Estimating treatment effects for time-to-treatment antibiotic stewardship in sepsis
Nature Machine Intelligence(2023)
摘要
Sepsis is a life-threatening condition with a high in-hospital mortality rate. The timing of antibiotic administration poses a critical problem for sepsis management. Existing work studying antibiotic timing either ignores the temporality of the observational data or the heterogeneity of the treatment effects. Here we propose a novel method (called T4) to estimate treatment effects for time-to-treatment antibiotic stewardship in sepsis. T4 estimates individual treatment effects by recurrently encoding temporal and static variables as potential confounders, and then decoding the outcomes under different treatment sequences. We propose mini-batch balancing matching that mimics the randomized controlled trial process to adjust the confounding. The model achieves interpretability through a global-level attention mechanism and a variable-level importance examination. Meanwhile, we equip T4 with an uncertainty quantification to help prevent overconfident recommendations. We demonstrate that T4 can identify effective treatment timing with estimated individual treatment effects for antibiotic stewardship on two real-world datasets. Moreover, comprehensive experiments on a synthetic dataset exhibit the outstanding performance of T4 compared with the state-of-the-art models on estimation of individual treatment effect.
更多查看译文
关键词
Data mining,Machine learning,Translational research,Engineering,general
AI 理解论文
溯源树
样例
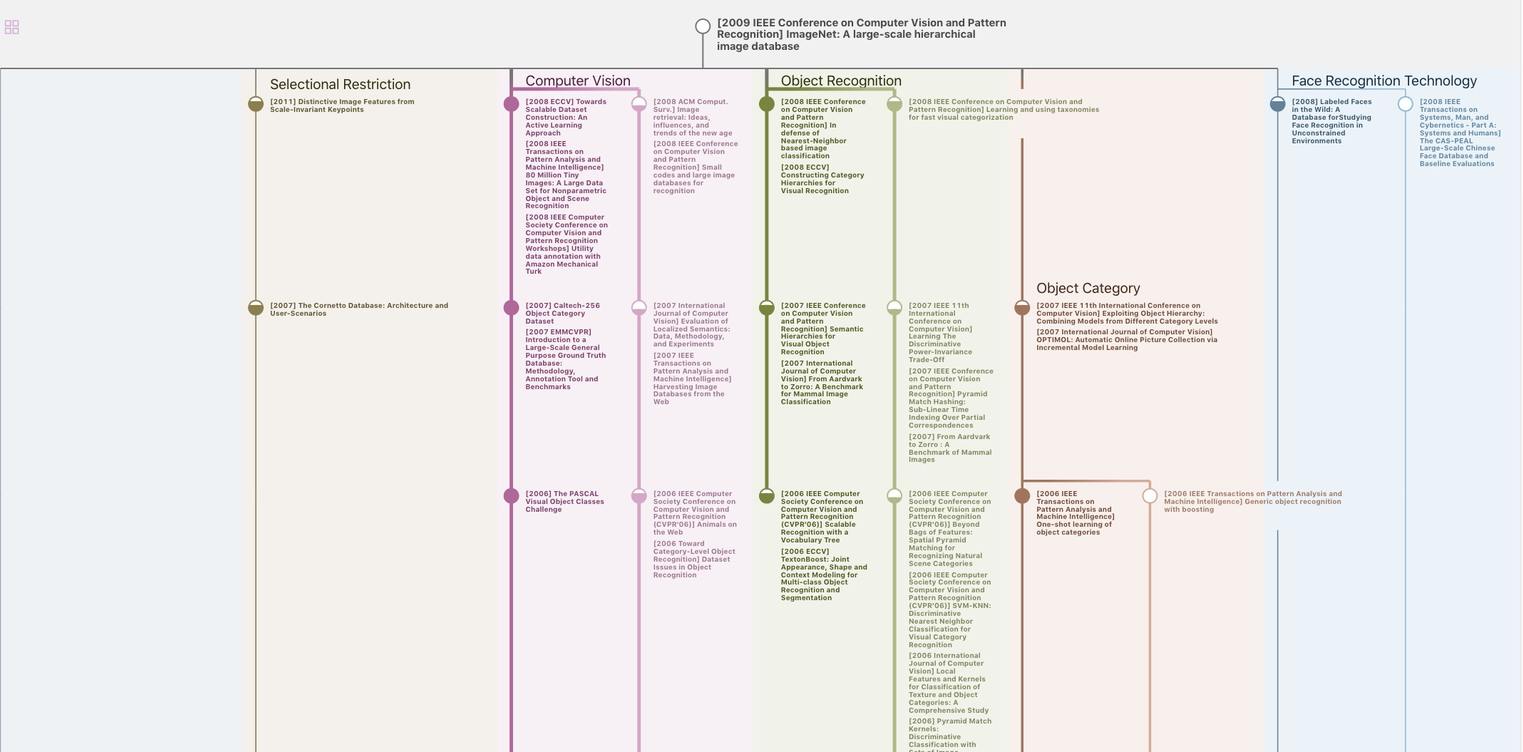
生成溯源树,研究论文发展脉络
Chat Paper
正在生成论文摘要