Analysis of functional connectivity using machine learning and deep learning in multimodal data from patients with schizophrenia
medRxiv (Cold Spring Harbor Laboratory)(2022)
摘要
Schizophrenia is a severe mental disorder associated with persistent or recurrent psychosis, hallucinations, delusions, and thought disorders that affect approximately 26 million people worldwide, according to the World Health Organization (WHO). Several studies encompass machine learning and deep learning algorithms to automate the diagnosis of this mental disorder. Others study schizophrenia brain networks to get new insights into the dynamics of information processing in patients suffering from the condition. In this paper, we offer a rigorous approach with machine learning and deep learning techniques for evaluating connectivity matrices and measures of complex networks to establish an automated diagnosis and comprehend the topology and dynamics of brain networks in schizophrenia patients. For this purpose, we employed an fMRI and EEG dataset in a multimodal fashion. In addition, we combined EEG measures, i.e., Hjorth mobility and complexity, to complex network measurements to be analyzed in our model for the first time in the literature. When comparing the schizophrenia group to the control group, we found a high positive correlation between the left superior parietal lobe and the left motor cortex and a positive correlation between the left dorsal posterior cingulate cortex and the left primary motor. In terms of complex network measures, the diameter, which corresponds to the longest shortest path length in a network, may be regarded as a biomarker because it is the most important measure in a multimodal fashion. Furthermore, the schizophrenia brain networks exhibit less segregation and lower distribution of information. As a final result, EEG measures outperformed complex networks in capturing the brain alterations associated with schizophrenia. As a result, our model achieved an AUC of 100%, an accuracy of 98% for the fMRI, an AUC of 95 %, and an accuracy of 95% for the EEG data set. These are excellent classification results. Furthermore, we investigated the impact of specific brain connections and network measures for these results, which helped us better describe changes in the diseased brain.
### Competing Interest Statement
The authors have declared no competing interest.
### Funding Statement
This study was funded by FAPESP and CNPQ funding.
### Author Declarations
I confirm all relevant ethical guidelines have been followed, and any necessary IRB and/or ethics committee approvals have been obtained.
Yes
The details of the IRB/oversight body that provided approval or exemption for the research described are given below:
The study used ONLY openly available human data .
