Identifying Hepatocellular Carcinoma from imaging reports using natural language processing to facilitate data extraction from electronic patient records
medRxiv (Cold Spring Harbor Laboratory)(2022)
摘要
Background The National Institute for Health Research Health Informatics Collaborative (NIHR HIC) viral hepatitis theme is working to overcome governance and data challenges to collate routine clinical data from electronic patients records from multiple UK hospital sites for translational research. The development of hepatocellular carcinoma (HCC) is a critical outcome for patients with viral hepatitis with the drivers of cancer transformation poorly understood.
Objective This study aims to develop a natural language processing (NLP) algorithm for automatic HCC identification from imaging reports to facilitate studies into HCC.
Methods 1140 imaging reports were retrieved from the NIHR HIC viral hepatitis research database v1.0. These reports were from two sites, one used for method development (site 1) and the other for validation (site 2). Reports were initially manually annotated as binary classes (HCC vs. non-HCC). We designed inference rules for recognising HCC presence, wherein medical terms for eligibility criteria of HCC were determined by domain experts. A rule-based NLP algorithm with five submodules (regular expressions of medical terms, terms recognition, negation detection, sentence tagging, and report label generation) was developed and iteratively tuned.
Results Our rule-based algorithm achieves an accuracy of 99.85% (sensitivity: 90%, specificity: 100%) for identifying HCC on the development set and 99.59% (sensitivity: 100%, specificity: 99.58%) on the validation set. This method outperforms several off-the-shelf models on HCC identification including “machine learning based” and “deep learning based” text classifiers in achieving significantly higher sensitivity.
Conclusion Our rule-based NLP method gives high sensitivity and high specificity for HCC identification, even from imbalanced datasets with a small number positive cases, and can be used to rapidly screen imaging reports, at large-scale to facilitate epidemiological and clinical studies into HCC.
Problem Establishing a cohort of hepatocellular carcinoma (HCC) from imaging reports via manual review requires advanced clinical knowledge and is costly, time consuming, impractical when performed on a large scale.
What is Already Known Although some studies have applied natural language processing (NLP) techniques to facilitate identifying HCC information from narrative medical data, the proposed methods based on a pre-selection by diagnosis codes, or subject to certain standard templates, have limitations in application.
What This Paper Adds We have developed a hierarchical rule-based NLP method for automatic identification of HCC that uses diagnostic concepts and tumour feature representations that suggest an HCC diagnosis to form reference rules, accounts for differing linguistic styles within reports, and embeds a data pre-processing module that can be configured and customised for different reporting formats. In doing so we have overcome major challenges including the analysis of imbalanced data (inherent in clinical records) and lack of existing unified reporting standards.
### Competing Interest Statement
GC reports personal fees from Gilead and Merck Sharp & Dohme outside the submitted work. EB and PCM have academic collaborative partnerships with GSK. Other authors have no conflict of interest.
### Funding Statement
This research has been conducted using National Institute for Health Research (NIHR) Health Informatics Collaborative (HIC) data resources and funded by the NIHR HIC, and has been supported by NIHR Biomedical Research Centres at Oxford and Imperial. EB is an NIHR senior investigator. GSC is supported in part by the Imperial NIHR Biomedical Research Centre and NIHR Research Professorship. PCM is funded by the Wellcome Trust (ref. 110110/Z/15/Z), the Francis Crick Institute, and UCL NIHR BRC. CC is a doctoral student who receives partial doctoral funding from GlaxoSmithKline. The views expressed in this article are those of the authors and not necessarily those of the National Health Service, the NIHR, or the Department of Health.
### Author Declarations
I confirm all relevant ethical guidelines have been followed, and any necessary IRB and/or ethics committee approvals have been obtained.
Yes
The details of the IRB/oversight body that provided approval or exemption for the research described are given below:
Ethics approval and consent to participate: The research database for the NIHR HIC viral hepatitis theme was approved by South Central - Oxford C Research Ethics Committee (REF Number: 15/SC/0523). All methods in this study were carried out in according to relevant guidelines and regulations. The requirement for written informed consent was waived by South Central - Oxford C Research Ethics Committee, because data have been anonymised before its use and the study is retrospective.
I confirm that all necessary patient/participant consent has been obtained and the appropriate institutional forms have been archived, and that any patient/participant/sample identifiers included were not known to anyone (e.g., hospital staff, patients or participants themselves) outside the research group so cannot be used to identify individuals.
Yes
I understand that all clinical trials and any other prospective interventional studies must be registered with an ICMJE-approved registry, such as ClinicalTrials.gov. I confirm that any such study reported in the manuscript has been registered and the trial registration ID is provided (note: if posting a prospective study registered retrospectively, please provide a statement in the trial ID field explaining why the study was not registered in advance).
Yes
I have followed all appropriate research reporting guidelines and uploaded the relevant EQUATOR Network research reporting checklist(s) and other pertinent material as supplementary files, if applicable.
Yes
Data from NIHR HIC viral hepatitis theme may be made available to researchers on request following positive review by the steering committee. Further details are available at https://hic.nihr.ac.uk. Queries regarding data access should be directed to orh-tr.nihrhic@nhs.net. MIMIC is provided through the work of researcher at the MIT Laboratory for Computational Physiology and the collaborators. Data are available through formally requesting access with the steps here: https://mimic.mit.edu/docs/gettingstarted/.
