Global spatiotemporal trends and determinants of COVID-19 vaccine acceptance on Twitter: a multilingual deep learning study in 135 countries and territories
medrxiv(2022)
摘要
Background COVID-19 vaccination has faced a range of challenges from supply-side barriers such as insufficient vaccine supply and negative information environment and demand-side barriers centring on public acceptance and confidence in vaccines. This study assessed global spatiotemporal trends in demand- and supply-side barriers to vaccine uptake using COVID-19-related social media data and explored the country-level determinants of vaccine acceptance.
Methods We accessed a total of 13,093,406 tweets sent between November 2020 and March 2022 about the COVID-19 vaccine in 90 languages from 135 countries using Meltwater™® (a social listening platform). Based on 8,125 manually-annotated tweets, we fine-tuned multilingual deep learning models to automatically annotate all 13,093,406 tweets. We present spatial and temporal trends in four key spheres: (1) COVID-19 vaccine acceptance; (2) confidence in COVID-19 vaccines; (3) the online information environment regarding the COVID-19 vaccine; and (4) perceived supply-side barriers to COVID-19 vaccination. Using univariate and multilevel regressions, we evaluated the association between COVID-19 vaccine acceptance on Twitter® and (1) country-level characteristics regarding governance, pandemic preparedness, trust, culture, social development, and population demographics; (2) country-level COVID-19 vaccine coverage; and (3) Google® search trends on adverse vaccine events.
Findings COVID-19 vaccine acceptance was high among Twitter® users in Southeast Asian, Eastern Mediterranean, and Western Pacific countries, including India, Indonesia, and Pakistan. In contrast, acceptance was relatively low in high-income nations like South Korea, Japan, and the Netherlands. Spatial variations were correlated with country-level governance, pandemic preparedness, public trust, culture, social development, and demographic determinants. At the country level, vaccine acceptance sentiments expressed on Twitter® predicted higher vaccine coverage. We noted the declining trend of COVID-19 vaccine acceptance among global Twitter® users since March 2021, which was associated with increased searches for adverse vaccine events. Interpretation In future pandemics, new vaccines may face the potential low-level and declining trend in acceptance, like COVID-19 vaccines, and early responses are needed. Social media mining represents a promising surveillance approach to monitor vaccine acceptance and can be validated against real-world vaccine uptake data.
Funding National Natural Science Foundation of China.
### Competing Interest Statement
The Vaccine Confidence Project, which HJL leads, receives collaborative grants with Astra Zeneca, GlaxoSmithKline, J&J, and Merck in addition to public sector grants. In the past 24 months, AdF has worked on Vaccine Confidence Project grants funded by MSD and consulted Pfizer on programs relating to strengthening childhood immunization programs. SHV has been funded by Sinovac for field effectiveness studies of CoronaVac inactivated SARS-CoV-2 vaccine in the Dominican Republic. None of those research grants are related to this paper.
### Funding Statement
Zhiyuan Hou acknowledges financial support from the National Natural Science Foundation of China (71874034), and the Soft Science Research Project of Shanghai "Science and Technology Innovation Action Plan" (22692107600).
### Author Declarations
I confirm all relevant ethical guidelines have been followed, and any necessary IRB and/or ethics committee approvals have been obtained.
Yes
The details of the IRB/oversight body that provided approval or exemption for the research described are given below:
The study was approved by the Institutional Review Board of the School of Public Health, Fudan University (IRB#2022-01-0938).
I confirm that all necessary patient/participant consent has been obtained and the appropriate institutional forms have been archived, and that any patient/participant/sample identifiers included were not known to anyone (e.g., hospital staff, patients or participants themselves) outside the research group so cannot be used to identify individuals.
Yes
I understand that all clinical trials and any other prospective interventional studies must be registered with an ICMJE-approved registry, such as ClinicalTrials.gov. I confirm that any such study reported in the manuscript has been registered and the trial registration ID is provided (note: if posting a prospective study registered retrospectively, please provide a statement in the trial ID field explaining why the study was not registered in advance).
Yes
I have followed all appropriate research reporting guidelines and uploaded the relevant EQUATOR Network research reporting checklist(s) and other pertinent material as supplementary files, if applicable.
