DFL. Experiment results based on real-world data demonstrate that our proposed approach provides a high level of localization performance for a suitable grid size. For instance, the CA-ResNet approach achieves high localization accuracy of almost 100% in general cases. Under the condition of introducing noise to data, e.g., a signal to noise ratio greater than -5 dB, our proposed method maintains high accuracy and strong robustness. Compared with the state-of-the-art DFL methods in several cases, the best localization accuracy obtained by our proposed method is 16.4% better than the compared AugRF, 15.5% better than the radio robust image fingerprint indoor localization algorithm (RRIFLoc), and 6.9% better than the convolutional autoencoder neural network, especially in challenging cases that partial sensor nodes are broken or power off under noisy condition.(c) 2023 Published by Elsevier B.V.
更多查看译文
关键词
Device -free localization,Residual network,Attention mechanism,Deep learning
AI 理解论文
溯源树
样例
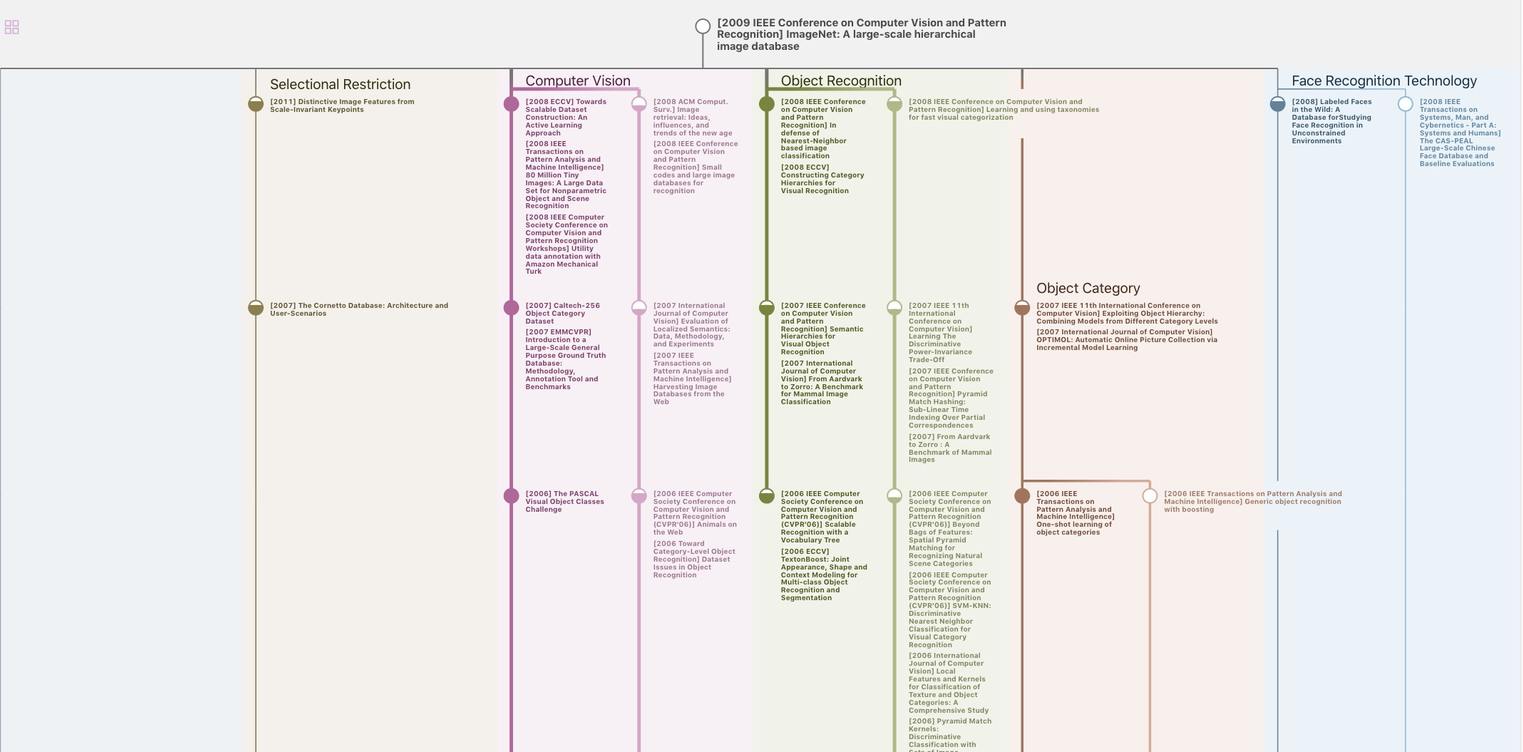
生成溯源树,研究论文发展脉络
Chat Paper
正在生成论文摘要