* AASLD
: American Association for the Study of Liver Diseases
ACR
: American College of Radiology
AFP
: Alpha fetoprotein
APASL
: Asian Pacific Association for the Study of the Liver
BERT
: bidirectional encoder representations from transformers
BOW
: bag-of-words
CNN
: convolutional neural network
CT
: multiphasic computed tomography
DL
: deep learning
EASL
: European Association for the Study of the Liver
EHR
: electronic health record
ESMO
: European Society for Medical Oncology
FP
: false positive
FN
: false negative
HAN
: hierarchical attention networks
HBV
: hepatitis B virus
HCC
: hepatocellular carcinoma
HCV
: hepatitis C virus
HIC
: Health Informatics Collaborative
ICHT
: Imperial College Healthcare NHS Trust
LI-RADS
: liver imaging reporting and data system
LR
: logistic regression
MRI
: magnetic resonance imaging
NB
: Naive Bayes
NHS
: National Health Service
NIHR
: National Institute for Health Research
NLP
: natural language processing
NLTK
: Natural Language Toolkit
OUH
: Oxford University Hospitals
SVM
: support vector machine
TF-IDF
: term frequency inverse document frequency
TP
: true positive
TN
: true negative.
更多查看译文
关键词
natural language processing,data extraction,hepatocellular carcinoma,electronic patient records
AI 理解论文
溯源树
样例
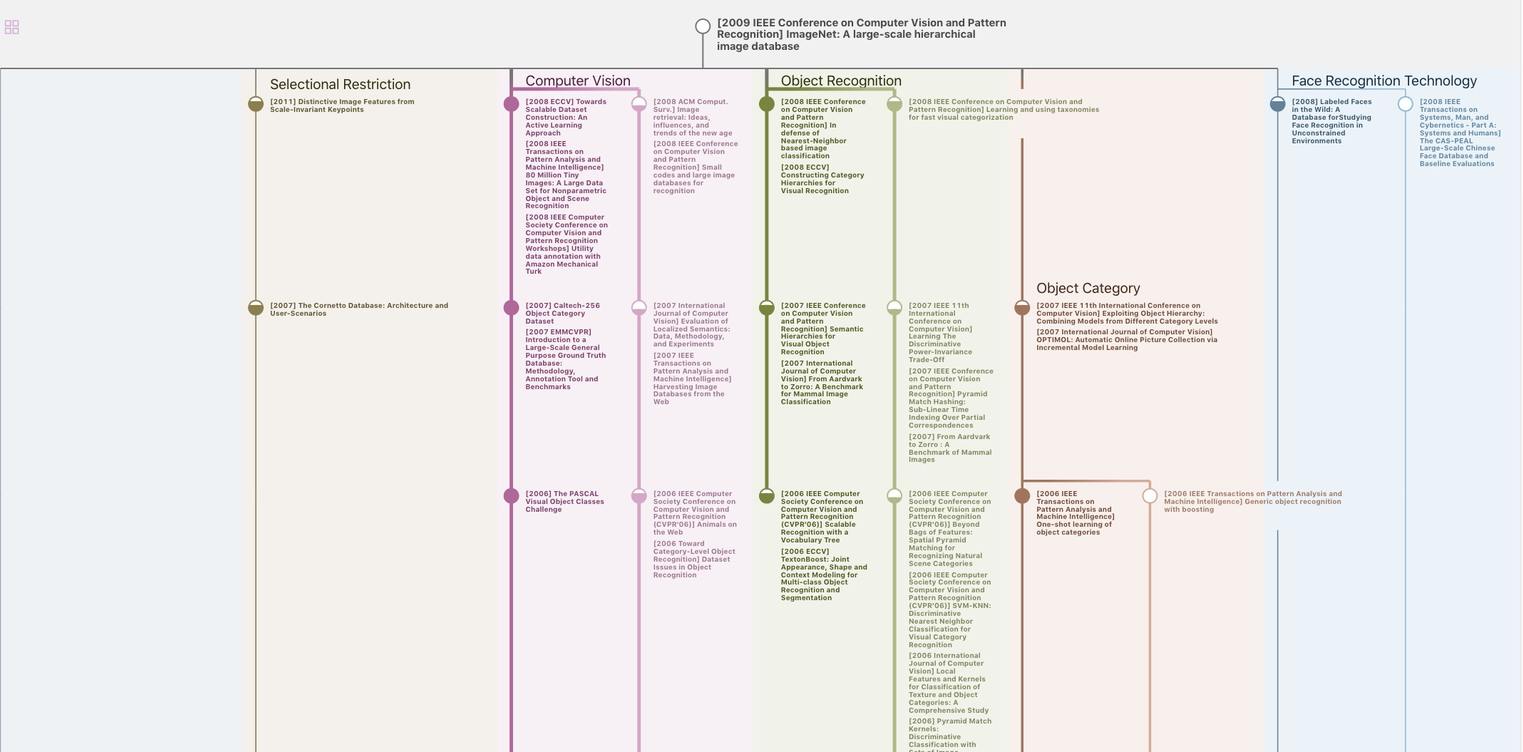
生成溯源树,研究论文发展脉络
Chat Paper
正在生成论文摘要