Yes
All data described in the results and Python/R codes for data analysis/visualization are shared on GitHub, upon acceptance of this paper. Original tweets are not shared according to Twitter's data policy. Other data and codes are available on request to the corresponding author.
更多查看译文
关键词
vaccine,multilingual deep learning study,twitter,global spatiotemporal trends
AI 理解论文
溯源树
样例
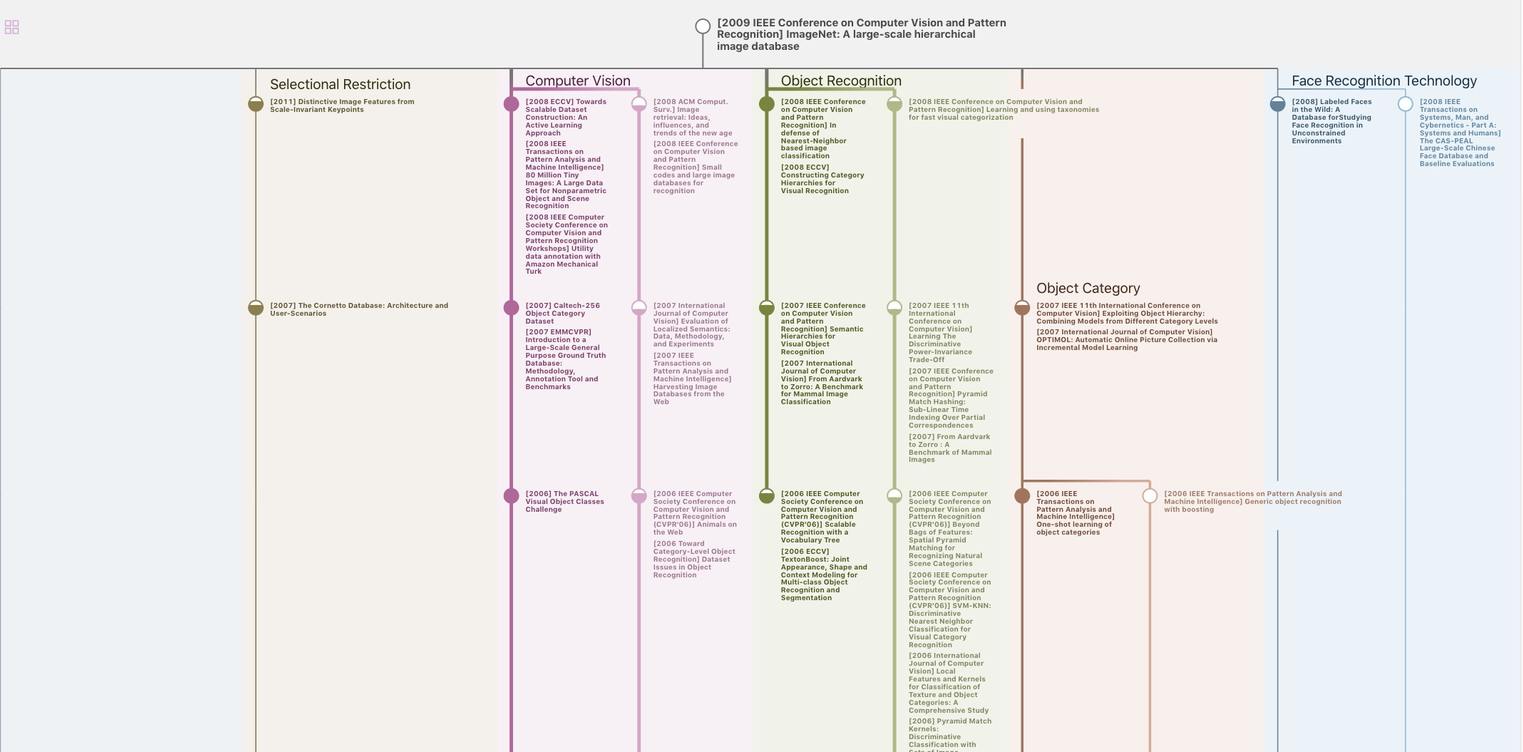
生成溯源树,研究论文发展脉络
Chat Paper
正在生成论文摘要