An ensemble deep learning model with empirical wavelet transform feature for oral cancer histopathological image classification
International Journal of Data Science and Analytics(2024)
摘要
Oral squamous cell carcinoma (OSCC) has become quite prevalent across many countries, and poor prognosis is one of the major reasons for the ensuing high mortality rate. Diagnosis of OSCC is generally done by pathologists who mostly rely on their years of empirical experience from tissue biopsy sections. The possibilities of human errors increase while detecting the cells using microscopy biopsy images physically. With the growth of artificial intelligence, deep learning models have gained immense importance in recent years and have become one of the core technologies in medical diagnosis. It not only enhances accuracy, but also fastens the image classification process, as a result, lowering human errors and workload. Here, we have made use of a customized deep learning model for aiding pathologists in better OSCC detection from histopathological images. We accumulated and analyzed a complete set of 696 histopathological oral images; among them, 80% have been taken in the training set, 10% of the images are included in the validation set, and the rest 10% for testing purposes. In this study, 2D empirical wavelet transform is used to extract features from the images; later an ensemble of two pretrained models, namely ResNet50 and DenseNet201, is used for the classification of images into normal and OSCC classes. The efficacy of the model is assessed and compared in terms of accuracy, sensitivity and specificity. The simulation results show that the proposed model has achieved an highest classification accuracy of 0.92. Thus, this method may be utilized for assisting in the binary classification of oral histopathological images.
更多查看译文
关键词
OSCC,Transfer learning,Ensemble learning,ResNet50,DenseNet201,EWT
AI 理解论文
溯源树
样例
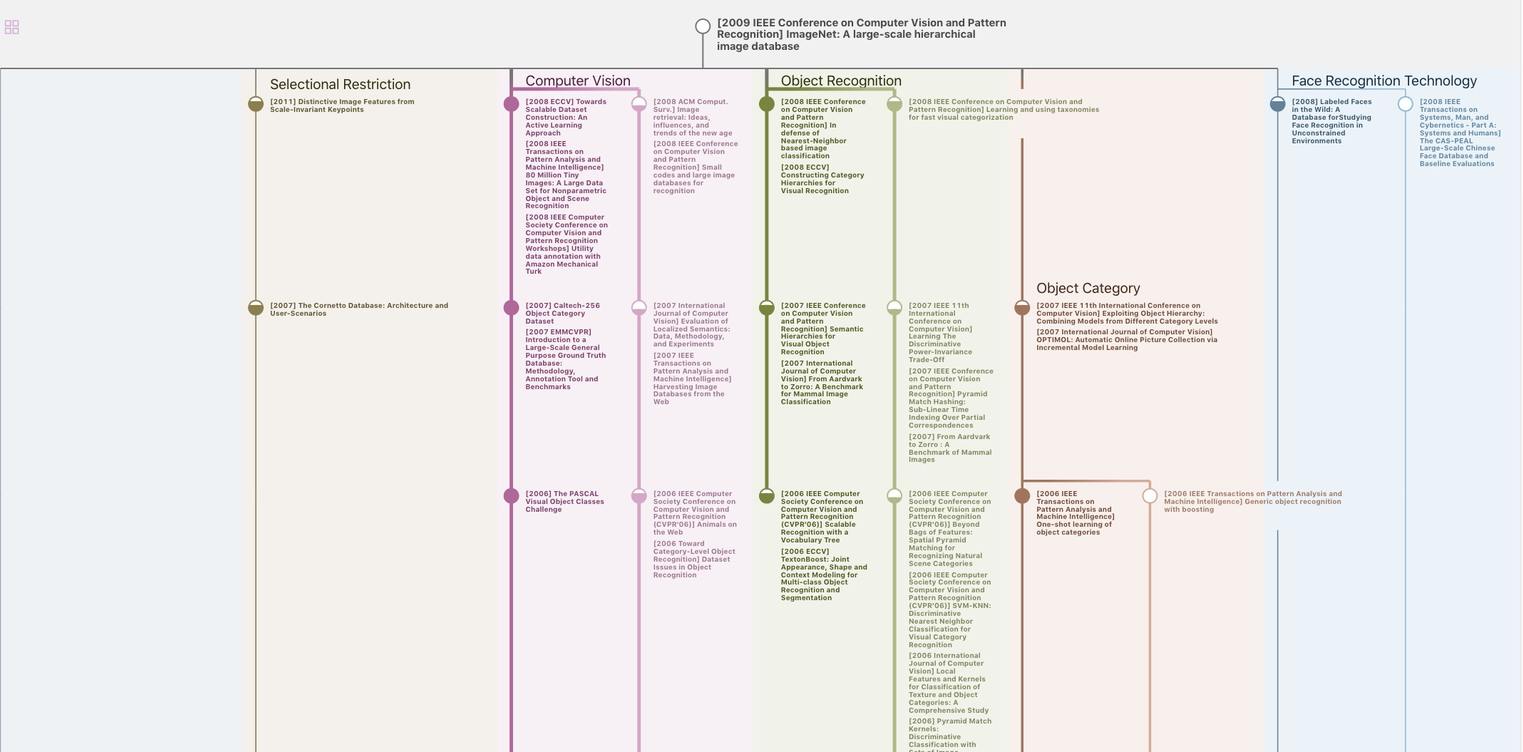
生成溯源树,研究论文发展脉络
Chat Paper
正在生成论文摘要