I confirm that all necessary patient/participant consent has been obtained and the appropriate institutional forms have been archived, and that any patient/participant/sample identifiers included were not known to anyone (e.g., hospital staff, patients or participants themselves) outside the research group so cannot be used to identify individuals.
Yes
I understand that all clinical trials and any other prospective interventional studies must be registered with an ICMJE-approved registry, such as ClinicalTrials.gov. I confirm that any such study reported in the manuscript has been registered and the trial registration ID is provided (note: if posting a prospective study registered retrospectively, please provide a statement in the trial ID field explaining why the study was not registered in advance).
Yes
I have followed all appropriate research reporting guidelines and uploaded the relevant EQUATOR Network research reporting checklist(s) and other pertinent material as supplementary files, if applicable.
Yes
All data produced in the present work are contained in the manuscript
* ### NOMENCLATURE
APL
: Average shortest path length
AUC
: Area Under ROC Curve
BA
: Brodmann Areas
BASC
: Bootstrap Analysis of Stable Clusters
BC
: Betweenness centrality
BM
: Biweight Midcorrelation
BOLD
: Blood Oxygenation Level Dependent
CC
: Closeness centrality
COBRE
: The Centers of Biomedical Research Excellence
DMN
: Default Mode Network
EBC
: Edge betweenness community detection
EC
: Eigenvector centrality
ED
: Entropy of the degree distribution
EEG
: Electroencephalogram
FC
: Fastgreedy community detection
fMRI
: Functional Magnetic Resonance imaging
GC
: Granger Causality
GL
: Graphical Lasso method
IC
: Infomap community detection
Knn
: Average degree of nearest neighbors
LC
: Leading eigenvector community detection
LPC
: Label propagation community detection
LSP
: Left Superior Parietal Lobe
LSTM
: Long short-term memory
LW
: Ledoit-Wolf shrinkage
MC
: Multilevel community detection
MI
: Mutual Information
MLP
: Multilayer Perceptron
NB
: Naive Bayes
PC
: Pearson Correlation
PCC
: Posterior Cingulate Cortex
PMC
: Primary Motor Cortex
RF
: Random Forest
ROC
: Receiver Operating Characteristic
ROIs
: Regions of interest
SC
: Spearman Correlation
SCC
: Canonical Correlation analysis
SCZ
: Schizophrenia
SHAP
: SHapley Additive Explanations
SMA
: Supplementary Motor Area
SMD
: Second moment of the degree distribution
SPC
: Spinglass community detection
TE
: Transfer Entropy
Tuned
: CNN tuned Convolution Neural Network
untuned
: CNN Untuned Convolution Neural Network
WHO
: World Health Organization
更多查看译文
关键词
functional connectivity,schizophrenia,multimodal data,deep learning
AI 理解论文
溯源树
样例
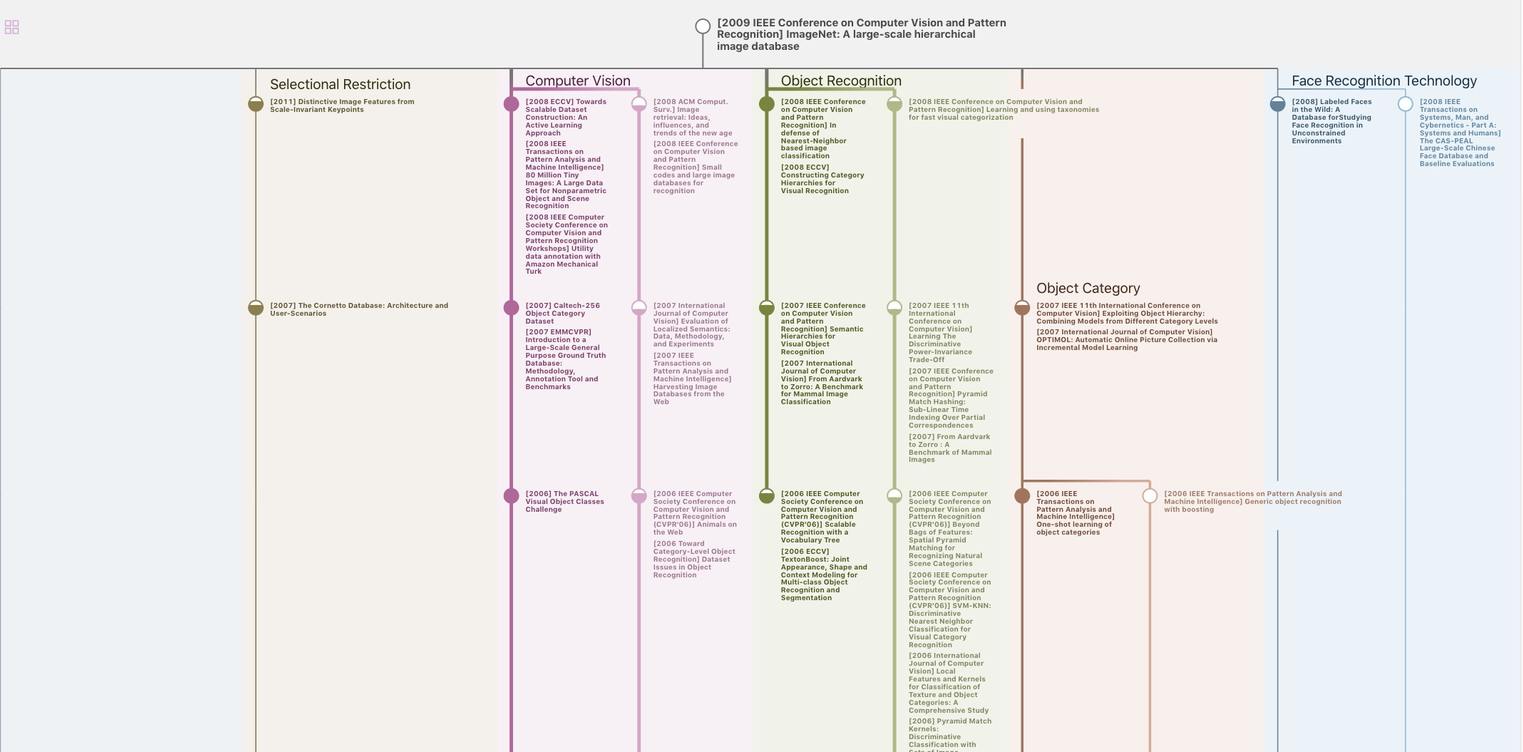
生成溯源树,研究论文发展脉络
Chat Paper
正在生成论文摘要